Compatibility Review for Object Detection Enhancement through Super-Resolution.
Sensors (Basel, Switzerland)(2024)
摘要
With the introduction of deep learning, a significant amount of research has been conducted in the field of computer vision in the past decade. In particular, research on object detection (OD) continues to progress rapidly. However, despite these advances, some limitations need to be overcome to enable real-world applications of deep learning-based OD models. One such limitation is inaccurate OD when image quality is poor or a target object is small. The performance degradation phenomenon for small objects is similar to the fundamental limitations of an OD model, such as the constraint of the receptive field, which is a difficult problem to solve using only an OD model. Therefore, OD performance can be hindered by low image quality or small target objects. To address this issue, this study investigates the compatibility of super-resolution (SR) and OD techniques to improve detection, particularly for small objects. We analyze the combination of SR and OD models, classifying them based on architectural characteristics. The experimental results show a substantial improvement when integrating OD detectors with SR models. Overall, it was demonstrated that, when the evaluation metrics (PSNR, SSIM) of the SR models are high, the performance in OD is correspondingly high as well. Especially, evaluations on the MS COCO dataset reveal that the enhancement rate for small objects is 9.4% higher compared to all objects. This work provides an analysis of SR and OD model compatibility, demonstrating the potential benefits of their synergistic combination. The experimental code can be found on our GitHub repository.
更多查看译文
关键词
deep learning,neural networks,super-resolution,object detection,face recognition
AI 理解论文
溯源树
样例
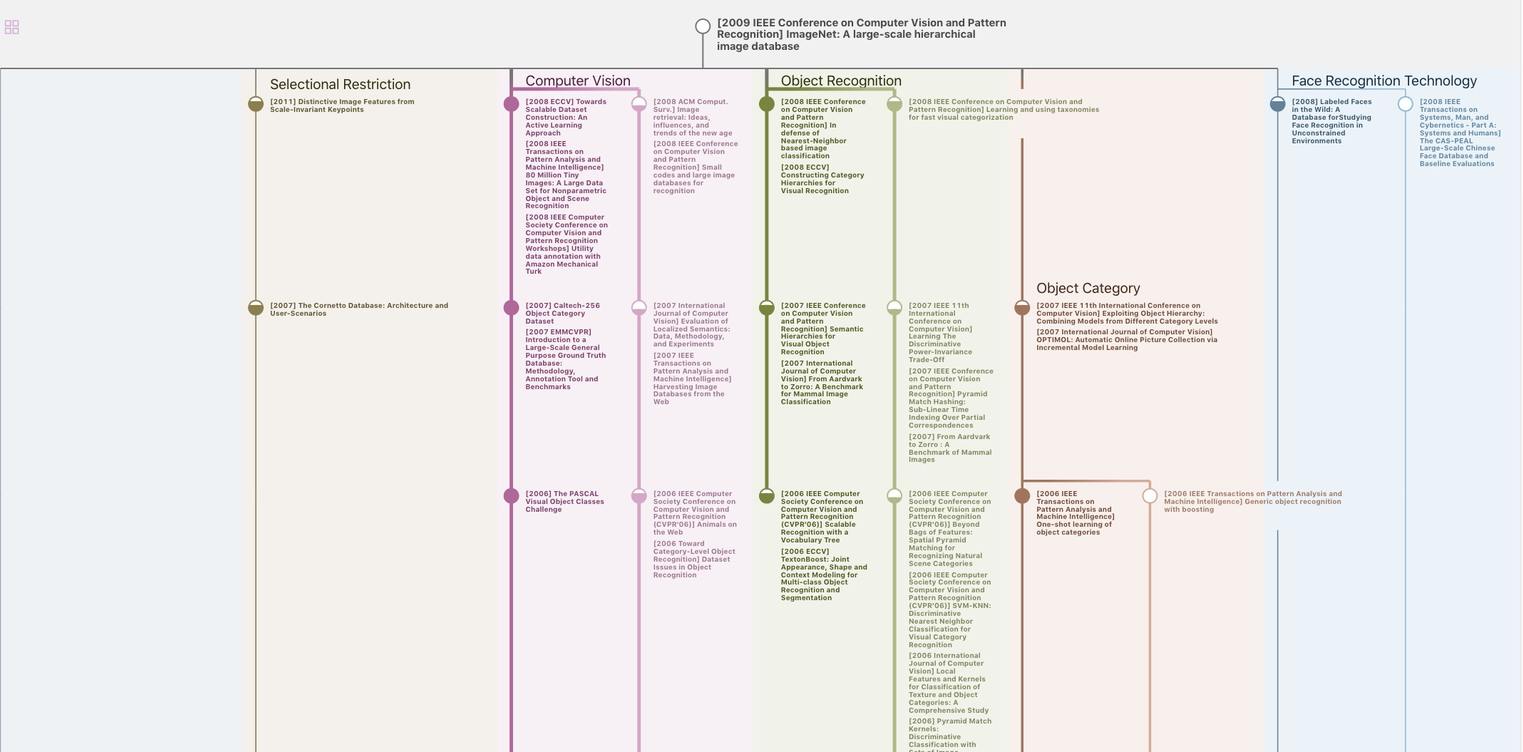
生成溯源树,研究论文发展脉络
Chat Paper
正在生成论文摘要