Novel drug discovery: Advancing Alzheimer's therapy through machine learning and network pharmacology
EUROPEAN JOURNAL OF PHARMACOLOGY(2024)
摘要
Alzheimer's disease (AD), marked by tau tangles and amyloid-beta plaques, leads to cognitive decline. Despite extensive research, its complex etiology remains elusive, necessitating new treatments. This study utilized machine learning (ML) to analyze compounds with neuroprotective potential. This approach exposed the disease's complexity and identified important proteins, namely MTOR and BCL2, as central to the pathogenic network of AD. MTOR regulates neuronal autophagy and survival, whereas BCL2 regulates apoptosis, both of which are disrupted in AD. The identified compounds, including Armepavine, Oprea1_264702,1-cyclopropyl-7-fluoro-8methoxy-4-oxoquinoline-3-carboxylic acid,(2S)-4 '-Hydroxy-5,7,3 '-trimethoxyflavan,Oprea1_130514,Sativanone, 5-hydroxy-7,8-dimethoxyflavanone,7,4 '-Dihydroxy-8,3 '-dimethoxyflavanone,N,1-dicyclopropyl-6,Difluoro-Meth oxy-Gatifloxacin,6,8-difluoro-1-(2-fluoroethyl),1-ethyl-6-fluoro-7-(4-methylpiperidin-1-yl),Avicenol C, demonstrated potential modulatory effects on these proteins. The potential for synergistic effects of these drugs in treating AD has been revealed via network pharmacology. By targeting numerous proteins at once, these chemicals may provide a more comprehensive therapeutic approach, addressing many aspects of AD's complex pathophysiology. A Molecular docking, dynamic simulation, and Principle Component Analysis have confirmed these drugs' efficacy by establishing substantial binding affinities and interactions with important proteins such as MTOR and BCL2. This evidence implies that various compounds may interact within the AD pathological framework, providing a sophisticated and multifaceted therapy strategy. In conclusion, our study establishes a solid foundation for the use of these drugs in AD therapy. Thus current study highlights the possibility of multitargeted, synergistic therapeutic approaches in addressing the complex pathophysiology of AD by integrating machine learning, network pharmacology, and molecular docking simulations. This holistic technique not only advances drug development but also opens up new avenues for developing more effective treatments for this difficult and widespread disease.
更多查看译文
关键词
Alzheimer's disease (AD),Network pharmacology,Machine learning,Molecular docking simulation,Compounds,Multi-target drug discovery
AI 理解论文
溯源树
样例
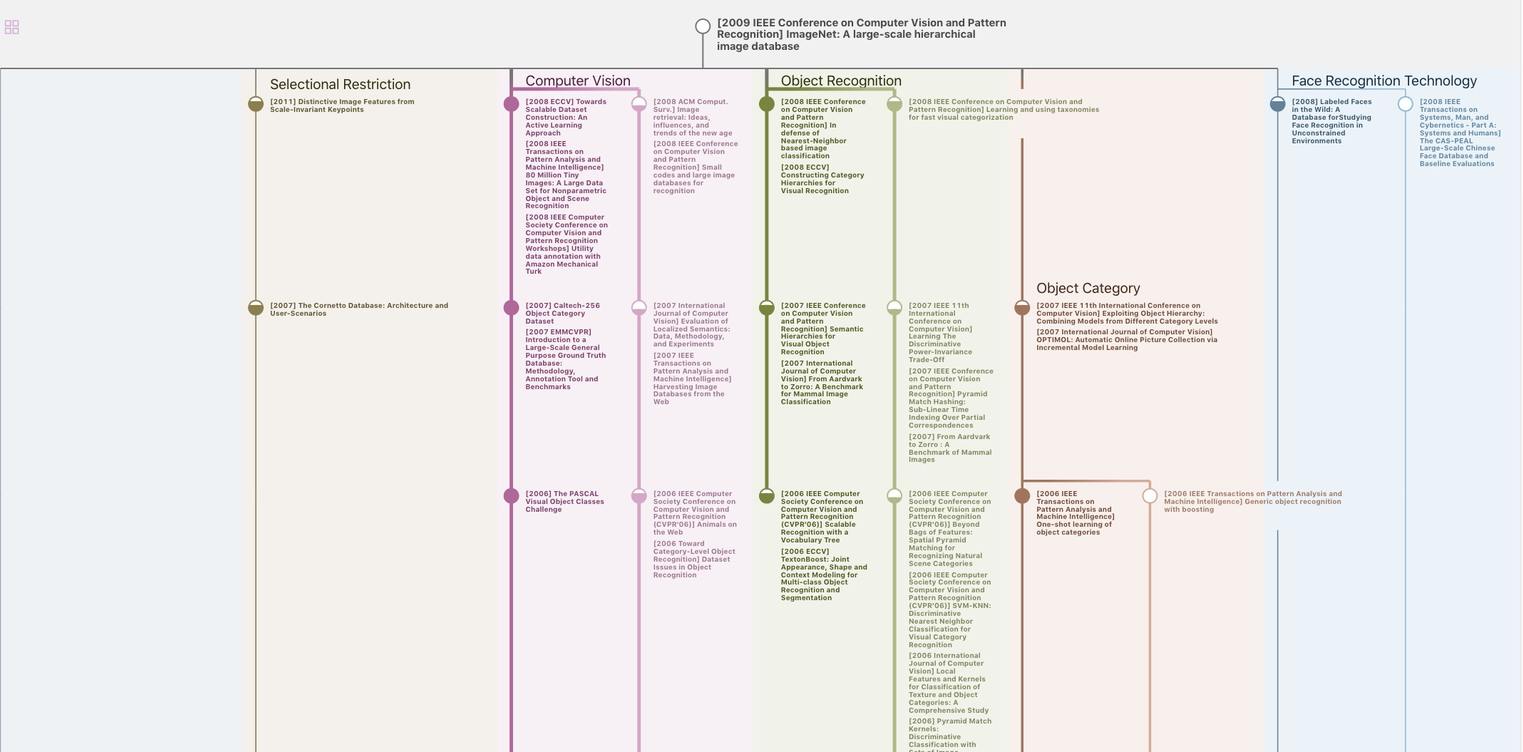
生成溯源树,研究论文发展脉络
Chat Paper
正在生成论文摘要