Domain Adaptive Thermal Object Detection with Unbiased Granularity Alignment
ACM Transactions on Multimedia Computing, Communications, and Applications(2023)
摘要
Domain Adaptive Object Detection (DAOD) alleviates the challenge of labor-intensive annotations by transferring semantic information from a labeled source domain to an unlabeled target domain. However, the DAOD suffers from biased discrimination and negative transfer in the thermal domain due to the inherent heterogeneity between the RGB and thermal images. To address the above issues, we propose the Unbiased Granularity Alignment (UGA) framework to facilitate the unified alignment for RGB-Thermal DAOD. Specifically, we devise a Channel Self-encoding Adaptation (CSA) module to mitigate biased discrimination from the discriminative enhancement perspective. CSA aligns the intra-domain channel subspace for inter-domain channel harmonizing. Upon revisiting instance alignment, we uncovered inaccuracies proposals and unstable positive sample phenomena. Therefore, we propose the Relative Relationship Adaptation (RRA) module to mitigate negative transfer. RRA ensures inter-domain semantic consistency through sparse instance alignment. Extensive experiments are conducted on visible-to-thermal and visible-to-visible benchmarks to validate the effectiveness, and our UGA framework outperforms state-of-the-art by a remarkable margin. The code of our UGA is available at https://github.com/zyfone/UGA .
更多查看译文
关键词
Domain adaptive thermal object detection,Unbiased granularity alignment,Intra-domain subspace alignment,Sparse instance alignment
AI 理解论文
溯源树
样例
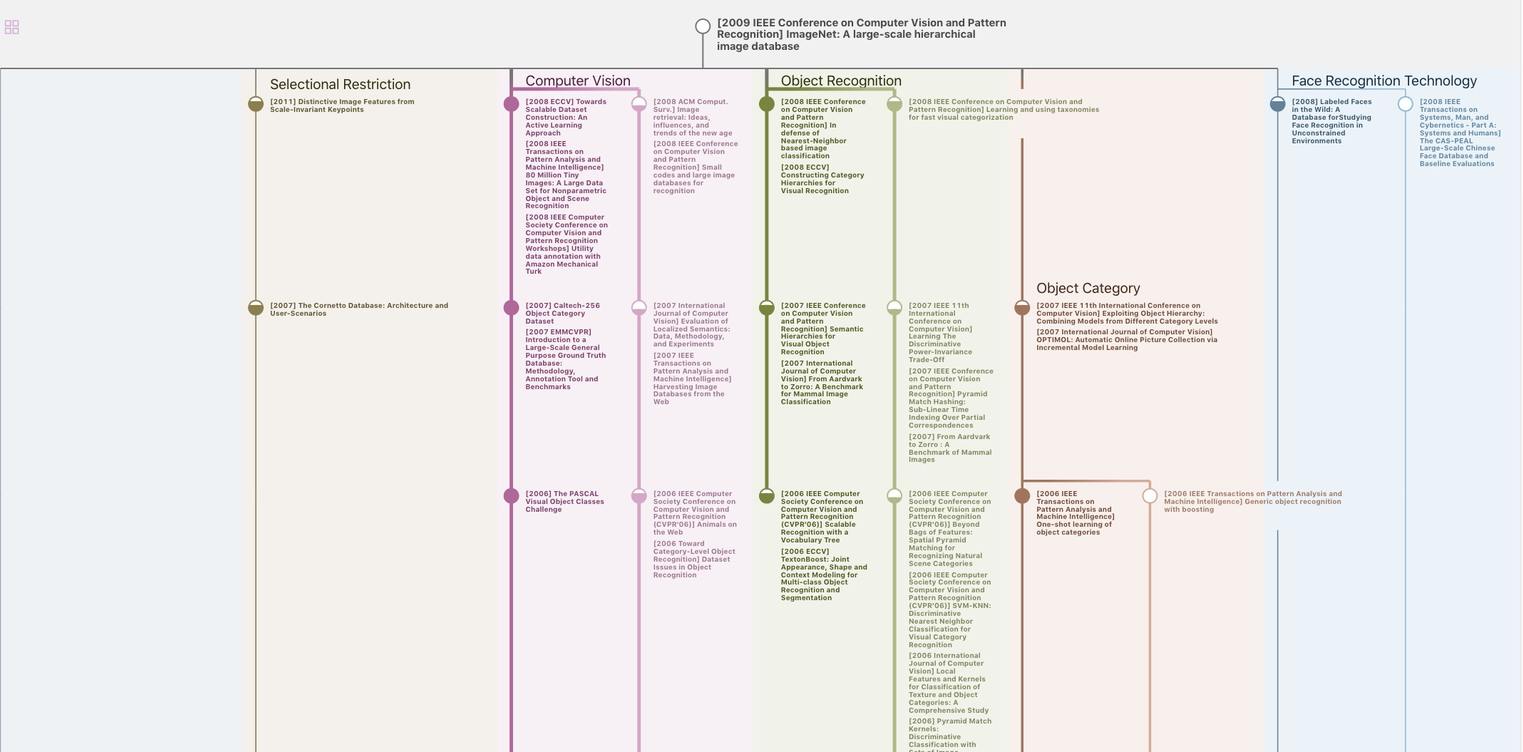
生成溯源树,研究论文发展脉络
Chat Paper
正在生成论文摘要