#1085 Prediction models for hospitalization in patients with advanced chronic kidney disease: systematic review, external validation and development study
Nephrology Dialysis Transplantation(2024)
摘要
Abstract Background and Aims Hospitalization is a common complication in patients with advanced chronic kidney disease (CKD). Predicting hospitalization would be beneficial for clinicians and patients. Clinicians may be assisted in adequate healthcare planning by being able to anticipate hospitalizations. Additionally, patients have indicated wanting more information about their risk of hospitalization. By providing patients a personal indication of their risk of hospitalization, they better know what to expect of the future which can foster a feeling of control. Especially for incident dialysis patients this might help, as they enter a new and to them still largely unknown stage of their disease. Moreover, if a patient knows they have a high risk of hospitalization, they may better cope with the hospitalization if it occurs, as it is less unexpected. Therefore, we aimed to 1) identify and give an overview of current prediction models for hospitalization in CKD patients, 2) externally validate these models, and 3) develop a new model if no well-performing models were identified. Method We performed a systematic review in PubMed to identify prediction models for hospitalization, length of stay (LOS), and readmission. Identified models were appraised using the Prediction model Risk of Bias Assessment Tool. We targeted three external validations in hemodialysis (HD), peritoneal dialysis (PD), and pre-dialysis patients in a Dutch dialysis and European pre-dialysis cohort. Prediction models were externally validated on discrimination (C-statistic) and calibration (calibration plot, slope, and calibration-in-the-large). We developed a Fine-Gray model for hospitalization within one year in patients initiating HD, taking into account the competing risk of death. Informed by model performance, we also investigated Cox and logistic regression as modeling strategies. We performed a sensitivity analysis, redefining the outcome to be predicted as the 1-year risk of hospitalization of 3 days or longer. Results From the systematic search, we identified 17 models in 6 studies. All models were developed in the USA or in China. Two models predicted hospitalization rate in incident PD patients and 3 models predicted 30-day readmission in maintenance HD patients and in CKD stage 3-5 patients not on dialysis discharged after a heart failure hospitalization. The risk of 1 and 6 hospitalizations within one year in prevalent HD patients were predicted by one model each. Prolonged LOS in PD patients was predicted by ten models. All models were of low quality with a high risk of bias. The most common shortcomings were using future information to make predictions, inadequate handling of missing data, and model overfitting. Due to underreporting and unavailability of predictors, we could only externally validate 3 models. These models were poorly calibrated and had poor discrimination. We developed a new model in 1,020 incident HD patients predicting 1-year risk of hospitalization. We used age, cardiovascular disease, diabetes mellitus, malignancy, estimated glomerular filtration rate, and body mass index as predictors, selected based on literature and clinical expertise. The model discriminated poorly (C-statistic, 95% confidence interval [CI]; 0.55, 0.53-0.58), also in targeted external validations in 620 PD patients (0.55, 0.52-0.58) and 1,718 pre-dialysis patients (0.52, 0.52-0.54). Calibration was poor for all models. Using different modelling strategies, model performance did not improve. In the sensitivity analysis, discrimination improved but was still low (HD population: 0.58, 0.55-0.62; PD population: 0.59, 0.54-0.63; pre-dialysis: 0.55, 0.52-0.57). Conclusion Hospitalizations are important but difficult to predict for patients with advanced CKD. Current models are insufficient to predict hospitalizations. We offer two main suggestions to improve future predictions of hospitalizations. First, the outcome of hospitalization should be more specific (e.g., cardiovascular hospitalizations) to reduce outcome heterogeneity. Second, the selection of predictors should be extended with more patient-centered factors, such as patient-reported outcomes.
更多查看译文
AI 理解论文
溯源树
样例
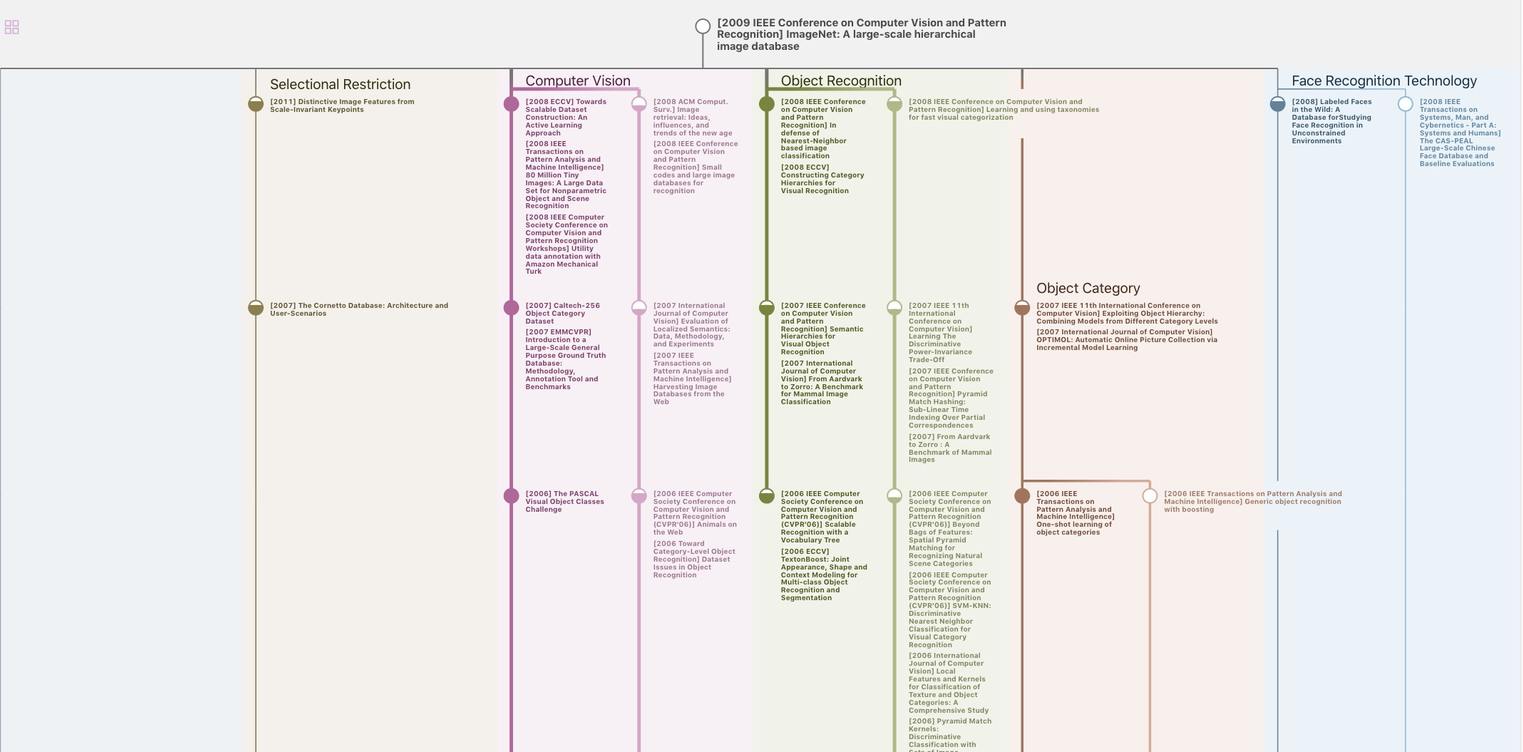
生成溯源树,研究论文发展脉络
Chat Paper
正在生成论文摘要