Citrus Pest Identification Model Based on Improved ShuffleNet
Applied Sciences(2024)
摘要
To address the current issues of complex structures and low accuracies in citrus pest identification models, a lightweight pest identification model was proposed. First, a parameterized linear rectification function was introduced to avoid neuronal death. Second, the model’s attention to pest characteristics was improved by incorporating an improved mixed attention mechanism. Subsequently, the network structure of the original model was adjusted to reduce architectural complexity. Finally, by employing transfer learning, an SCHNet model was developed. The experimental results indicated that the proposed model achieved an accuracy rate of 94.48% with a compact size of 3.84 MB. Compared to the original ShuffleNet V2 network, the SCHNet model showed a 3.12% accuracy improvement while reducing the model size by 22.7%. The SCHNet model exhibited an excellent classification performance for citrus pest identification, enabling the accurate identification of citrus pests.
更多查看译文
关键词
citrus,lightweight,parametric linear rectification,attention mechanism,transfer learning
AI 理解论文
溯源树
样例
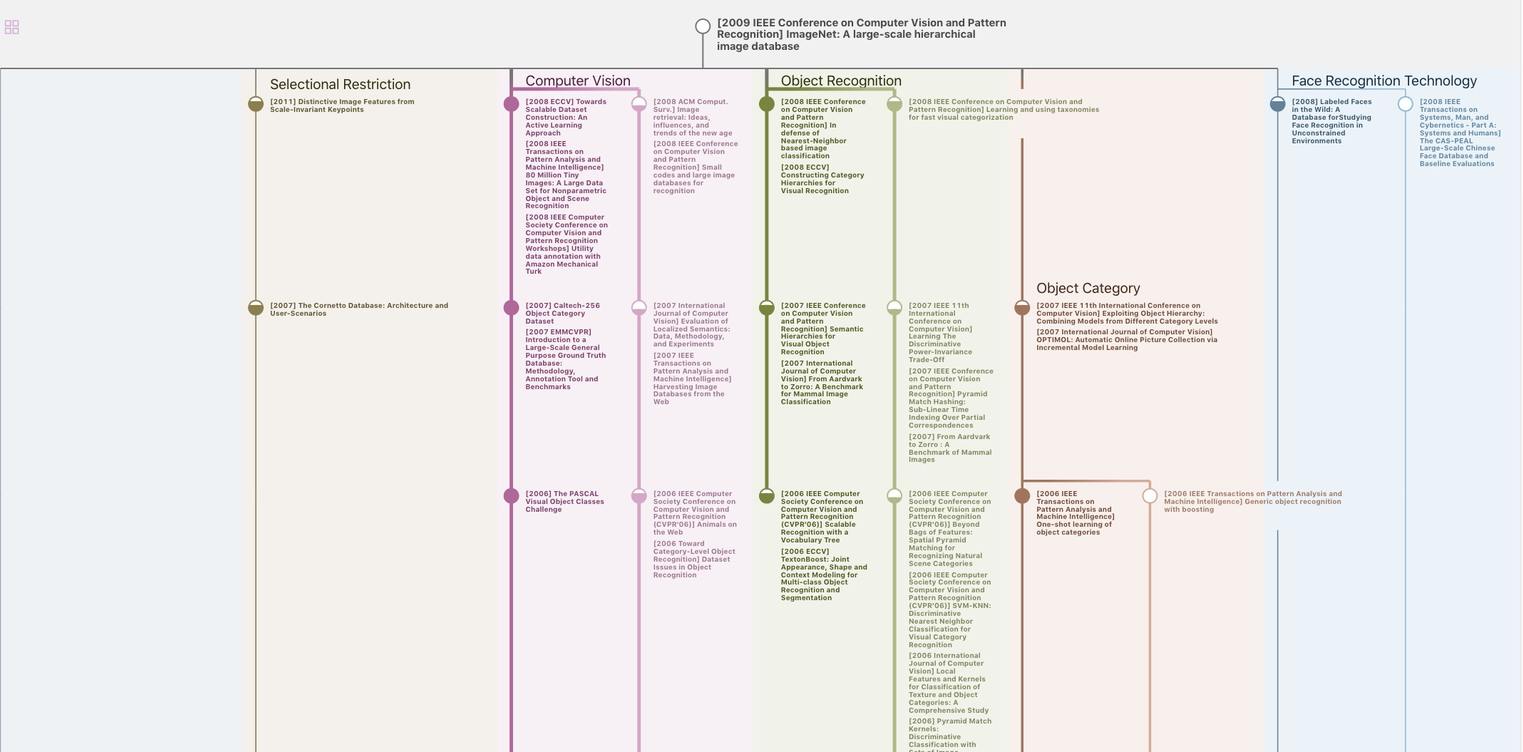
生成溯源树,研究论文发展脉络
Chat Paper
正在生成论文摘要