Aligning Embeddings and Geometric Random Graphs: Informational Results and Computational Approaches for the Procrustes-Wasserstein Problem
CoRR(2024)
Abstract
The Procrustes-Wasserstein problem consists in matching two high-dimensional
point clouds in an unsupervised setting, and has many applications in natural
language processing and computer vision. We consider a planted model with two
datasets X,Y that consist of n datapoints in ℝ^d, where Y is a
noisy version of X, up to an orthogonal transformation and a relabeling of
the data points. This setting is related to the graph alignment problem in
geometric models. In this work, we focus on the euclidean transport cost
between the point clouds as a measure of performance for the alignment. We
first establish information-theoretic results, in the high (d ≫log n) and
low (d ≪log n) dimensional regimes. We then study computational aspects
and propose the Ping-Pong algorithm, alternatively estimating the orthogonal
transformation and the relabeling, initialized via a Franke-Wolfe convex
relaxation. We give sufficient conditions for the method to retrieve the
planted signal after one single step. We provide experimental results to
compare the proposed approach with the state-of-the-art method of Grave et al.
(2019).
MoreTranslated text
AI Read Science
Must-Reading Tree
Example
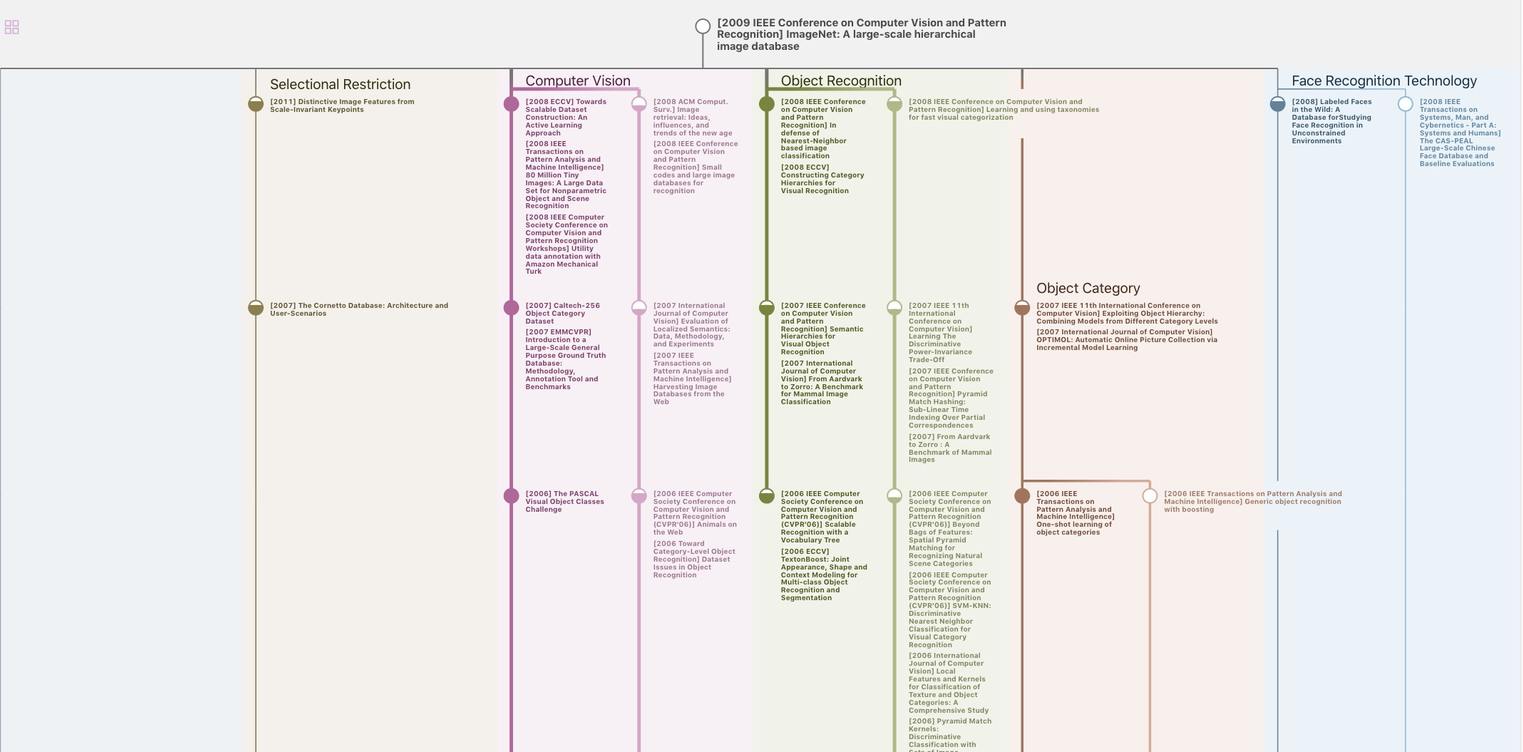
Generate MRT to find the research sequence of this paper
Chat Paper
Summary is being generated by the instructions you defined