Predicting the pathways of string-like motions in metallic glasses via path-featurizing graph neural networks.
Science advances(2024)
摘要
String-like motions (SLMs)-cooperative, "snake"-like movements of particles-are crucial for dynamics in diverse glass formers. Despite their ubiquity, questions persist: Do SLMs prefer specific paths? If so, can we predict these paths? Here, in Al-Sm glasses, our isoconfigurational ensemble simulations reveal that SLMs do follow certain paths. By designing a graph neural network (GNN) to featurize the environment around directional paths, we achieve a high-fidelity prediction of likely SLM pathways, solely based on the static structure. GNN gauges a structural measure to assess each path's propensity to engage in SLMs, akin to a "softness" metric, but for paths rather than for atoms. Our GNN interpretation reveals the critical role of the bottleneck zone along a path in steering SLMs. By monitoring "path softness," we elucidate that SLM-favored paths transit from fragmented to interconnected upon glass transition. Our findings reveal that, beyond atoms or clusters, glasses have another dimension of structural heterogeneity: "paths."
更多查看译文
AI 理解论文
溯源树
样例
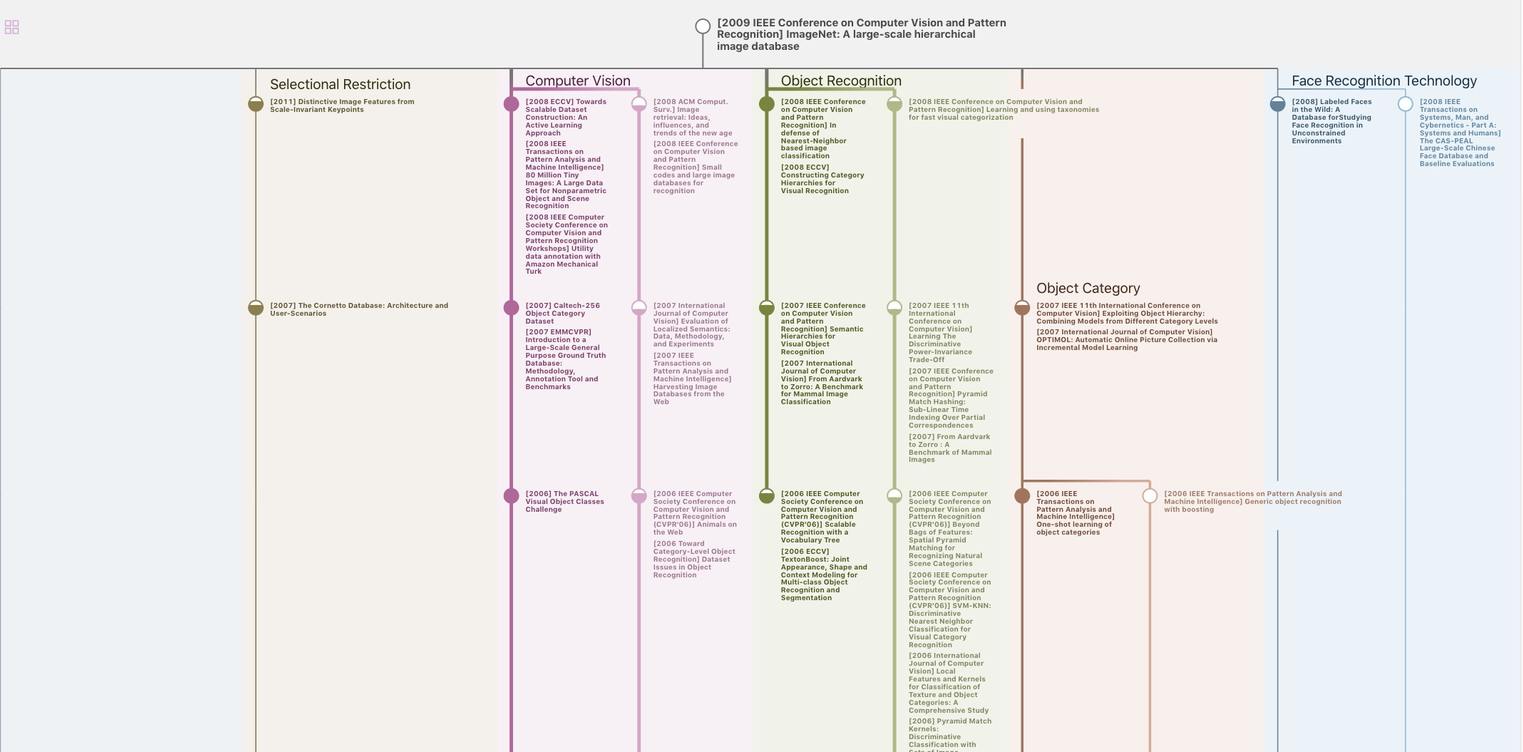
生成溯源树,研究论文发展脉络
Chat Paper
正在生成论文摘要