Graph Sparsification via Mixture of Graphs
CoRR(2024)
Abstract
Graph Neural Networks (GNNs) have demonstrated superior performance across
various graph learning tasks but face significant computational challenges when
applied to large-scale graphs. One effective approach to mitigate these
challenges is graph sparsification, which involves removing non-essential edges
to reduce computational overhead. However, previous graph sparsification
methods often rely on a single global sparsity setting and uniform pruning
criteria, failing to provide customized sparsification schemes for each node's
complex local context. In this paper, we introduce Mixture-of-Graphs (MoG),
leveraging the concept of Mixture-of-Experts (MoE), to dynamically select
tailored pruning solutions for each node. Specifically, MoG incorporates
multiple sparsifier experts, each characterized by unique sparsity levels and
pruning criteria, and selects the appropriate experts for each node.
Subsequently, MoG performs a mixture of the sparse graphs produced by different
experts on the Grassmann manifold to derive an optimal sparse graph. One
notable property of MoG is its entirely local nature, as it depends on the
specific circumstances of each individual node. Extensive experiments on four
large-scale OGB datasets and two superpixel datasets, equipped with five GNN
backbones, demonstrate that MoG (I) identifies subgraphs at higher sparsity
levels (8.67%∼ 50.85%), with performance equal to or better than the
dense graph, (II) achieves 1.47-2.62× speedup in GNN inference with
negligible performance drop, and (III) boosts “top-student” GNN performance
(1.02%↑ on RevGNN+ogbn-proteins and 1.74%↑ on
DeeperGCN+ogbg-ppa).
MoreTranslated text
AI Read Science
Must-Reading Tree
Example
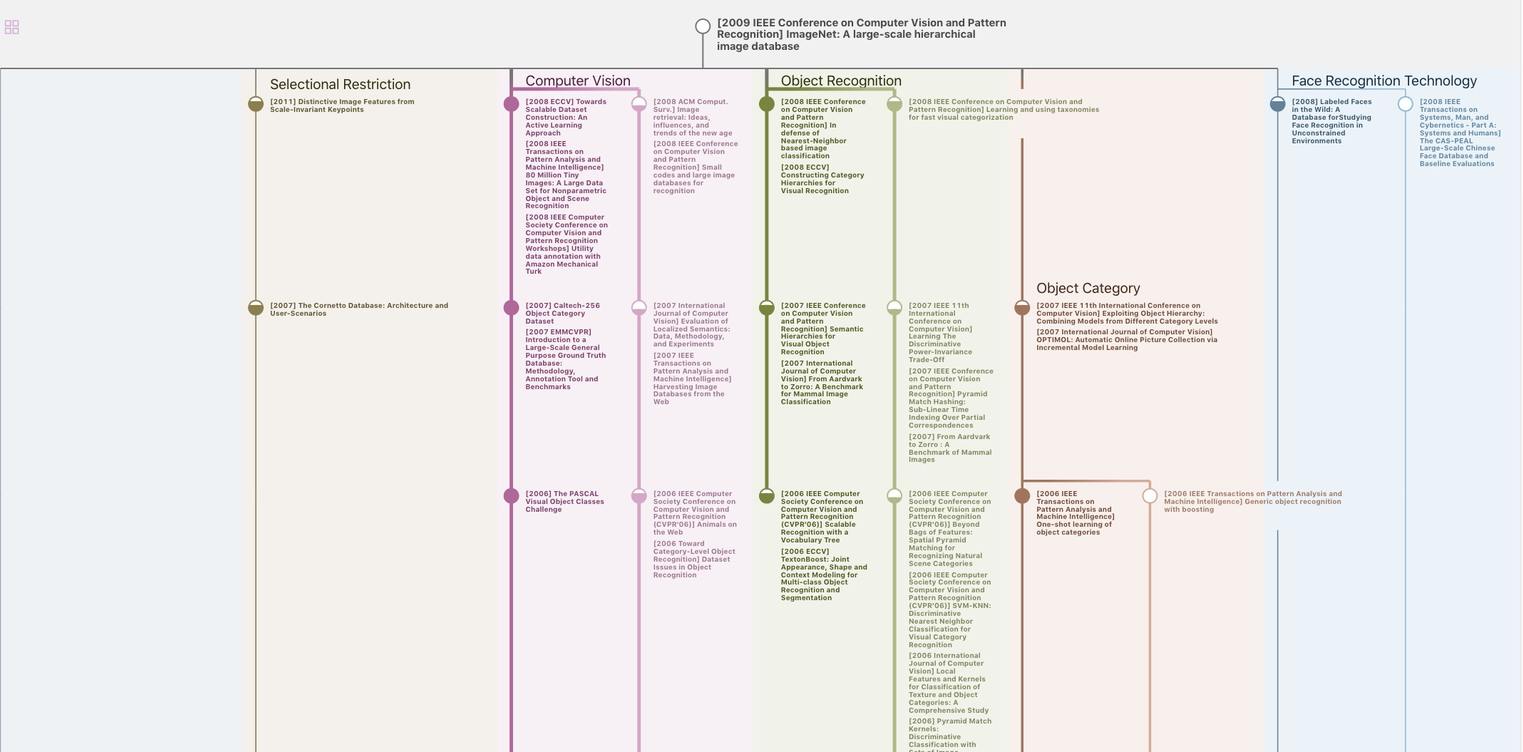
Generate MRT to find the research sequence of this paper
Chat Paper
Summary is being generated by the instructions you defined