Beyond Trend and Periodicity: Guiding Time Series Forecasting with Textual Cues
CoRR(2024)
Abstract
This work introduces a novel Text-Guided Time Series Forecasting (TGTSF)
task. By integrating textual cues, such as channel descriptions and dynamic
news, TGTSF addresses the critical limitations of traditional methods that rely
purely on historical data. To support this task, we propose TGForecaster, a
robust baseline model that fuses textual cues and time series data using
cross-attention mechanisms. We then present four meticulously curated benchmark
datasets to validate the proposed framework, ranging from simple periodic data
to complex, event-driven fluctuations. Our comprehensive evaluations
demonstrate that TGForecaster consistently achieves state-of-the-art
performance, highlighting the transformative potential of incorporating textual
information into time series forecasting. This work not only pioneers a novel
forecasting task but also establishes a new benchmark for future research,
driving advancements in multimodal data integration for time series models.
MoreTranslated text
AI Read Science
Must-Reading Tree
Example
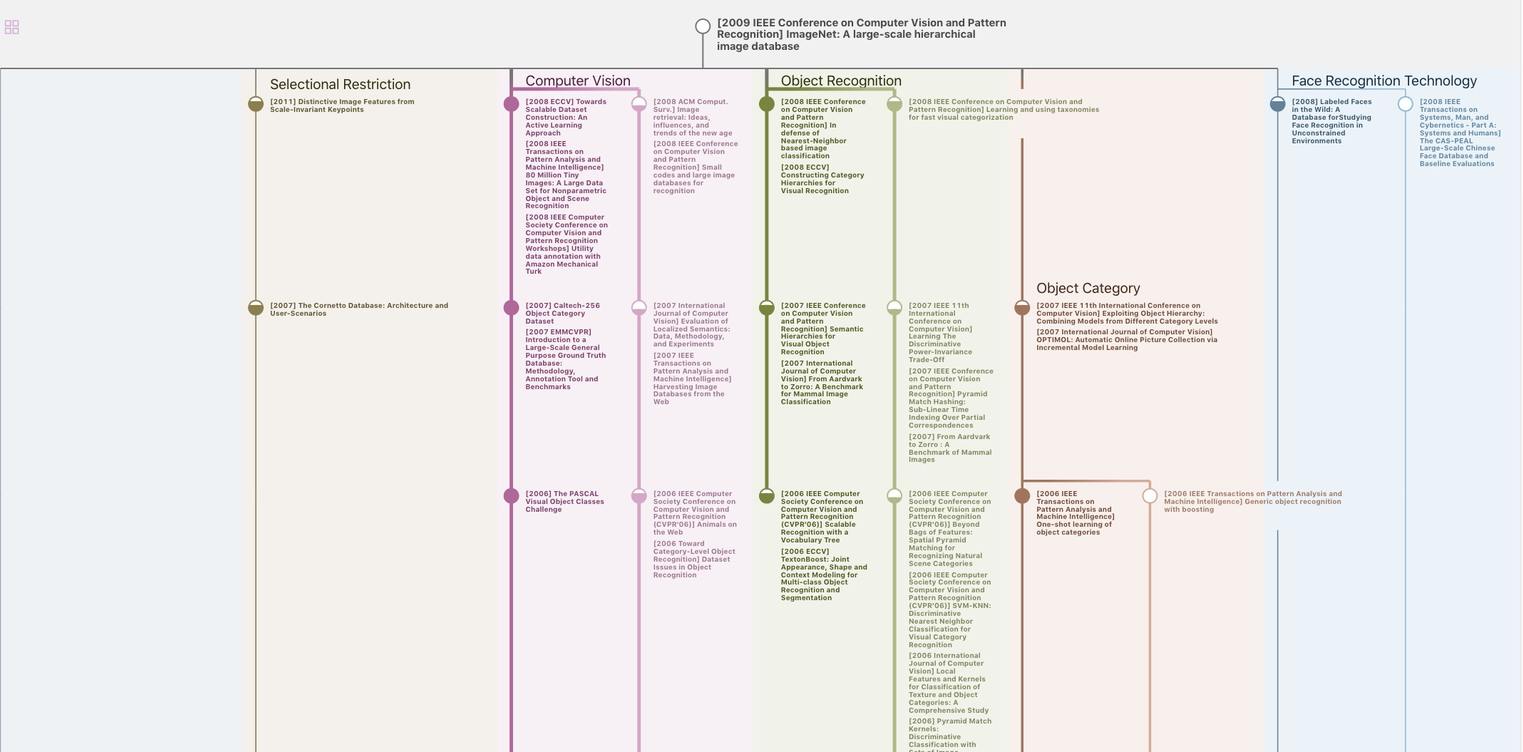
Generate MRT to find the research sequence of this paper
Chat Paper
Summary is being generated by the instructions you defined