Intelligent circumferential deformation prediction of structures in PCCP-E with a large diameter under internal water overpressure based on prototype experiments and Inception-ResNet-LSTM
Engineering Structures(2024)
摘要
Prestressed Concrete Cylinder Pipe (PCCP) is extensively employed in long-distance water transmission projects, owing to its notable pressure-bearing capacity and durability. Nonetheless, the operation under overpressure conditions poses a risk of structural damage and functional failure to PCCP during its service life. Consequently, accurate prediction of the deformation characteristics of overpressure operation with the structural deformation data under standard water pressure is of great importance for ensuring the safety and reliability of the project. However, it is a challenge to obtain spatiotemporal features for traditional algorithms. As a consequence, a novel deep learning model, i.e. Inception-ResNet-LSTM, is put forward to forecast PCCP deformation. It integrates the Inception, ResNet, and Long Short-Term Memory (LSTM). Based on the prototype experiments, the monitoring was undertaken on the deformations of the inner and outer concrete layers, the steel cylinders, and the prestressed steel wire under internal water pressures of 0 MPa, 0.2 MPa, 0.4 MPa, 0.6 MPa, 0.8 MPa, 1.0 MPa, and 1.15 MPa. In the case study, the deformation data of different structures are input into Inception-ResNet-LSTM to establish a model. For all the structures, the R2 (coefficient of determination) of the Inception-ResNet-LSTM model exceeds 0.97. Based on the results, it is demonstrated that the model has captured the spatiotemporal features from the monitoring data. In comparison to models based on DL and ML algorithms, Inception-ResNet-LSTM demonstrates superior performance, exhibiting heightened accuracy and enhanced generalization capabilities. It provides a new method for PCCP deformation estimation under overpressure.
更多查看译文
关键词
PCCP deformation,Overpressure,Inception,ResNet,LSTM,Deep learning
AI 理解论文
溯源树
样例
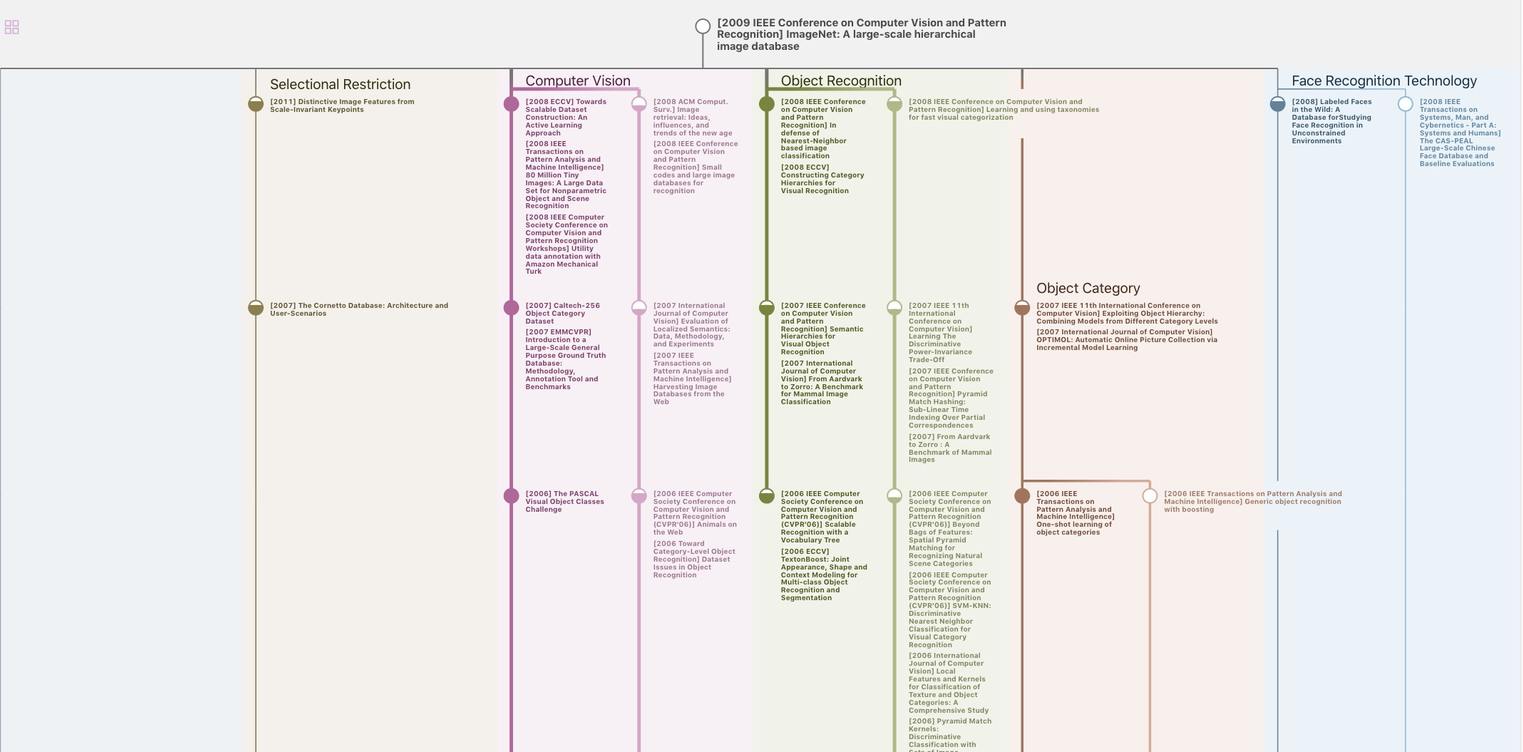
生成溯源树,研究论文发展脉络
Chat Paper
正在生成论文摘要