Methodology and real-world applications of dynamic uncertain causality graph for clinical diagnosis with explainability and invariance
Artificial Intelligence Review(2024)
Abstract
AI-aided clinical diagnosis is desired in medical care. Existing deep learning models lack explainability and mainly focus on image analysis. The recently developed Dynamic Uncertain Causality Graph (DUCG) approach is causality-driven, explainable, and invariant across different application scenarios, without problems of data collection, labeling, fitting, privacy, bias, generalization, high cost and high energy consumption. Through close collaboration between clinical experts and DUCG technicians, 46 DUCG models covering 54 chief complaints were constructed. Over 1,000 diseases can be diagnosed without triage. Before being applied in real-world, the 46 DUCG models were retrospectively verified by third-party hospitals. The verified diagnostic precisions were no less than 95
MoreTranslated text
Key words
Diagnosis,Causality,Probabilistic reasoning,Explainability,Counterfactual inference
AI Read Science
Must-Reading Tree
Example
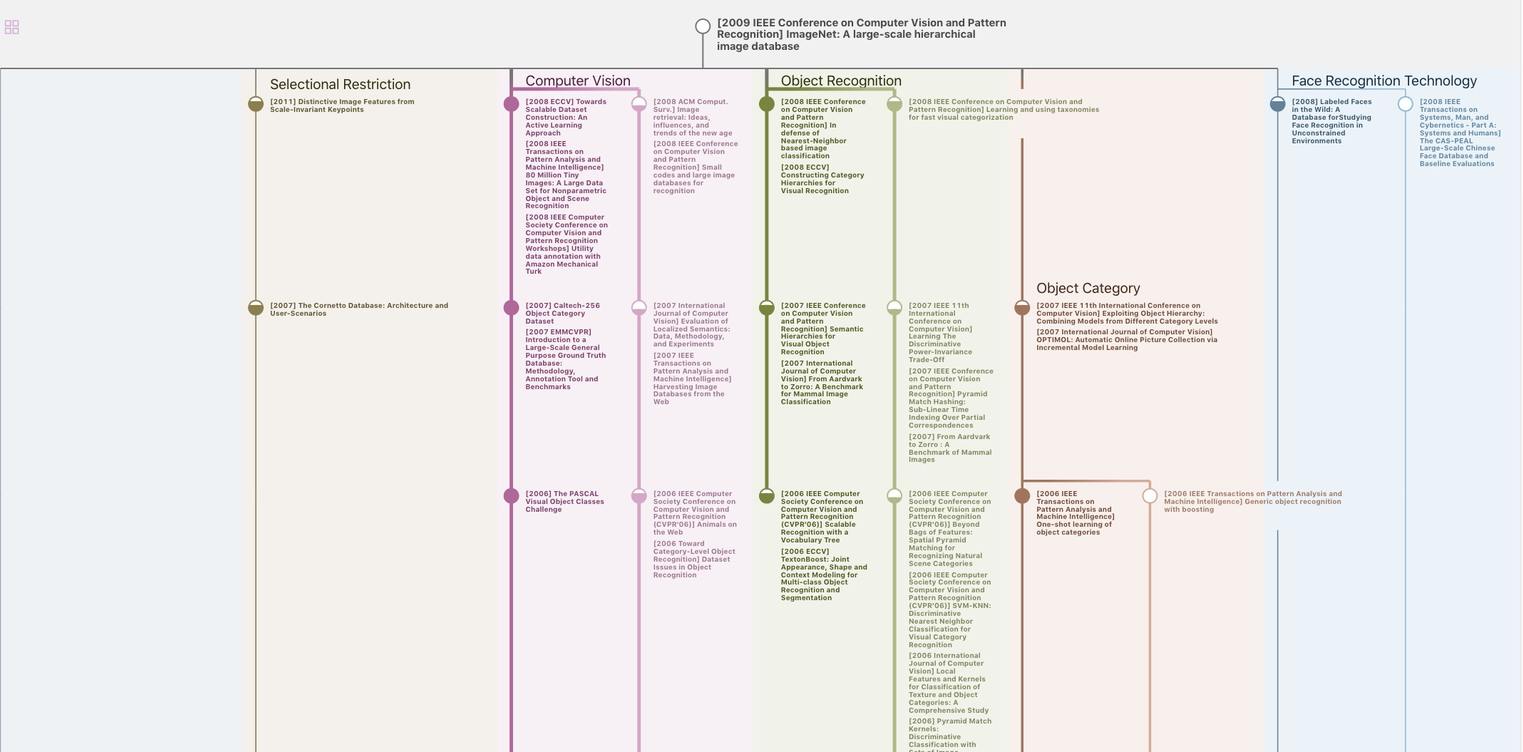
Generate MRT to find the research sequence of this paper
Chat Paper
Summary is being generated by the instructions you defined