An attention-based deep learning model considering data contamination for energy management system application of hybrid vehicle
Computers and Electrical Engineering(2024)
Abstract
Hybrid vehicles are pivotal in transitioning to sustainable transportation, but effective energy management is challenged by data contamination. This paper proposes a novel approach, utilizing deep learning trained with diverse data from existing energy management systems, to assess its adaptability to varying contamination levels. The training dataset covers emission test cycles, loadings, and driving styles, with dimensionality and noise reduction applied to control parameters. A Long Short-Term Memory (LSTM) prediction model with attention mechanism forecasts power demand, outperforming five other deep learning models. The study demonstrates exceptional performance, achieving an average Root Mean Square Error (RMSE) of 4.9761, surpassing RMSE values from 7.9497 to 9.1933. Real driving conditions yield a Mean Absolute Error (MAE) of 3.965 and RMSE of 5.908, highlighting the model's adaptability and predictive accuracy despite data contamination. Such robustness makes it valuable for hybrid electric vehicle energy management systems research and development.
MoreTranslated text
Key words
Energy management system,Deep learning,Attention mechanism,LSTM,Noise reduction
AI Read Science
Must-Reading Tree
Example
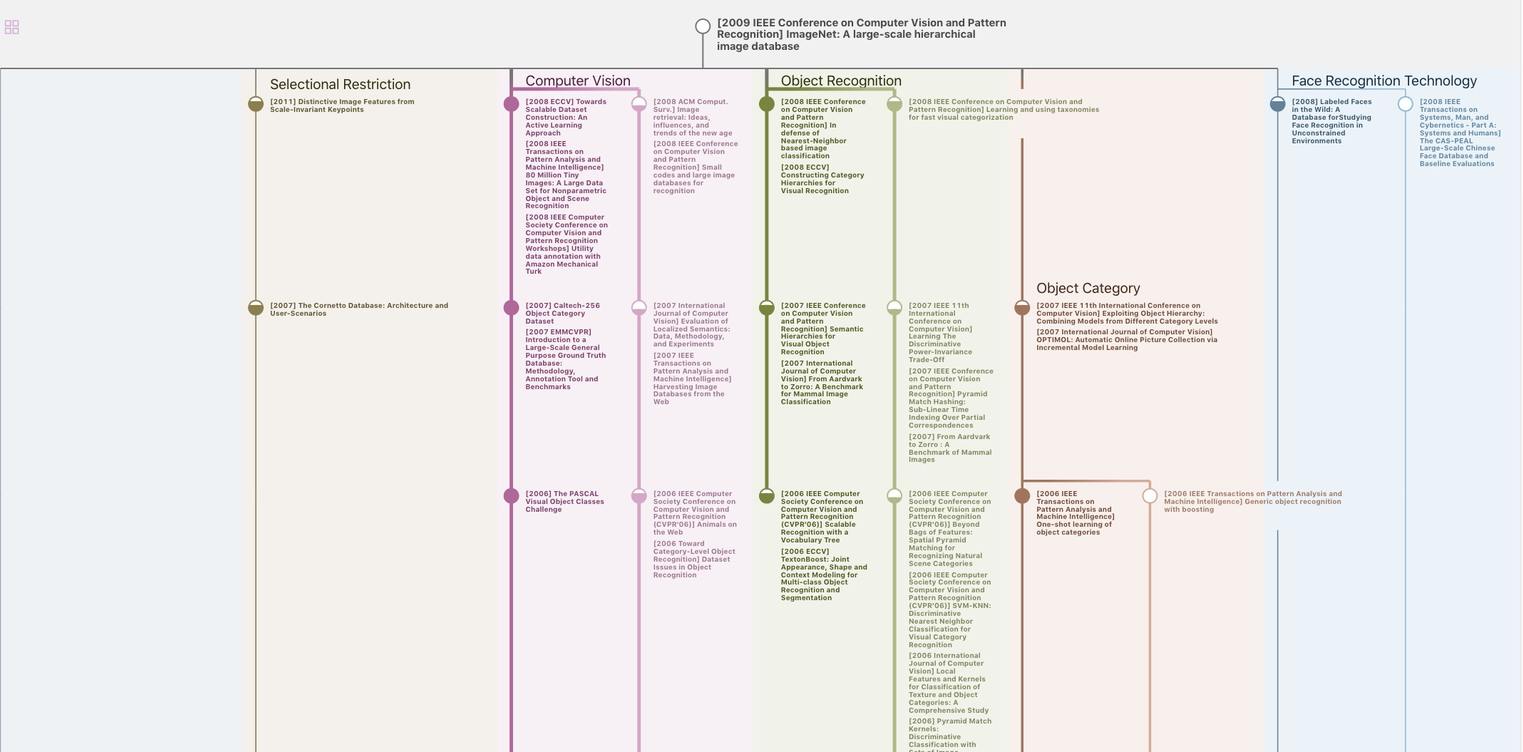
Generate MRT to find the research sequence of this paper
Chat Paper
Summary is being generated by the instructions you defined