Cyberbullying Detection Using Deep Learning: A Comparative Study.
ICCR(2024)
Abstract
Cyberbullying has become a serious issue with the rise in social media usage, especially among adolescents. Victims of cyberbullying often suffer from psychological distress, anxiety, depression and even suicidal tendencies. With the rapid growth of user-generated content online, manual detection of cyberbullying is extremely difficult. Hence, automated cyber-bullying detection systems are needed. In recent years, deep learning models have emerged as a promising technique for cyberbullying detection. This paper provides a comprehensive review of the use of deep learning for cyberbullying detection. The key contributions are: (1) a taxonomy of cyberbullying detection techniques, (2) a review of datasets used for model training and evaluation, (3) a comparative analysis of various deep learning models including CNN, RNN, LSTM, GRU and Transformer- based models, (4) a discussion of key challenges and limitations, and (5) suggestions for future research directions. The paper provides a critical perspective on model performance across multiple datasets. Key findings indicate RNNs and specifically LSTM and GRU models demonstrate state-of-the-art performance for sequential cyberbullying detection. The study concludes that deep ensemble models combining LSTMs and pretrained contextual embeddings show significant promise to advance the field.
MoreTranslated text
AI Read Science
Must-Reading Tree
Example
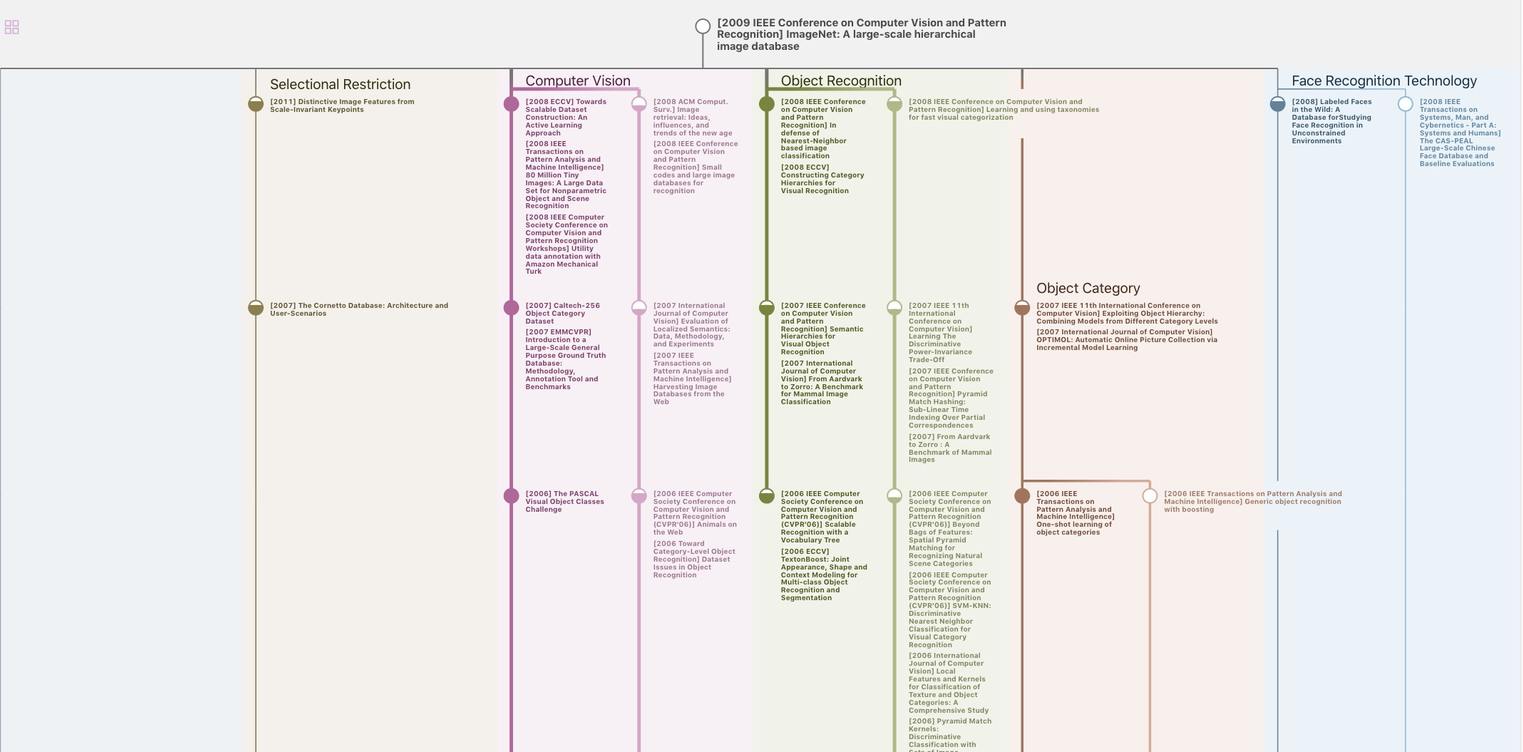
Generate MRT to find the research sequence of this paper
Chat Paper
Summary is being generated by the instructions you defined