(De/Re)-Composition of Data-Parallel Computations via Multi-Dimensional Homomorphisms
ACM Transactions on Programming Languages and Systems(2024)
Abstract
Data-parallel computations, such as linear algebra routines (BLAS) and stencil computations, constitute one of the most relevant classes in parallel computing, e.g., due to their importance for deep learning. Efficiently de-composing such computations for the memory and core hierarchies of modern architectures and re-composing the computed intermediate results back to the final result – we say (de/re)-composition for short – is key to achieve high performance for these computations on, e.g., GPU and CPU. Current high-level approaches to generating data-parallel code are often restricted to a particular subclass of data-parallel computations and architectures (e.g., only linear algebra routines on only GPU, or only stencil computations), and/or the approaches rely on a user-guided optimization process for a well-performing (de/re)-composition of computations, which is complex and error prone for the user. We formally introduce a systematic (de/re)-composition approach, based on the algebraic formalism of Multi-Dimensional Homomorphisms (MDHs) 1 . Our approach is designed as general enough to be applicable to a wide range of data-parallel computations and for various kinds of target parallel architectures. To efficiently target the deep and complex memory and core hierarchies of contemporary architectures, we exploit our introduced (de/re)-composition approach for a correct-by-construction, parametrized cache blocking and parallelization strategy. We show that our approach is powerful enough to express, in the same formalism, the (de/re)-composition strategies of different classes of state-of-the-art approaches (scheduling-based, polyhedral, etc), and we demonstrate that the parameters of our strategies enable systematically generating code that can be fully automatically optimized (auto-tuned) for the particular target architecture and characteristics of the input and output data (e.g., their sizes and memory layouts). Particularly, our experiments confirm that via auto-tuning, we achieve higher performance than state-of-the-art approaches, including hand-optimized solutions provided by vendors (such as NVIDIA cuBLAS/cuDNN and Intel oneMKL/oneDNN), on real-world data sets and for a variety of data-parallel computations, including: linear algebra routines, stencil and quantum chemistry computations, data mining algorithms, and computations that recently gained high attention due to their relevance for deep learning.
MoreTranslated text
AI Read Science
Must-Reading Tree
Example
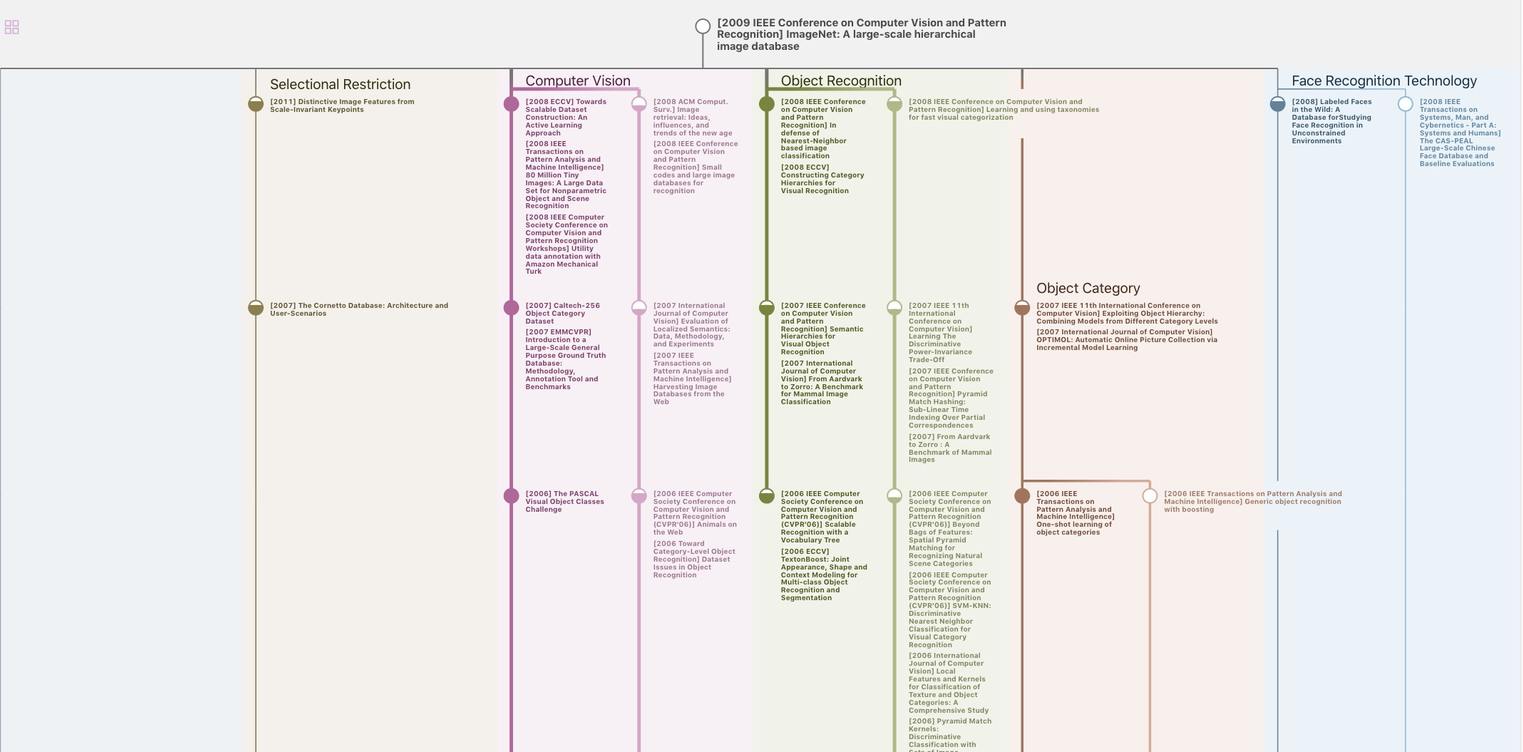
Generate MRT to find the research sequence of this paper
Chat Paper
Summary is being generated by the instructions you defined