Multimodal Fusion Network for 3-D Lane Detection.
IEEE transactions on neural networks and learning systems(2024)
摘要
3-D lane detection is a challenging task due to the diversity of lanes, occlusion, dazzle light, and so on. Traditional methods usually use highly specialized handcrafted features and carefully designed postprocessing to detect them. However, these methods are based on strong assumptions and single modal so that they are easily scalable and have poor performance. In this article, a multimodal fusion network (MFNet) is proposed through using multihead nonlocal attention and feature pyramid for 3-D lane detection. It includes three parts: multihead deformable transformation (MDT) module, multidirectional attention feature pyramid fusion (MA-FPF) module, and top-view lane prediction (TLP) ones. First, MDT is presented to learn and mine multimodal features from RGB images, depth maps, and point cloud data (PCD) for achieving optimal lane feature extraction. Then, MA-FPF is designed to fuse multiscale features for presenting the vanish of lane features as the network deepens. Finally, TLP is developed to estimate 3-D lanes and predict their position. Experimental results on the 3-D lane synthetic and ONCE-3DLanes datasets demonstrate that the performance of the proposed MFNet outperforms the state-of-the-art methods in both qualitative and quantitative analyses and visual comparisons.
更多查看译文
关键词
3-D lane detection,deformable transformation,feature pyramid,multihead attention,multimodal fusion
AI 理解论文
溯源树
样例
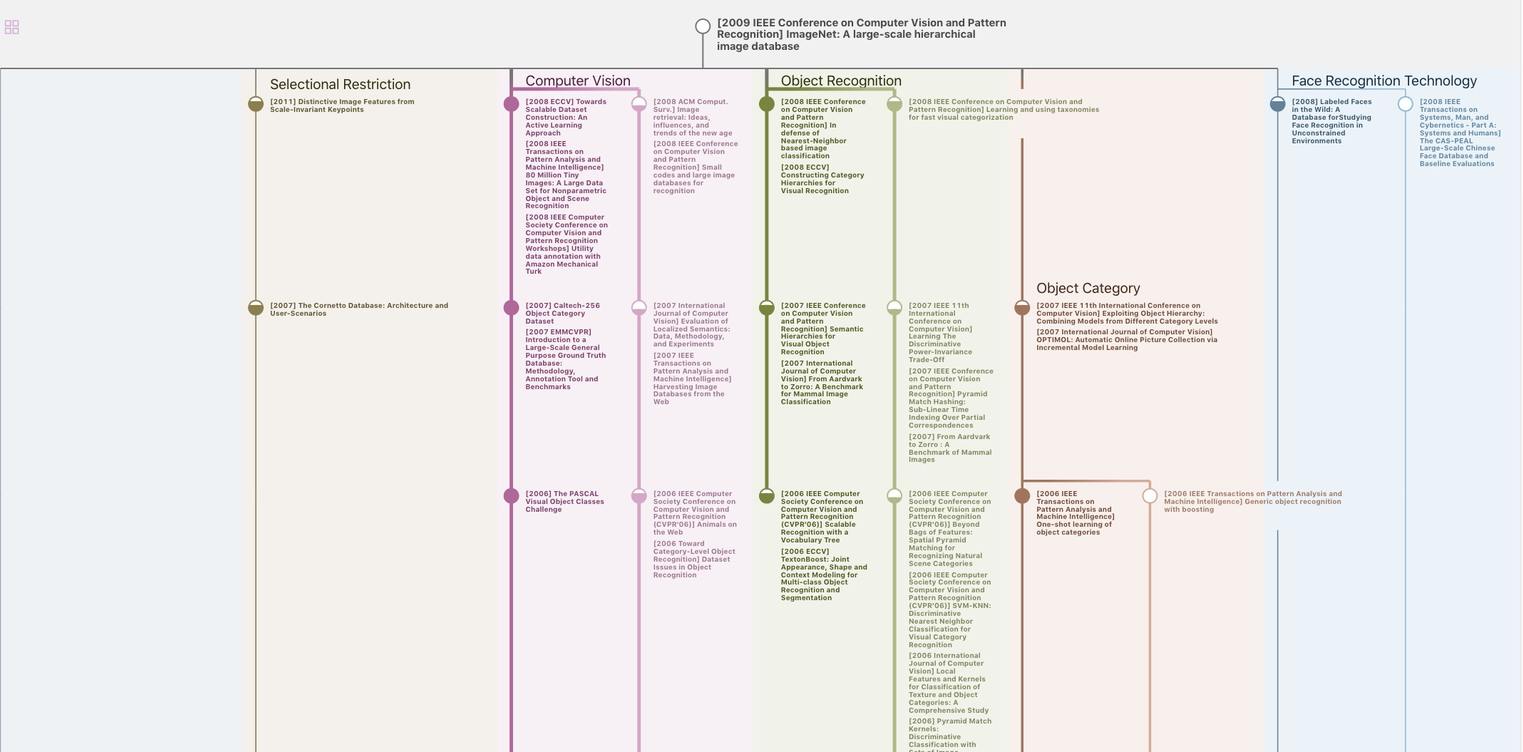
生成溯源树,研究论文发展脉络
Chat Paper
正在生成论文摘要