Interrelated Dense Pattern Detection in Multilayer Networks
IEEE Transactions on Knowledge and Data Engineering(2024)
摘要
Given a heterogeneous multilayer network with various connections in pharmacology, how can we detect components with intensive interactions and strong dependencies? Can we accurately capture suspicious groups in a multi-lot transaction network under camouflage? These challenges related to dense subgraph detection have been extensively studied in simple graphs (such as bipartite graph, multi-view network) but remain under-explored on complex networks. Existing methods struggle to effectively handle the
intricate dependencies
, let alone accurately identify the
interrelated dense connected patterns
within a series of complex heterogeneous networks. In this paper, we propose
InDuen
, a novel algorithm designed to detect interrelated densest subgraphs in multilayer networks through joint optimization of coupled factorization and local search for an elaborate-designed joint density measure. It is
(a)
effective for both large synthetic and real networks,
(b)
resistant to camouflage for anomaly detection, and
(c)
linearly scalable. Experimental results demonstrate that
InDuen
outperforms the state-of-the-art baselines in accurately detecting interrelated densest subgraphs under various settings. Furthermore,
InDuen
uncovers some intriguing patterns in real-world data, i.e., closely cooperated academic groups and interrelated dependent functional components in biology-net.
InDuen
achieves more than
$35 \times$
speedup compared to the SOTA method
Destine
.
更多查看译文
关键词
Multilayer network,dense subgraph detection,interrelated pattern mining,algorithm design
AI 理解论文
溯源树
样例
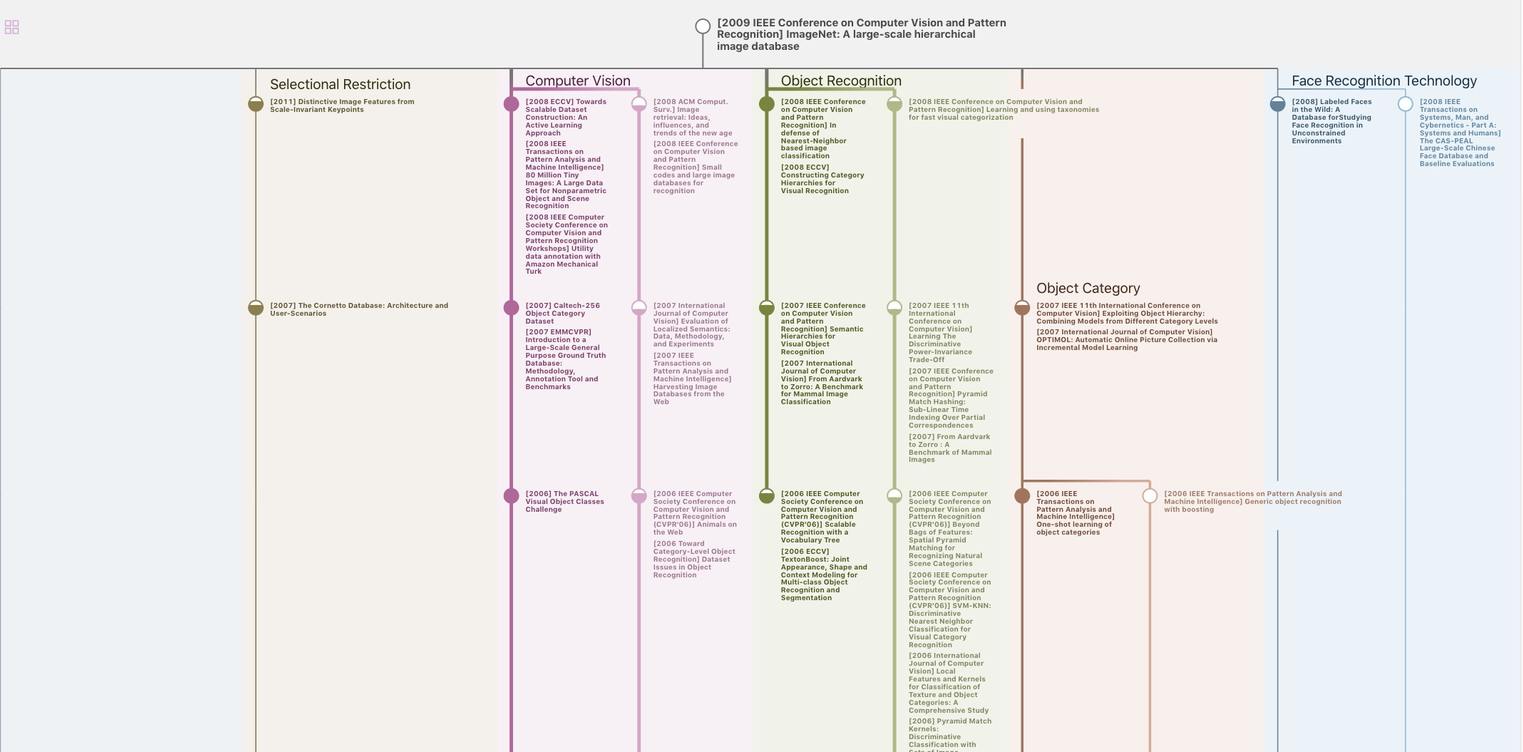
生成溯源树,研究论文发展脉络
Chat Paper
正在生成论文摘要