Optimized Online Remaining Useful Life Prediction for Nuclear Circulating Water Pump Considering Time-Varying Degradation Mechanism
IEEE Transactions on Industrial Informatics(2024)
摘要
Remaining useful life (RUL) prediction is crucial for ensuring machine operating safety and reducing maintenance costs in nuclear power plants. Existing RUL prediction methods generally use run-to-failure data or known degradation mechanisms to establish a static model for degradation process characterization. However, the inherent degradation mechanisms of machines are time-varying, and a static model may only cover part of the degradation, resulting in an inaccurate RUL result. Hence, we propose an optimized online RUL prediction considering time-varying degradation mechanisms. The degradation model type (discrete variables) and boundary/initial conditions (continuous variables) are first set as the main variables affecting the approximation of the time-varying degradation mechanism. The RUL prediction is then formulated as a feedback-decision process through interacting with the anomaly data stream, in which variables are jointly optimized with reinforcement learning by minimizing the approximation error. Based on the degradation model established with optimized variables, the RUL can finally be deduced. The proposed method is validated by a run-to-failure dataset collected in a nuclear circulating water pump test bench.
更多查看译文
关键词
Feedback-decision process,nuclear circulating water pump,reinforcement learning,remaining useful life (RUL) prediction,time-varying degradation mechanism
AI 理解论文
溯源树
样例
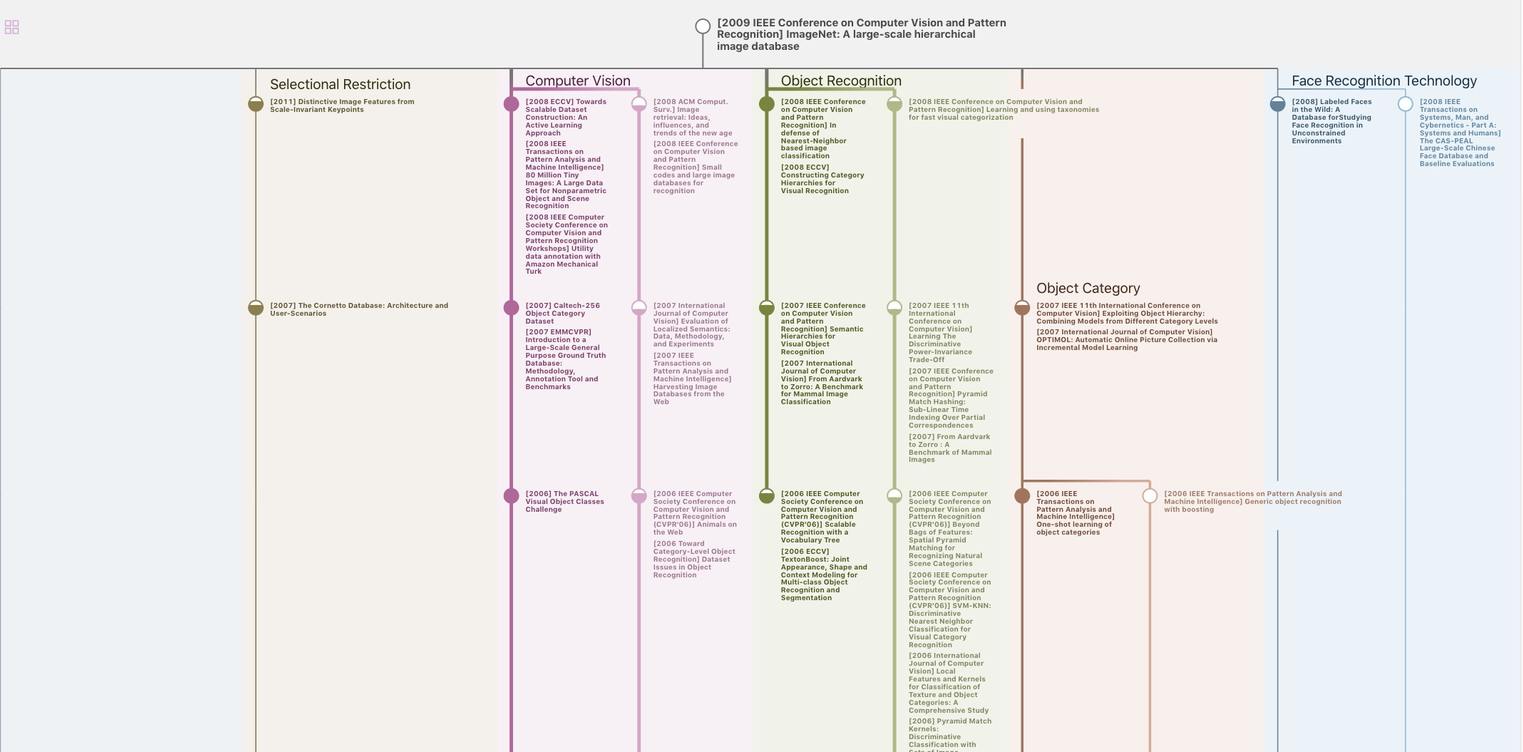
生成溯源树,研究论文发展脉络
Chat Paper
正在生成论文摘要