Decision model for durable clinical benefit from front- or late-line immunotherapy alone or with chemotherapy in non-small cell lung cancer
Med(2024)
摘要
Background
Predictive biomarkers and models of immune checkpoint inhibitors (ICIs) have been extensively studied in non-small cell lung cancer (NSCLC). However, evidence for many biomarkers remains inconclusive, and the opaqueness of machine learning models hinders practicality. We aimed to provide compelling evidence for biomarkers and develop a transparent decision tree model.
Methods
We consolidated data from 3,288 ICI-treated patients with NSCLC across real-world multicenter, public cohorts and the Choice-01 trial (ClinicalTrials.gov: NCT03856411). Over 50 features were examined for predicting durable clinical benefits (DCBs) from ICIs. Noteworthy biomarkers were identified to establish a decision tree model. Additionally, we explored the tumor microenvironment and peripheral CD8+ programmed death-1 (PD-1)+ T cell receptor (TCR) profiles.
Findings
Multivariate logistic regression analysis identified tumor histology, PD-ligand 1 (PD-L1) expression, tumor mutational burden, line, and regimen of ICI treatment as significant factors. Mutation subtypes of EGFR, KRAS, KEAP1, STK11, and disruptive TP53 mutations were associated with DCB. The decision tree (DT10) model, using the ten clinicopathological and genomic markers, showed superior performance in predicting DCB in the training set (area under the curve [AUC] = 0.82) and consistently outperformed other models in test sets. DT10-predicted-DCB patients manifested longer survival, an enriched inflamed tumor immune phenotype (67%), and higher peripheral TCR diversity, whereas the DT10-predicted-NDB (non-durable benefit) group showed an enriched desert immune phenotype (86%) and higher peripheral TCR clonality.
Conclusions
The model effectively predicted DCB after front-/subsequent-line ICI treatment, with or without chemotherapy, for squamous and non-squamous lung cancer, offering clinicians valuable insights into efficacy prediction using cost-effective variables.
Funding
This study was supported by the National Key R&D Program of China.
更多查看译文
关键词
immune checkpoint inhibitors,non-small cell lung cancer,decision tree model,machine learning,predictive model
AI 理解论文
溯源树
样例
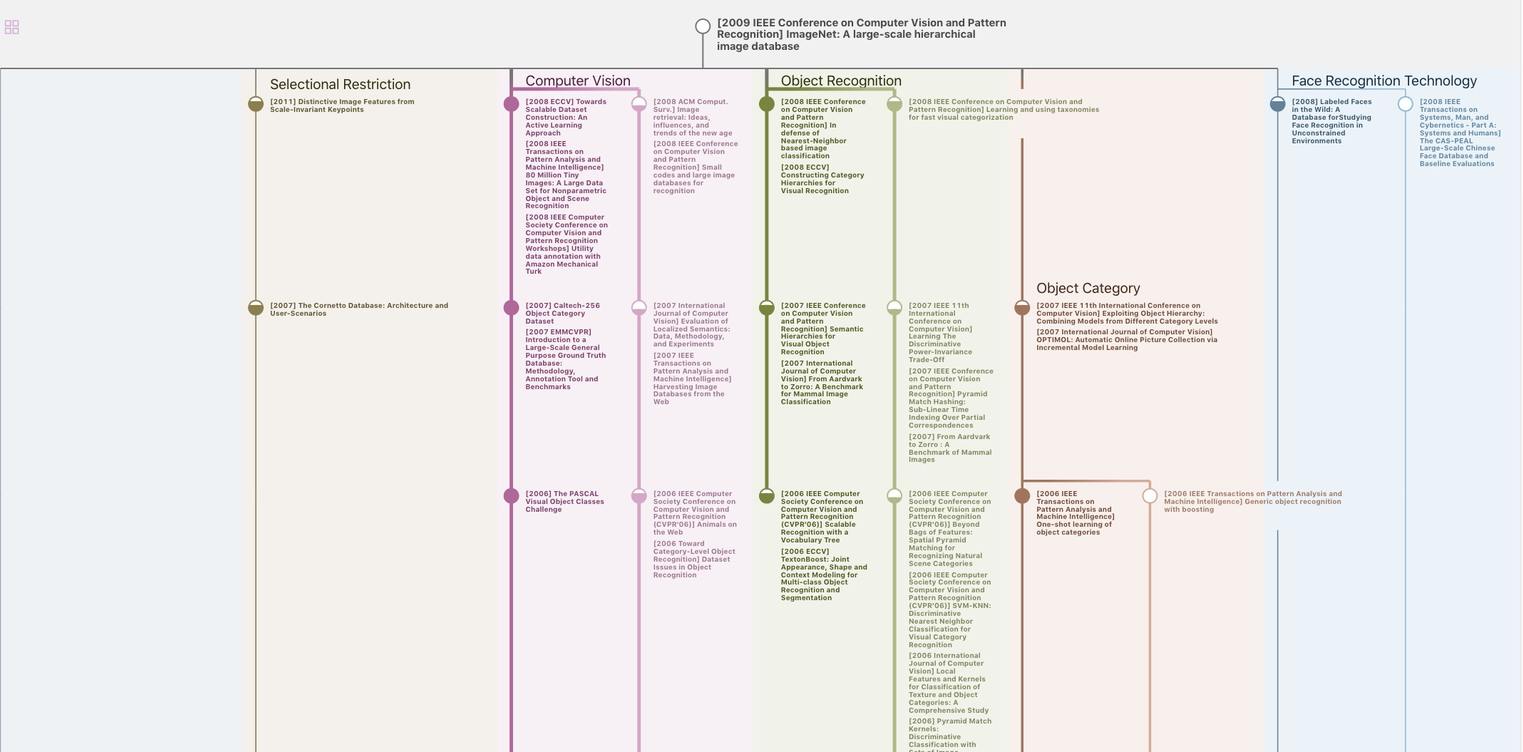
生成溯源树,研究论文发展脉络
Chat Paper
正在生成论文摘要