Merging Multi-Level Evidential Observations for Dynamic Reliability Assessment of Hierarchical Multi-State Systems: A Dynamic Bayesian Network Approach
Reliability Engineering & System Safety(2024)
摘要
Dynamic reliability assessment has offered a new paradigm for engineered systems to integrate multi-level observations to update the reliability measures of a specific running system. The existent work on dynamic reliability assessment can only leverage precise observations and cannot be straightforwardly implemented in scenarios where observations are imprecise. However, the observations are inevitably imprecise owing to the limited accuracy of inspection techniques and vague judgments of the system state. In engineering scenarios, multi-state systems (MSSs) with a hierarchical structure are commonly existent, and the imprecise observations can be collected across multiple physical levels of the system. In this article, the uncertainty associated with imprecise observations is characterized by the evidence theory, and it can be therefore regarded as an evidential form. A dynamic Bayesian network (DBN) model is utilized to evaluate the reliability of hierarchical MSSs with multi-level evidential observations. Subsequently, the evidence theory is implemented to quantify epistemic uncertainties associated with imprecise observations. These observations, sourced from multiple physical levels, are merged by the DBN model using the Dempster rule of combination (DRC) to update the reliability of a specific running system in a dynamic fashion. The feasibility and correctness of the proposed method have been demonstrated through a numerical case and a real engineering case of a kerosene filling control system (KFCS), and the result indicates that the proposed DBN-based algorithm methods have high applicability and generalizability.
更多查看译文
关键词
Multi-state system,dynamic reliability assessment,imprecise reliability data,evidence theory,dynamic Bayesian network
AI 理解论文
溯源树
样例
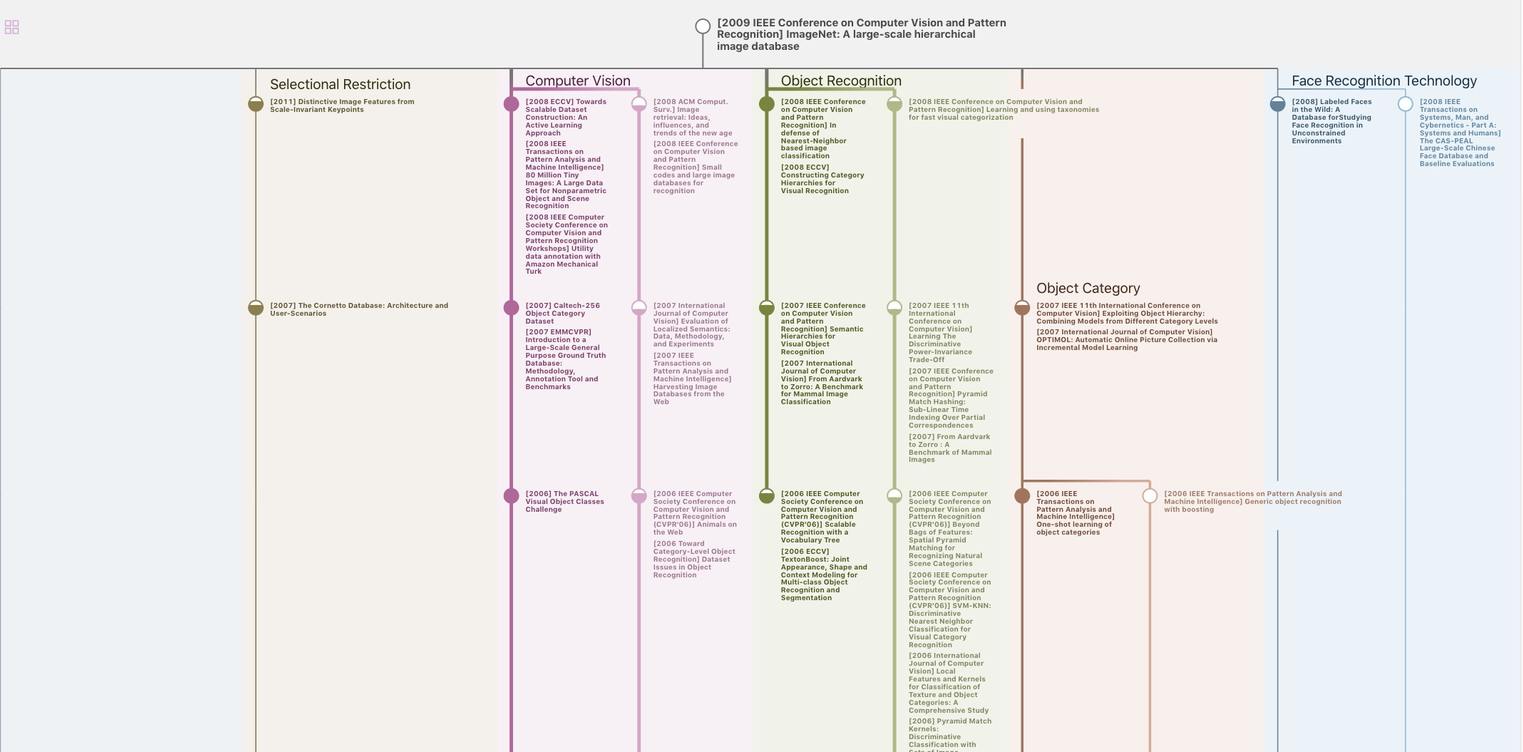
生成溯源树,研究论文发展脉络
Chat Paper
正在生成论文摘要