SpatialDiffusion: Predicting Spatial Transcriptomics with Denoising Diffusion Probabilistic Models
crossref(2024)
摘要
Spatial Transcriptomics (ST) allows deep characterization of the 2D organization of expression data within tissue slices. The ST technology provides a tissue contextualization of deep single-cell profiles. Recently, numerous computational and machine learning methods have addressed challenges such as data quality, augmentation, annotation, and the development of integrative platforms for data analysis. In contrast, here we ask whether unseen spatial transcriptomics data can be predicted and if we can interpolate novel transcriptomic slices. To this end, we adopt a denoising diffusion probabilistic-based model (DDPM) to demonstrate the learning of generative ST models for several tissues. Furthermore, our generative diffusion model interpolates (predicts) unseen slices located “between” the collected finite number of ST slices. This methodology set the stage for learning predictive deep 3D models of tissues from a finite number of spatial transcriptomics slices, thus harboring the advent of AI-augmented spatial transcriptomics.
### Competing Interest Statement
The authors have declared no competing interest.
更多查看译文
AI 理解论文
溯源树
样例
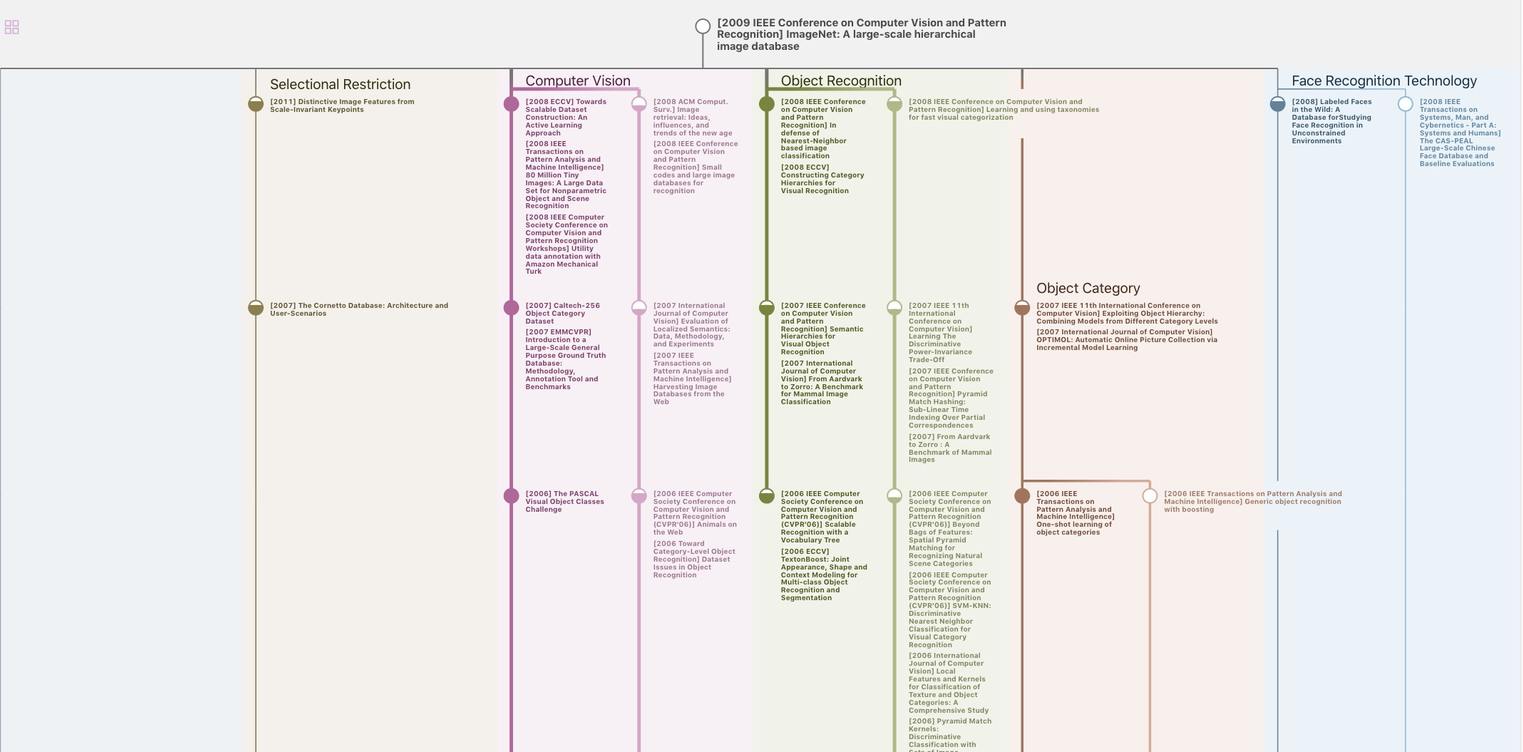
生成溯源树,研究论文发展脉络
Chat Paper
正在生成论文摘要