Contextual Embeddings and Graph Convolutional Networks for Concept Prerequisite Learning
SAC '24 Proceedings of the 39th ACM/SIGAPP Symposium on Applied Computing(2024)
摘要
Concept prerequisite learning (CPL) plays a crucial role in education. The objective of CPL is to predict prerequisite relations between different concepts. In this paper, we present a new approach for CPL using Sentence Transformers and Relational Graph Convolutional Networks (R-GCNs). This approach creates concept embeddings from single-sentence definitions extracted from Wikipedia using a Sentence Transformer. These embeddings are then used as an input feature matrix for the R-GCN, in addition to a graph structure that distinguishes prerequisites and non-prerequisites as distinct link types. Furthermore, the R-GCN is optimized simultaneously on CPL and concept domain classification to enhance prerequisite prediction generalization for unseen domains. Extensive experiments on the AL-CPL dataset show the effectiveness of our approach for the in-domain and cross-domain settings, as it outperforms the State-Of-The-Art (SOTA) methods on this dataset. Finally, we introduce a novel data split algorithm for this task to address a methodological issue found in previous studies. The new data split algorithm makes CPL more challenging to solve, but also more realistic as it excludes simple inferences by transitivity.
更多查看译文
AI 理解论文
溯源树
样例
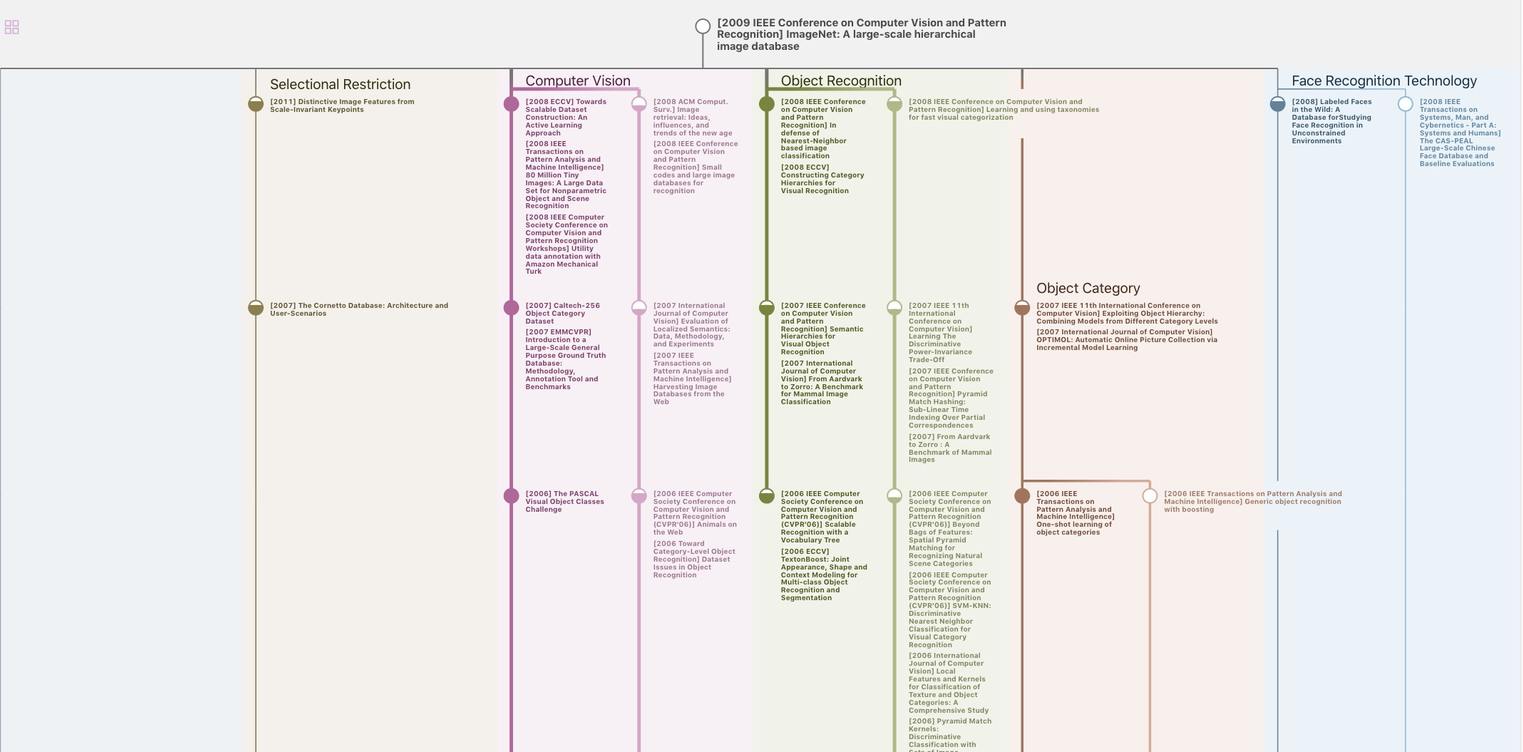
生成溯源树,研究论文发展脉络
Chat Paper
正在生成论文摘要