Human-like Learning in Temporally Structured Environments.
AAAI Spring Symposia(2024)
Abstract
Natural environments have correlations at a wide range of timescales. Human cognition is tuned to this temporal structure, as seen by power laws of learning and memory, and by spacing effects whereby the intervals between repeated training data affect how long knowledge is retained. Machine learning is instead dominated by batch iid training or else relatively simple nonstationarity assumptions such as random walks or discrete task sequences. The main contributions of our work are: (1) We develop a Bayesian model formalizing the brain's inductive bias for temporal structure and show our model accounts for key features of human learning and memory. (2) We translate the model into a new gradient-based optimization technique for neural networks that endows them with human-like temporal inductive bias and improves their performance in realistic nonstationary tasks. Our technical approach is founded on Bayesian inference over 1/f noise, a statistical signature of many natural environments with long-range, power law correlations. We derive a new closed-form solution to this problem by treating the state of the environment as a sum of processes on different timescales and applying an extended Kalman filter to learn all timescales jointly. We then derive a variational approximation of this model for training neural networks, which can be used as a drop-in replacement for standard optimizers in arbitrary architectures. Our optimizer decomposes each weight in the network as a sum of subweights with different learning and decay rates and tracks their joint uncertainty. Thus knowledge becomes distributed across timescales, enabling rapid adaptation to task changes while retaining long-term knowledge and avoiding catastrophic interference. Simulations show improved performance in environments with realistic multiscale nonstationarity. Finally, we present simulations showing our model gives essentially parameter-free fits of learning, forgetting, and spacing effects in human data. We then explore the analogue of human spacing effects in a deep net trained in a structured environment where tasks recur at different rates and compare the model's behavioral properties to those of people.
MoreTranslated text
AI Read Science
Must-Reading Tree
Example
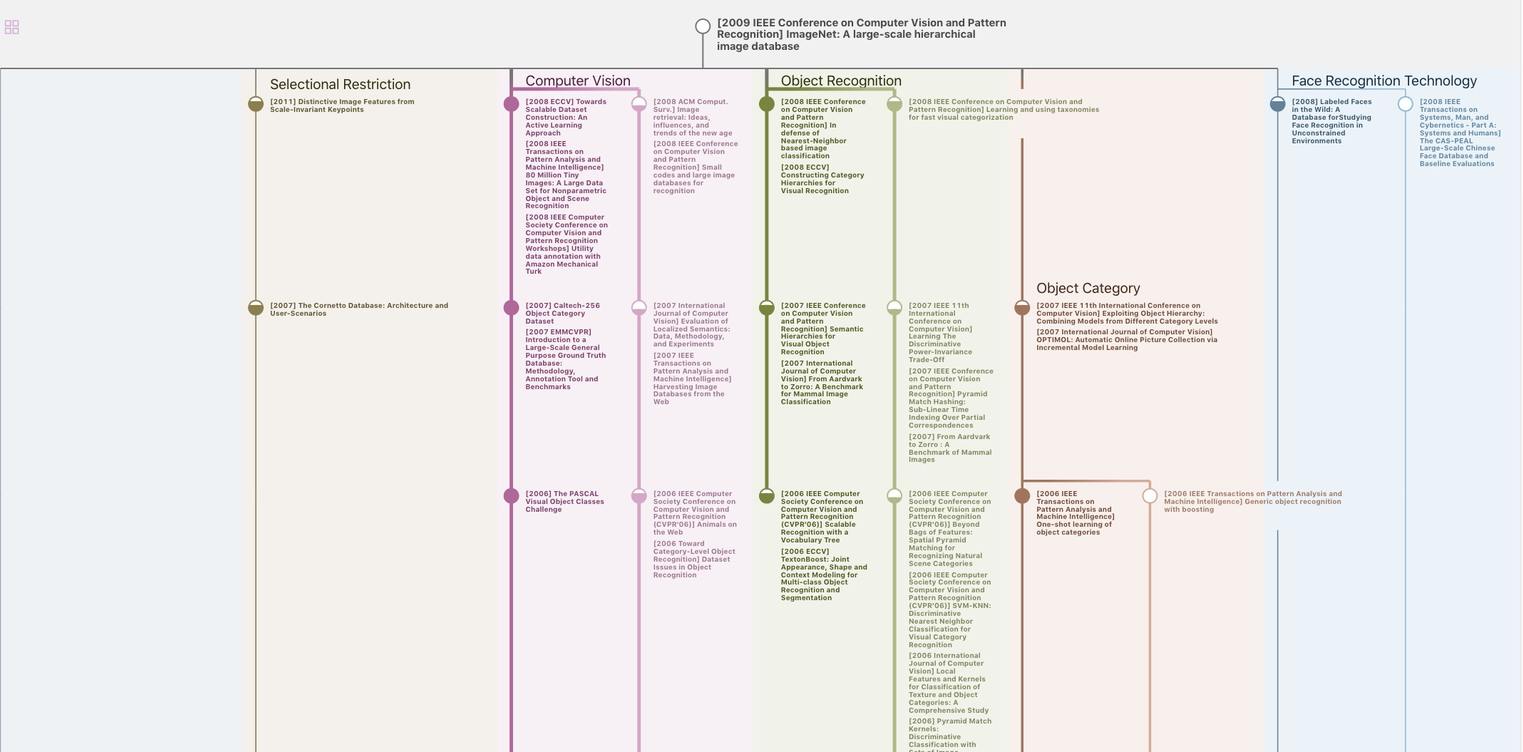
Generate MRT to find the research sequence of this paper
Chat Paper
Summary is being generated by the instructions you defined