Bridging Global Context Interactions for High-Fidelity Pluralistic Image Completion.
IEEE transactions on pattern analysis and machine intelligence(2024)
摘要
We introduce PICFormer, a novel framework for Pluralistic Image Completion using a transFormer based architecture, that achieves both high quality and diversity at a much faster inference speed. Our key contribution is to introduce a code-shared codebook learning using a restrictive CNN on small and non-overlapping receptive fields (RFs) for the local visible token representation. This results in a compact yet expressive discrete representation, facilitating efficient modeling of global visible context relations by the transformer. Unlike the prevailing autoregressive approaches, we proposed to sample all tokens simultaneously, leading to more than 100× faster inference speed. To enhance appearance consistency between visible and generated regions, we further propose a novel attention-aware layer (AAL), designed to better exploit distantly related high-frequency features. Through extensive experiments, we demonstrate that the efficiently learns semantically-rich discrete codes, resulting in significantly improved image quality. Moreover, our diverse image completion framework surpasses state-of-the-art methods on multiple image completion datasets. The project page is available at https://chuanxiaz.com/picformer/.
更多查看译文
关键词
Image Completion,Codebook Learning,Image Editing,Transformer
AI 理解论文
溯源树
样例
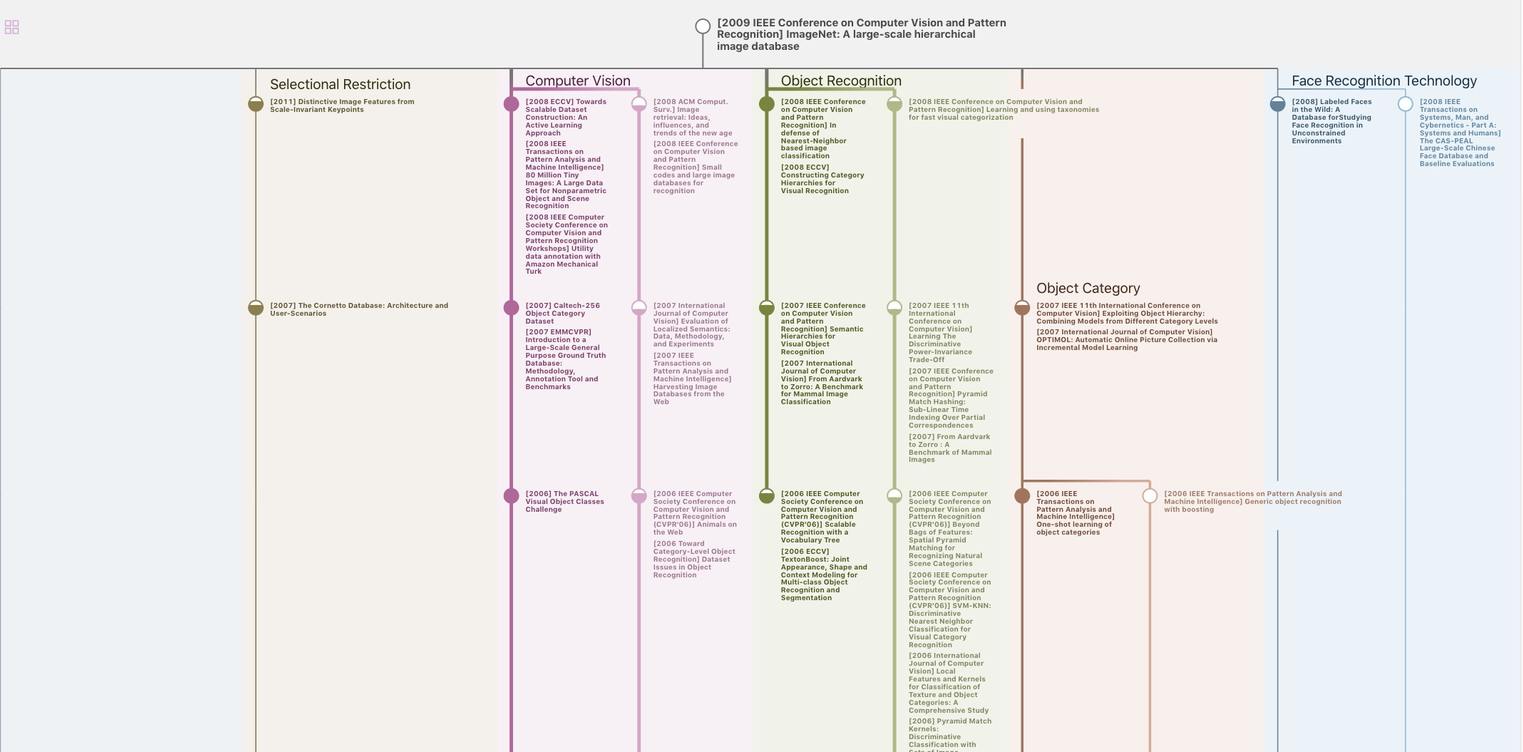
生成溯源树,研究论文发展脉络
Chat Paper
正在生成论文摘要