Deep Multi-Task Multi-Agent Reinforcement Learning Based Joint Bidding and Pricing Strategy of Price-Maker Load Serving Entity
IEEE Transactions on Power Systems(2024)
Abstract
Deep reinforcement learning (DRL)-based methods have been widely used to learn optimal bidding and/or pricing strategies of load serving entities (LSEs) in electricity markets. However, previous studies on joint bidding and pricing (JBP) problem have been limited to model-based methods for price-maker LSEs or model-free methods for price-taker LSEs. In the context of addressing this research gap, this paper explores for the very first time a model-free multi-agent reinforcement learning (MARL)-based approach for the price-maker JBP problem. The original problem is first formulated as a Decentralized Partially Observable Markov Decision Process (Dec-POMDP), where multiple agents are trained to find the optimal joint strategy in a fully cooperative setting. In order to overcome the challenges such as credit assignment and coordination, this paper proposes a parallelizable deep MT-MARL framework by incorporating multi-task learning (MTL) with MARL. Furthermore, an easily implementable multi-task multi-agent (MTMA) version of soft actor-critic (SAC), named as MTMA-SAC, is proposed to solve the Dec-POMDP efficiently based on the deep MT-MARL framework. The effectiveness, superiority and scalability of the proposed method is validated by numerical studies on systems of different scales. Case studies provide insightful analysis of some interesting characteristics caused by price-maker and line congestion.
MoreTranslated text
Key words
Electricity market,load serving entity,price-maker,bidding,pricing,multi-agent reinforcement learning,multi-task learning
AI Read Science
Must-Reading Tree
Example
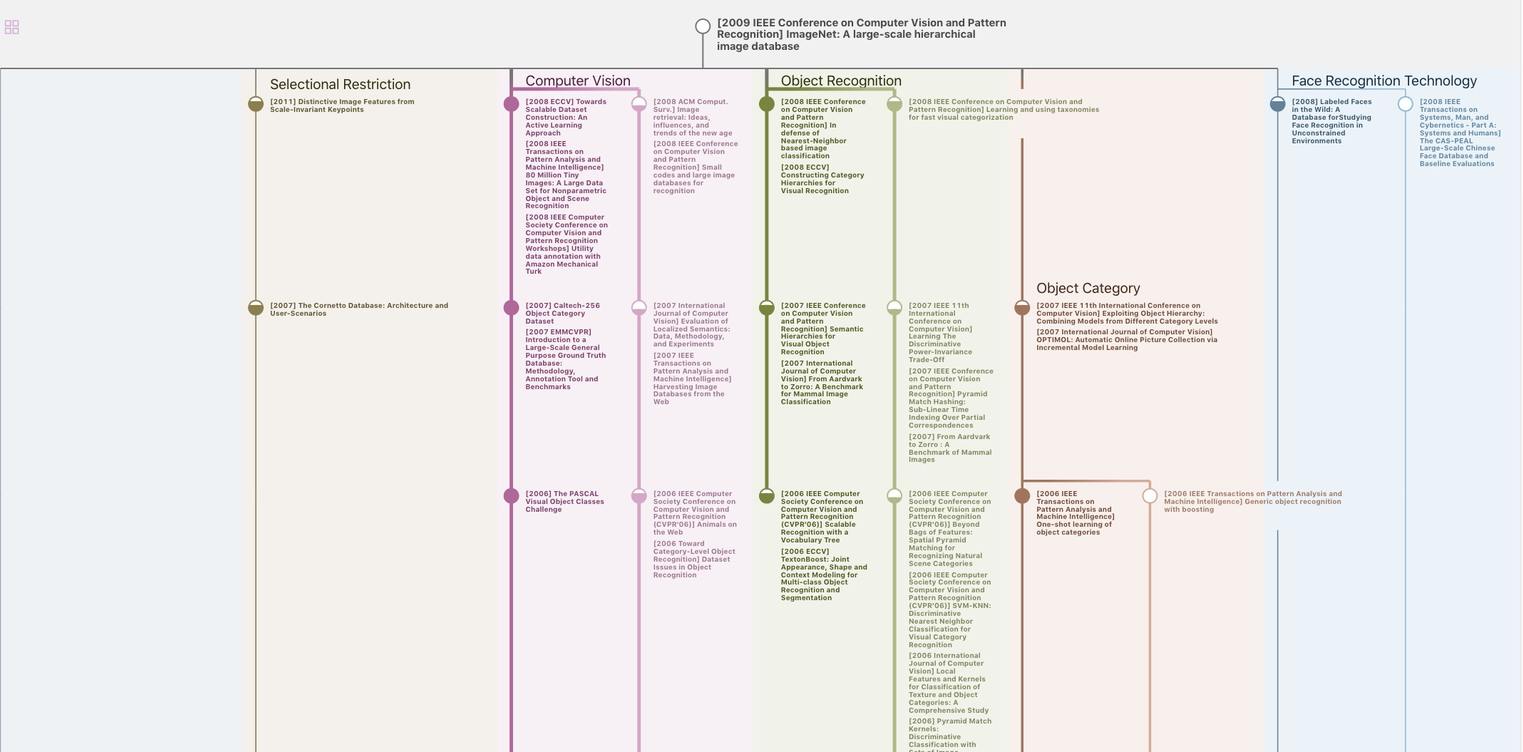
Generate MRT to find the research sequence of this paper
Chat Paper
Summary is being generated by the instructions you defined