Lung tumor discrimination by deep neural network model CanDo via DNA methylation in bronchial lavage
iScience(2024)
摘要
Bronchoscopic-assisted discrimination of lung tumors presents challenges, especially in cases with contraindications or inaccessible lesions. Through meta-analysis and validation using the HumanMethylation450 database, this study identified methylation markers for molecular discrimination in lung tumors and designed a sequencing panel. DNA samples from 118 bronchial washing fluid (BWF) specimens underwent enrichment via multiplex PCR before targeted methylation sequencing. The Recursive Feature Elimination Cross-Validation and deep neural network algorithm established the CanDo classification model, which incorporated 11 methylation features (including 8 specific to the TBR1 gene), demonstrating a sensitivity of 98.6% and specificity of 97.8%. In contrast, bronchoscopic rapid on-site evaluation (bronchoscopic-ROSE) had lower sensitivity (87.7%) and specificity (80%). Further validation in 33 individuals confirmed CanDo's discriminatory potential, particularly in challenging cases for bronchoscopic-ROSE due to pathological complexity. CanDo serves as a valuable complement to bronchoscopy for the discriminatory diagnosis and stratified management of lung tumors utilizing BWF specimens.
更多查看译文
关键词
Lung cancer,DNA Methylation,Bronchial washing fluid,Auxiliary Diagnosis,TBR1
AI 理解论文
溯源树
样例
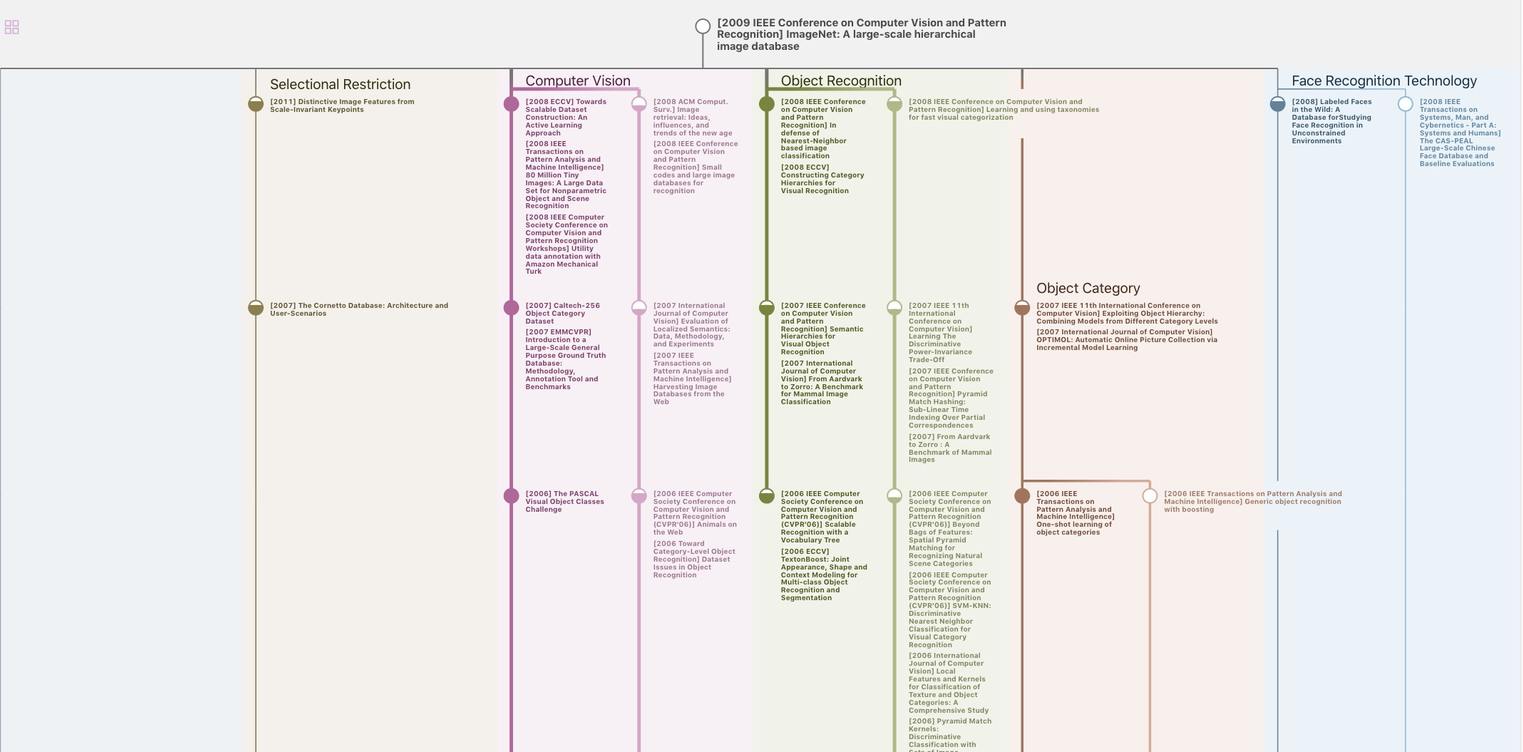
生成溯源树,研究论文发展脉络
Chat Paper
正在生成论文摘要