Estimation of shale adsorption gas content based on machine learning algorithms
GAS SCIENCE AND ENGINEERING(2024)
Abstract
Shale gas is a clean and low -carbon natural gas resource. It mainly exists in both adsorbed and free states in pores and fractures. To accurately estimate the in -situ adsorption gas content (AGC), which is helpful in resource evaluation and development planning, methane isothermal adsorption data and geological parameters have been collected, such as total organic carbon (TOC) content, thermal maturity (R o ), siliceous mineral content (V QF ), total clay content (V TC ), water content (V WC ), and temperature (T). Using machine learning (ML) methods, the in -situ AGC estimation models were constructed and optimized. Various geological factors affecting methane adsorption were evaluated, and an application was conducted in the Wufeng-Formation shale. The results reveal that the four ML models have higher accuracy in predicting Langmuir volume (V L ) and Langmuir pressure (P L ) than empirical formulas and linear regression models. Among the four ML models, the Random Forest Regression (RFR) and eXtreme Gradient Boosting Regression (XGBR) models perform the best, with R 2 higher than 0.85. TOC and T are the main factors affecting methane adsorption, followed by R o and V QF , while the importance of V TC and V WC is relatively low. According to different combinations of geological parameters, there are three schemes for ML model construction. Among them, scheme 1 based on all six geological parameters has the highest accuracy and is most beneficial to predicting AGC. Gradually reducing V WC , V TC , and V QF results in a slight decrease in accuracy, with R 2 decreasing by at most about 6%, scheme 2 is suitable for rougher estimation of AGC. Further removal of T and R o results in a significant decrease in accuracy, with R 2 decreasing by up to 50% and MRE exceeding 30%, rendering scheme 3 unavailable for AGC prediction. The AGC of WufengLongmaxi shale is successfully predicted based on XGBR model, with AGC mainly in 1.0 m 3 /ton-4.0 m 3 /ton. Overall, the ML models based on multiple geological parameters can simulate the real reservoir environment and achieve rapid and accurate estimation of in -situ AGC.
MoreTranslated text
Key words
Shale gas,Machine learning,Adsorption gas content,Multiple geological parameters
AI Read Science
Must-Reading Tree
Example
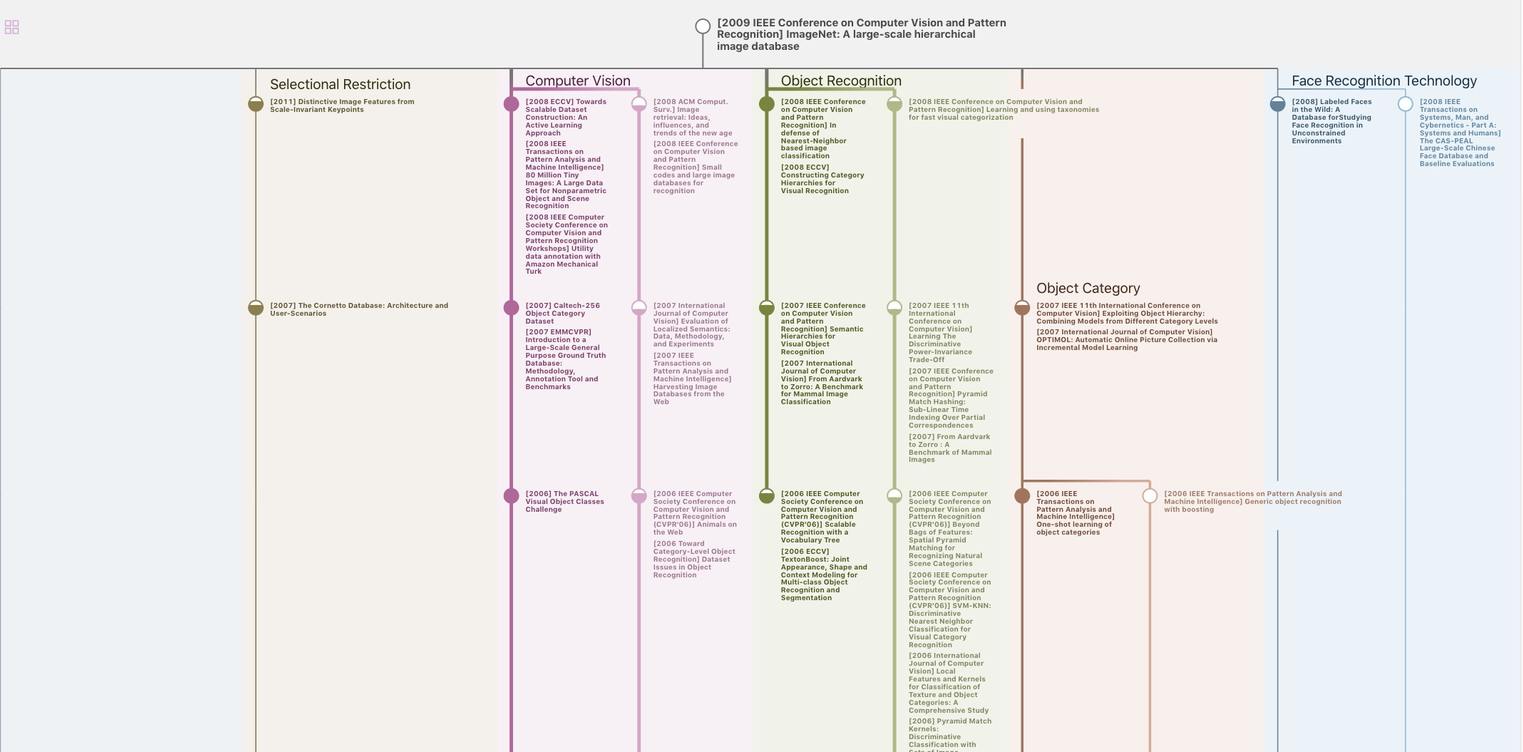
Generate MRT to find the research sequence of this paper
Chat Paper
Summary is being generated by the instructions you defined