Advancing near-infrared spectroscopy: A synergistic approach through Bayesian optimization and model stacking
Spectrochimica Acta Part A: Molecular and Biomolecular Spectroscopy(2024)
摘要
Fourier transform near-infrared (FT-NIR) spectroscopy is a versatile and non-destructive analytical tool widely utilized in industries such as food, pharmaceuticals, and agriculture. While traditional FT-NIR instruments pose limitations in terms of cost and complexity, the advent of portable and affordable systems like NeoSpectra Scanners has broadened accessibility. Partial Least Squares Regression (PLSR) stands as an industry-standard method in Chemometrics for analyzing chemical compositions. This work addresses optimizing PLSR models in FT-NIR spectroscopy, focusing on enhancing accuracy and adaptability in material analysis. Unlike traditional PLSR models which often rely on grid searching a limited number of parameters, such as latent variables, the presented approach effectively expands the parameter space. A novel framework combining Bayesian search and stacking techniques is introduced to enable more customization while ensuring time and performance efficiency, along with automation in model development. Bayesian search efficiently explores hyperparameters space, enabling faster convergence to optimal model settings without exhaustive exploration. The proposed stacked model leverages learned knowledge from the top-performing PLSR models optimized through Bayesian methods, amalgamating a unified and potent body of knowledge. Bayesian-stacked models are compared with PLSR models that use grid search for a limited parameter set. Findings show a marked improvement in model performance: a 51.5% reduction in Root Mean Square Error (RMSE) for the training dataset and a 26.1% reduction for the testing dataset, alongside a 10.9% increase in the correlation coefficient square (R2) for the training dataset and a 10.4% increase for the testing dataset. Notably, Bayesian search reduces the model optimization time by approximately 90% compared with the grid search. Furthermore, when addressing instrumental variations, the models demonstrate an additional improvement, evident in the average reduction of 24.1% in the mean range of prediction. Overall, results demonstrate that the presented approach not only increases the prediction accuracy but also offers a more efficient, automated and robust solution for diverse spectroscopic applications.
更多查看译文
关键词
Near-Infrared Spectroscopy (NIRS),Chemometrics,Partial Least Squares Regression (PLSR),Bayesian Optimization,Model Stacking,Meta Learner
AI 理解论文
溯源树
样例
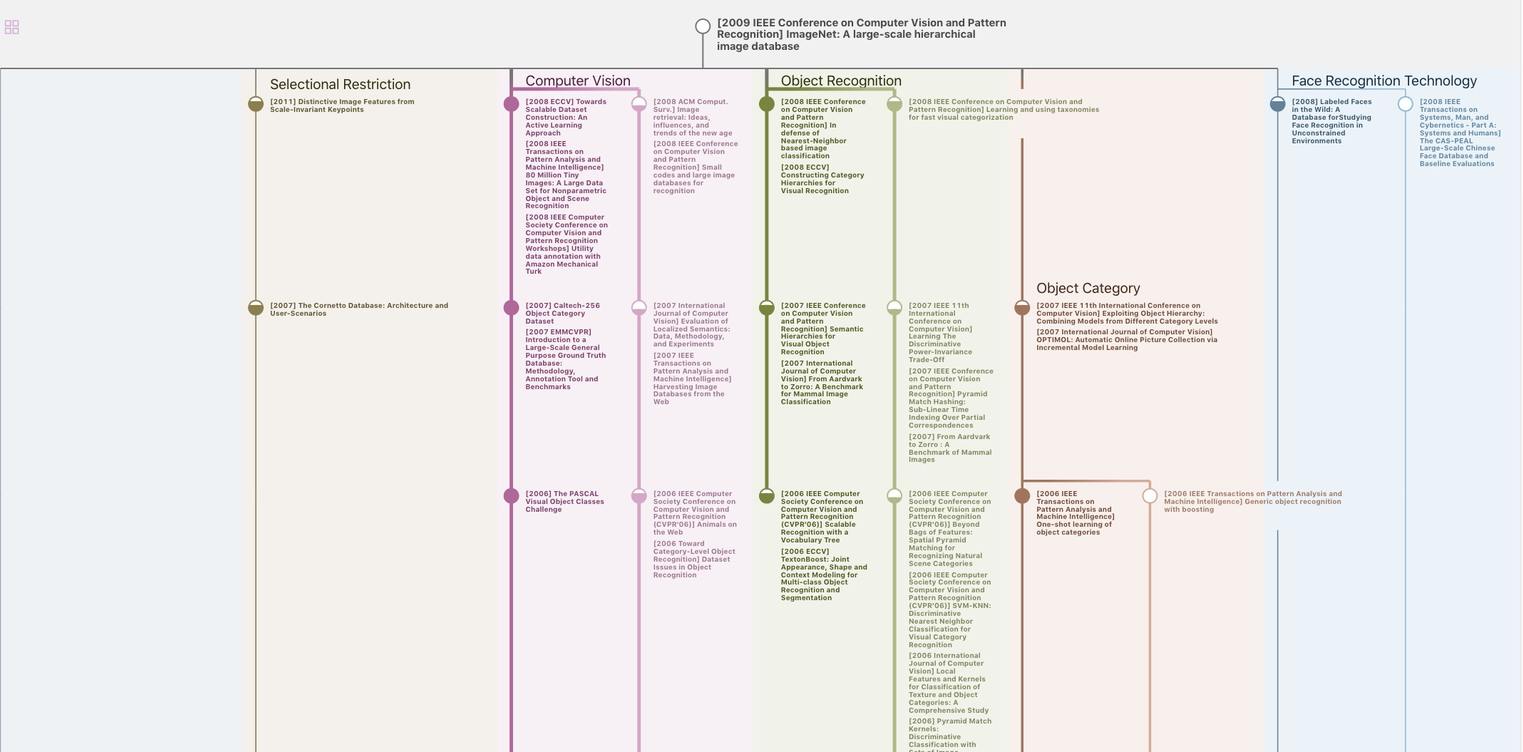
生成溯源树,研究论文发展脉络
Chat Paper
正在生成论文摘要