SensorNet An Adaptive Attention Convolutional Neural Network for Sensor Feature Learning
SENSORS(2024)
Abstract
This work develops a generalizable neural network, SENSORNET, for sensor feature learning across various applications. The primary challenge addressed is the poor portability of pretrained neural networks to new applications with limited sensor data. To solve this challenge, we design SensorNet, which integrates the flexibility of self-attention with multi-scale feature locality of convolution. Moreover, we invent patch-wise self-attention with stacked multi-heads to enrich the sensor feature representation. SensorNet is generalizable to pervasive applications with any number of sensor inputs, and is much smaller than the state-of-the-art self-attention and convolution hybrid baseline (0.83 M vs. 3.87 M parameters) with similar performance. The experimental results show that SensorNet is able to achieve state-of-the-art performance compared with the top five models on a competition activity recognition dataset (SHL'18). Moreover, pretrained SensorNet in a large inertial measurement unit (IMU) dataset can be fine-tuned to achieve the best accuracy on a much smaller IMU dataset (up to 5% improvement in WISDM) and to achieve the state-of-the-art performance on an EEG dataset (SLEEP-EDF-20), showing the strong generalizability of our approach.
MoreTranslated text
Key words
sensor feature learning,human behavior recognition,attention convolutional neural network
AI Read Science
Must-Reading Tree
Example
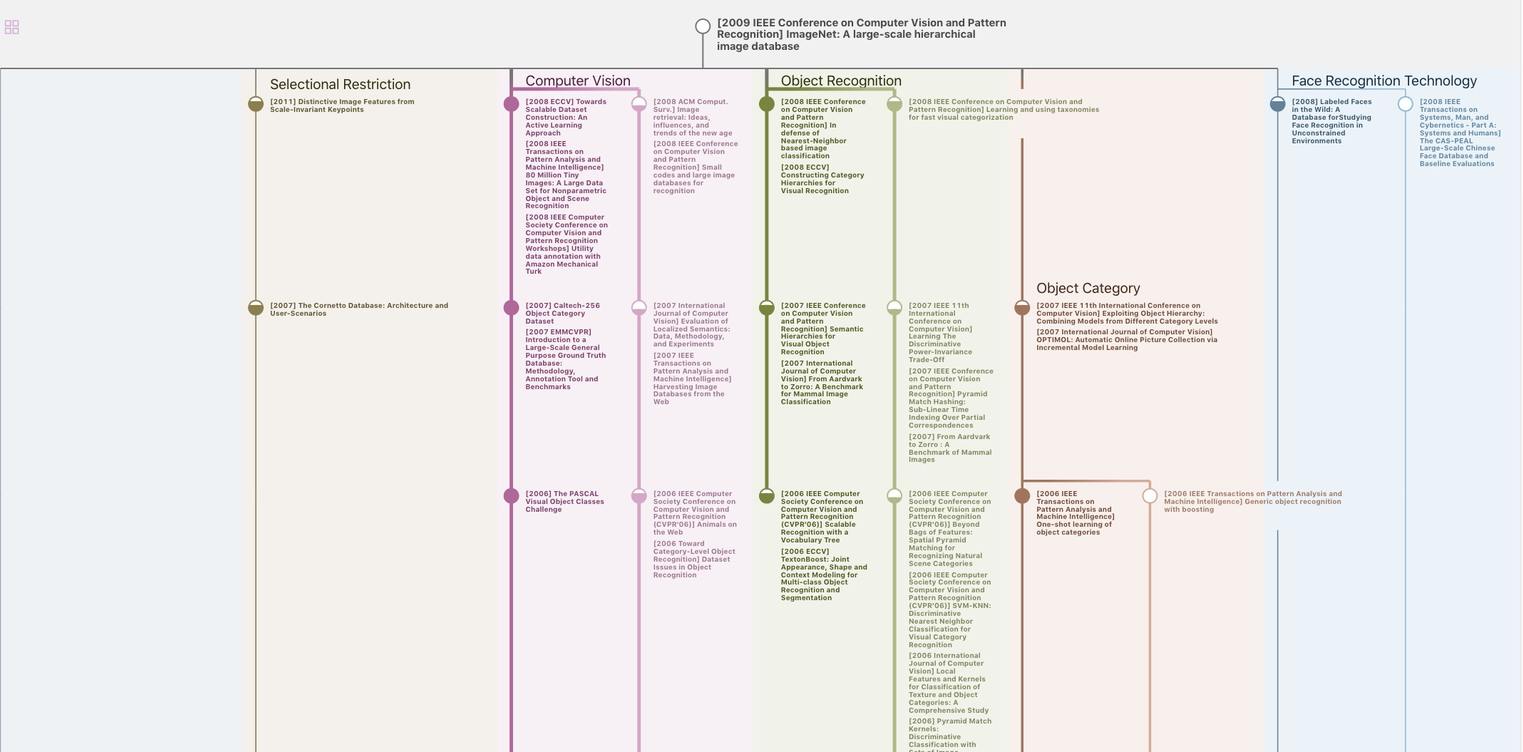
Generate MRT to find the research sequence of this paper
Chat Paper
Summary is being generated by the instructions you defined