Combining Spatial Downscaling Techniques and Diurnal Temperature Cycle Modelling to Estimate Diurnal Patterns of Land Surface Temperature at Field Scale
PFG – Journal of Photogrammetry, Remote Sensing and Geoinformation Science(2024)
摘要
Land surface Temperature (LST) at high spatial resolution and at sub-daily scale is highly useful for monitoring evaporative stress in plants, heatwave events, and droughts. Spatial downscaling methods are often used to improve the spatial resolution of LST and Diurnal Temperature Cycle (DTC) models are available to estimate the diurnal variation in LST using limited multi-temporal satellite observations. In this paper, we propose a simple approach to estimate DTC at field scale combining spatial downscaling and DTC modelling. For downscaling the LST from medium-resolution sensors, we have compared three spatial downscaling techniques: Principal Component Regression based disaggregation, DisTrad disaggregation model and a Spatio Temporal Integrated Temperature Fusion Model (STITFM). The PCR-based disaggregation technique uses multiple fine-resolution auxiliary datasets such as vegetation indices, radar backscattering coefficient, etc. The downscaled LSTs from PCR and DisTrad were compared with the original fine-resolution LST from ECOSTRESS and Landsat. The spatially downscaled LST observations from all the three models were then used in the GOT01‑ts DTC model to estimate the corresponding diurnal temperature cycle at fine resolution. The DTC estimated from the downscaled LSTs from all the three methods were compared with in situ DTC obtained from ground observations over four sites. The PCR technique using multiple indices captured the spatial and diurnal patterns of LST across four different sites, yielding a combined Root Mean Square Error (RMSE) of 2.48 K and 0.95 coefficient of determination (R2). The proposed approach can be potentially used to model the diurnal variability of land surface fluxes over different landscapes with finer spatial resolution.
更多查看译文
关键词
Land surface temperature disaggregation,Diurnal temperature cycle model,Principal Component Regression,DisTrad,Spatiotemporal fusion
AI 理解论文
溯源树
样例
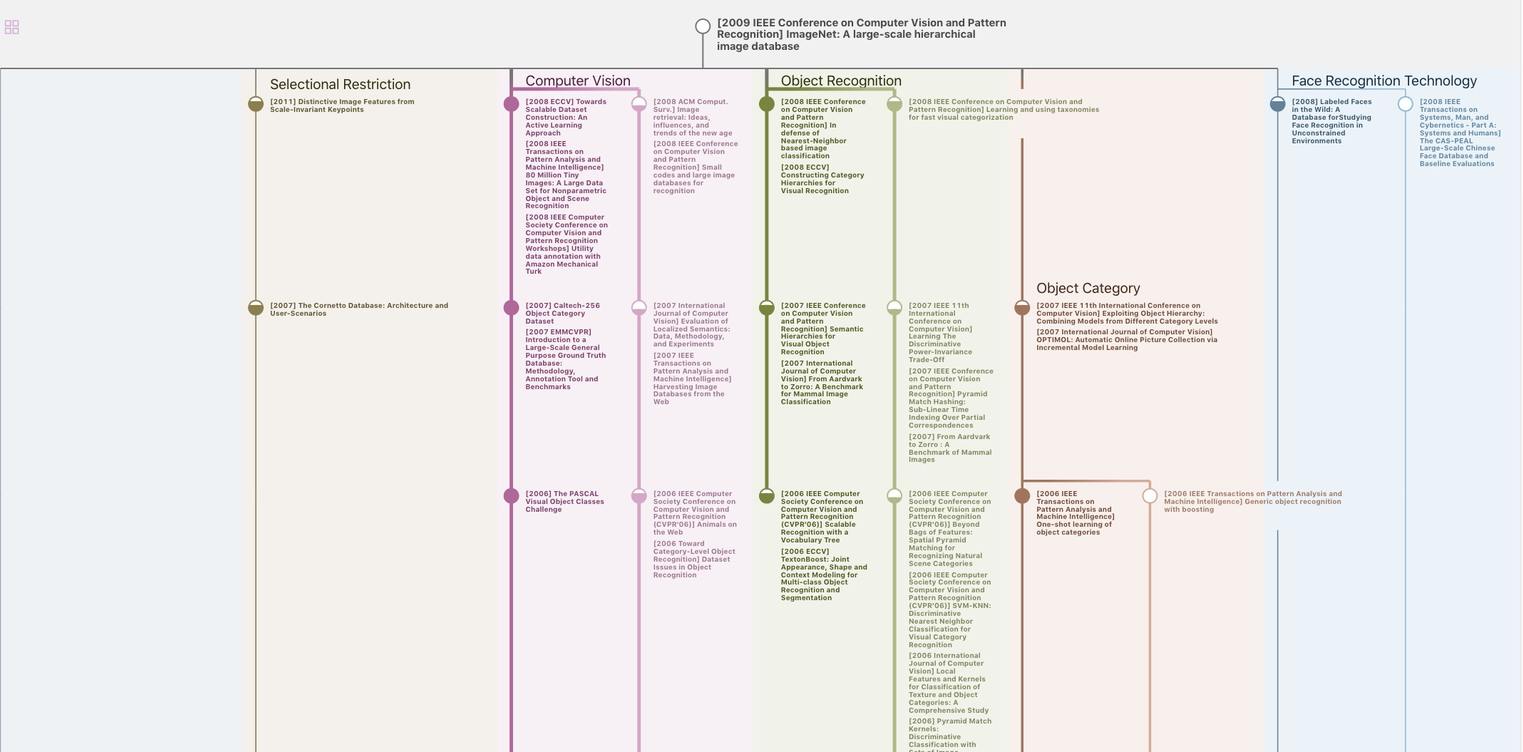
生成溯源树,研究论文发展脉络
Chat Paper
正在生成论文摘要