Machine Learning Techniques for Estimation of Pc5 Geomagnetic Pulsations Observed at Geostationary Orbits During Solar Cycle 23
Journal of Atmospheric and Solar-Terrestrial Physics(2024)
摘要
Pc5 geomagnetic pulsations can accelerate electrons in the radiation belts, which can pose adverse threats to both astronauts and satellites in space. The estimation of Pc5 waves in space is crucial to radiation belt dynamics studies and will help mitigate these challenges. Here, we explore the advantages of the Feed-forward Neural Network (FFNN) and Random Forest (RF) algorithm for effective estimation of Pc5 geomagnetic pulsations observed in space at geostationary orbit during solar cycle 23. The dataset used in this study is the vector magnetic field measurements retrieved from the Geostationary Operational Environmental Satellite-10 (GOES-10) and the solar wind parameters: and component of the solar wind in the Geocentric Solar Ecliptic (GSE) coordinate system, proton density, flow pressure, and plasma beta obtained from the OMNI Web database during part of solar cycle 23. Pc5 geomagnetic pulsations were extracted from the toroidal component of the magnetic field time series using a bandpass Butterworth filter. The continuous wavelet transform (CWT) was utilized to study the characteristics of the extracted wave in the time-frequency domain for its validation. The validated Pc5 events were used as the target in the model's development, with the solar wind parameters as the inputs. In addition to the solar wind parameters, we included an attribute of the magnetic field time series as an input variable in the model. The dataset is carefully divided to ensure effective training and testing of the models. Finally, we trained both models using the same inputs and targets and explored their estimation abilities. The model was tested during the maximum, descending, and minimum phases of solar cycle 23. Both the FFNN and RF models have a similar estimation, with average cross-correlation score (R) values of 0.74 and 0.73 and corresponding average root mean squared error (RMSEs) of 0.16 nT and 0.67 nT, respectively. The model was deployed to investigate the response of Pc5 waves during three storm days in each testing year. The machine learning (ML) model outputs showed good coherence with the observed Pc5 waves. To validate the models, we studied the correlation between the estimated Pc5 events with the Kp index, and a good correlation was seen to exist between both events. This validates the good performance of the developed models. This work will aid in the study of radiation belt dynamics and the construction of electron depletion regions in the radiation belt.
更多查看译文
关键词
Feed-forward neural network,Random forest algorithm,Pc5 geomagnetic pulsations,Ultra-low frequency waves,Machine learning techniques,Solar cycle 23
AI 理解论文
溯源树
样例
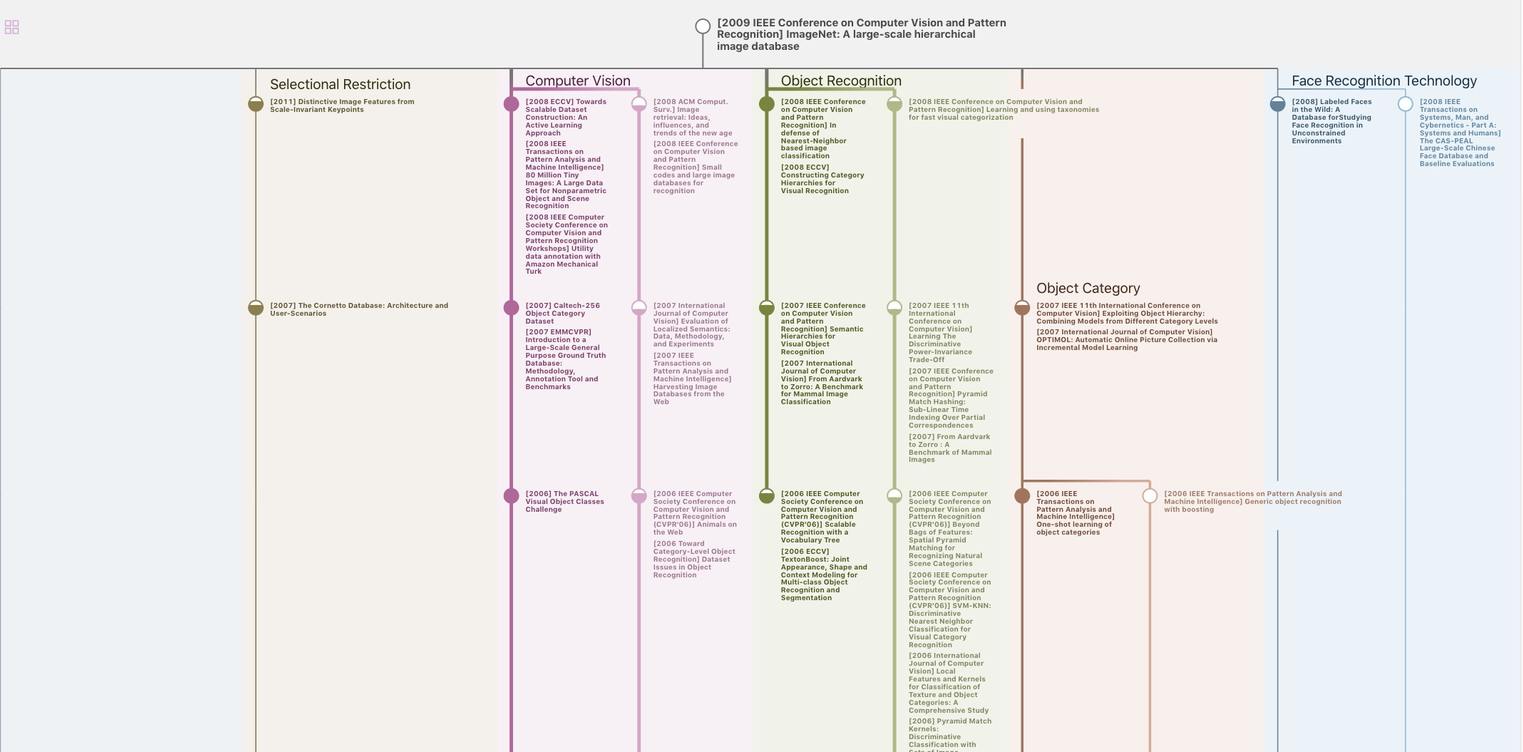
生成溯源树,研究论文发展脉络
Chat Paper
正在生成论文摘要