GNSS NLOS detection method based on stacking ensemble learning and applications in smartphones
GPS Solutions(2024)
摘要
Global Satellite Navigation System (GNSS) has been widely used in various high-precision positioning services and has become an indispensable part of daily production and life. However, in urban environments, GNSS is highly susceptible to interference from non-line-of-sight (NLOS) signals, resulting in a sharp deterioration in positioning accuracy. The use of machine learning methods for NLOS detection has become a research hotspot due to its advantages of no need to change hardware devices and high accuracy. However, in existing machine learning NLOS detection work, the machine learning models used to detect NLOS are usually simple versions. In practice, these existing methods using a single model may fall into the local optimal solution. Therefore, we propose a GNSS NLOS detection method based on the stacking ensemble learning (SEL) model, which reduces the bias of each base model and reduces generalization error by fusing different and heterogeneous base models. The experiment used collected multi-scene smartphone GNSS data to conduct a detailed evaluation of the characteristics of GNSS observations, the accuracy of the models for NLOS detection, and the impact of the SEL NLOS detection model on smartphone GNSS positioning performance. The results show that the GNSS NLOS detection method based on the SEL model has higher detection accuracy and generalization ability than base models. Under static conditions, smartphones can achieve more than 90
更多查看译文
关键词
GNSS,Machine learning,Non-Line-of-Sight (NLOS),Smartphones,Stacking ensemble learning
AI 理解论文
溯源树
样例
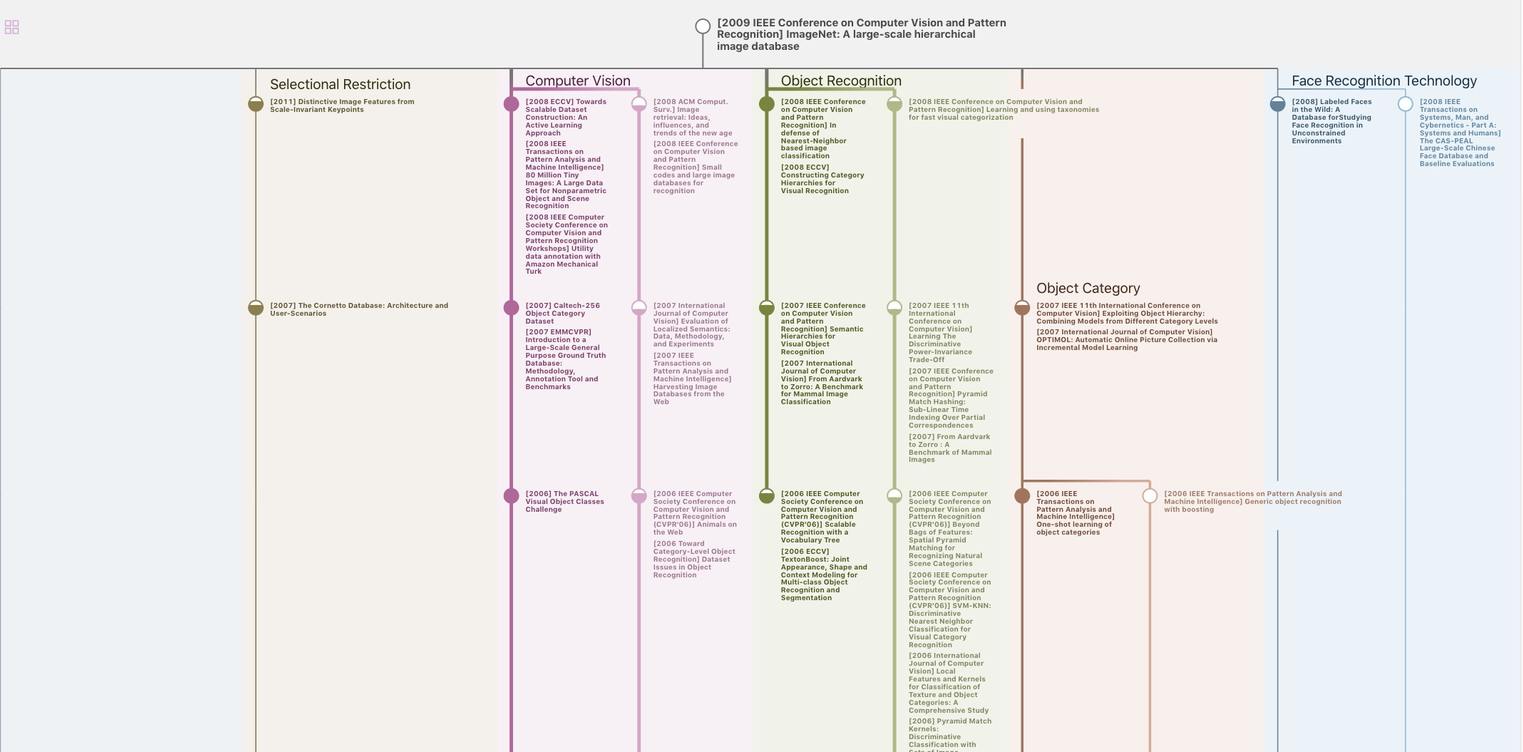
生成溯源树,研究论文发展脉络
Chat Paper
正在生成论文摘要