Novel classification algorithms inspired by firing rate stochastic resonance
crossref(2024)
摘要
Abstract The aim of this paper is to present a category of novel pattern classification algorithms inspired by the phenomenon of the firing rate based stochastic resonance (SR) in a noisy leaky integrate-and-fire neuron. To this end, the firing rate-based SR phenomenon in the noisy leaky integrate-and-fire neuron model is displayed by means of the approximation of adiabatic elimination. And then, a multi-layer neural network with back-propagation learning is constructed by using the stationary firing rare for activation function. Since the intensity of the involving Gaussian white noise is taken as an independent trainable parameter, the benefit of noise can be maximally utilized. The algorithm and its improvements have been verified with binary classification and handwritten digit recognition. By further simplifying calculation of the firing rate activation, this algorithm is embedded into the network architecture of PreAct-ResNet-18 for more complex tasks. It is shown that the improved version based on the stochastic gradient descent (SGD) optimizer outperforms several typical artificial neural network algorithms and brain-inspired spiking neural network (SNN) algorithms on the CIFAR-10 dataset, and it achieves a good accuracy on CIFAR-100, surpassing the accuracy based on the ReLU activation. Since the trained intensity of Gaussian white noise is nonzero in all the applications, stochastic resonance like effect has been observed. Hence it is disclosed from this study that noise can really be designed as an optimizable factor into the brain-inspired machine learning algorithms.
更多查看译文
AI 理解论文
溯源树
样例
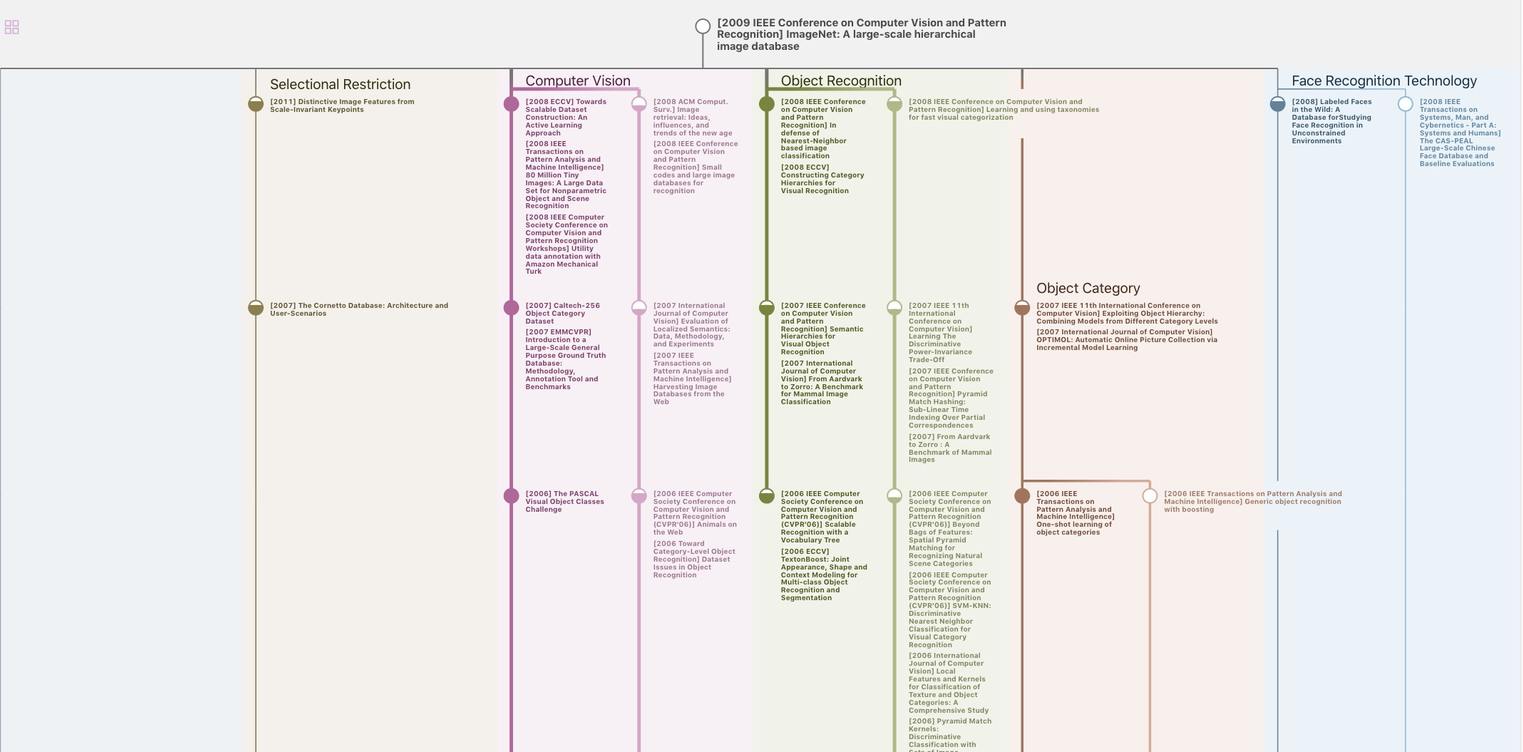
生成溯源树,研究论文发展脉络
Chat Paper
正在生成论文摘要