CTFNet: Coarse-to-Fine Segmented Lane Line Detection in Complex Road Conditions
TRANSPORTATION RESEARCH RECORD(2024)
Abstract
To maintain robustness in complex and uncontrollable real-world driving scenarios, this paper proposes a new segmentation-based coarse-to-fine lane line model (CTFNet). Which embeds dual-pathway attention (DPA) in the coarse segmentation encoder-decoder architecture to fuse high and low-level features with dual inputs, taking the strengths and complementing the weaknesses, and at the same time being able to capture more spatial detail information. However, the extracted lane line cues are limited in extreme conditions. As a result, a feature localization module (FLM) is proposed which extracts the global contextual information of the occluded region along the vertical and horizontal axes and determines lane line location by predicting the confidence of the lane lines based on the extracted information. Additionally, some regions of the initial feature map of the coarse segmentation network are difficult to distinguish between classes, the uncertain region refinement module (URRM) is designed in the fine stage to gradually refine the uncertain pixels using the relationship between adjacent features. Finally, the model is extensively tested on the tvtLANE data set, and the results show that CTFNet outperforms most state-of-the-art methods with an F1-measure of 91.48%, which not only reduces false detection but also maintains good robustness in extremely difficult scenarios.
MoreTranslated text
Key words
data and data science,artificial intelligence and advanced computing applications,artificial intelligence,deep learning,machine learning (artificial intelligence),neural networks
AI Read Science
Must-Reading Tree
Example
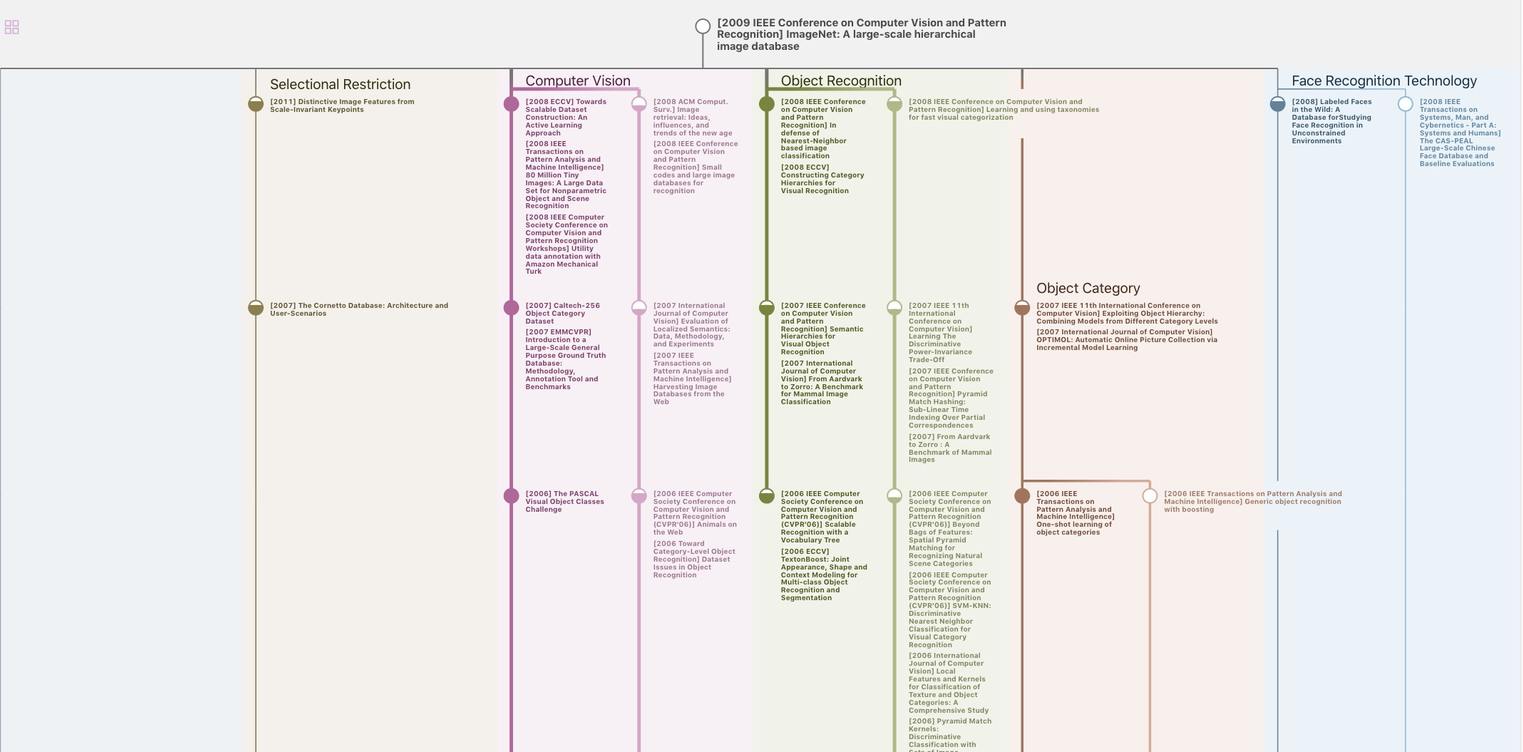
Generate MRT to find the research sequence of this paper
Chat Paper
Summary is being generated by the instructions you defined