Accelerating Genome- and Phenome-Wide Association Studies using GPUs - A case study using data from the Million Veteran Program.
bioRxiv : the preprint server for biology(2024)
摘要
The expansion of biobanks has significantly propelled genomic discoveries yet the sheer scale of data within these repositories poses formidable computational hurdles, particularly in handling extensive matrix operations required by prevailing statistical frameworks. In this work, we introduce computational optimizations to the SAIGE (Scalable and Accurate Implementation of Generalized Mixed Model) algorithm, notably employing a GPU-based distributed computing approach to tackle these challenges. We applied these optimizations to conduct a large-scale genome-wide association study (GWAS) across 2,068 phenotypes derived from electronic health records of 635,969 diverse participants from the Veterans Affairs (VA) Million Veteran Program (MVP). Our strategies enabled scaling up the analysis to over 6,000 nodes on the Department of Energy (DOE) Oak Ridge Leadership Computing Facility (OLCF) Summit High-Performance Computer (HPC), resulting in a 20-fold acceleration compared to the baseline model. We also provide a Docker container with our optimizations that was successfully used on multiple cloud infrastructures on UK Biobank and All of Us datasets where we showed significant time and cost benefits over the baseline SAIGE model.
更多查看译文
AI 理解论文
溯源树
样例
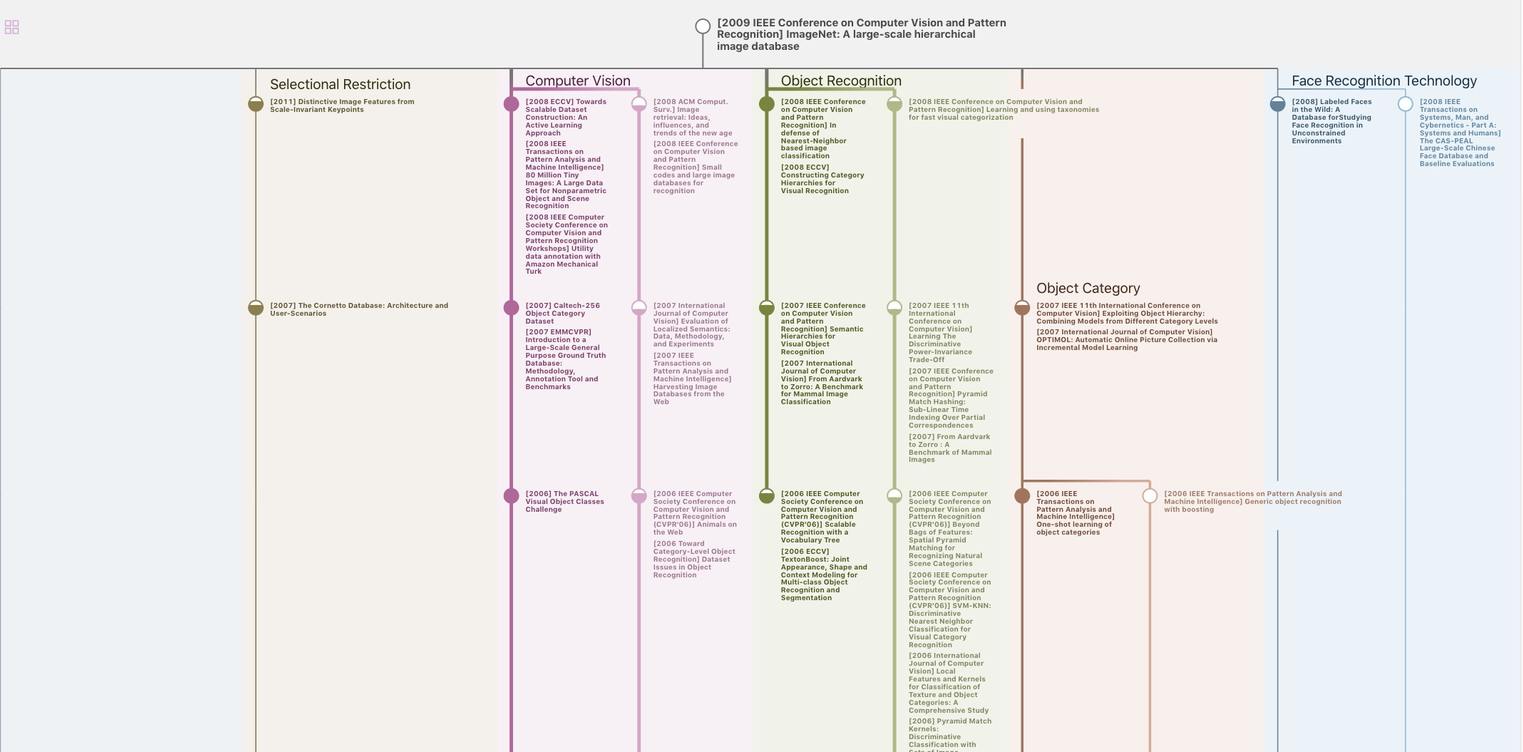
生成溯源树,研究论文发展脉络
Chat Paper
正在生成论文摘要