Inadequate Data Augmentation with Convolutional Neural Network in Brain Electrical Impedance Tomography
IEEE Sensors Journal(2024)
摘要
Electrical impedance tomography (EIT) is a potential technique for imaging intracranial hemorrhage. Generally, 16 electrodes are used in the brain EIT. In some situations, it may be not possible to attach so many electrodes. This reduces the number of measurement and causes poor reconstruction quality. Besides, the skull has poor conductivity which obstructs the injection of current and degrades the quality of the reconstructed image. Thus, inadequate boundary measurement caused by reduced number of electrodes is a great challenge in brain EIT. To address this problem, a novel approach based on convolutional neural network is proposed for inadequate data augmentation when only 8 electrodes can be equipped. The proposed method enables the establishment of a non-linear relationship between the inadequate voltage data and the desired augmented data. The voltage data augmented for the 8-electrode brain EIT is compared with the measured voltage of the brain EIT equipped with 16 electrodes. It is found that two groups of data are very similar. The effectiveness of the proposed approach is further confirmed by imaging hemorrhage in the brain tissue layer. Images reconstructed for various cases are compared with the results which use directly measured voltage. The qualitative evaluation as well as quantitative analysis demonstrates that the proposed approach greatly enhances the reconstruction quality in the case of inadequate measurement. In summary, the voltage data directly measured from the 8-electrode brain EIT can be well augmented with the proposed method.
更多查看译文
关键词
Electrical impedance tomography,convolutional neural network,data augmentation,hemorrhage
AI 理解论文
溯源树
样例
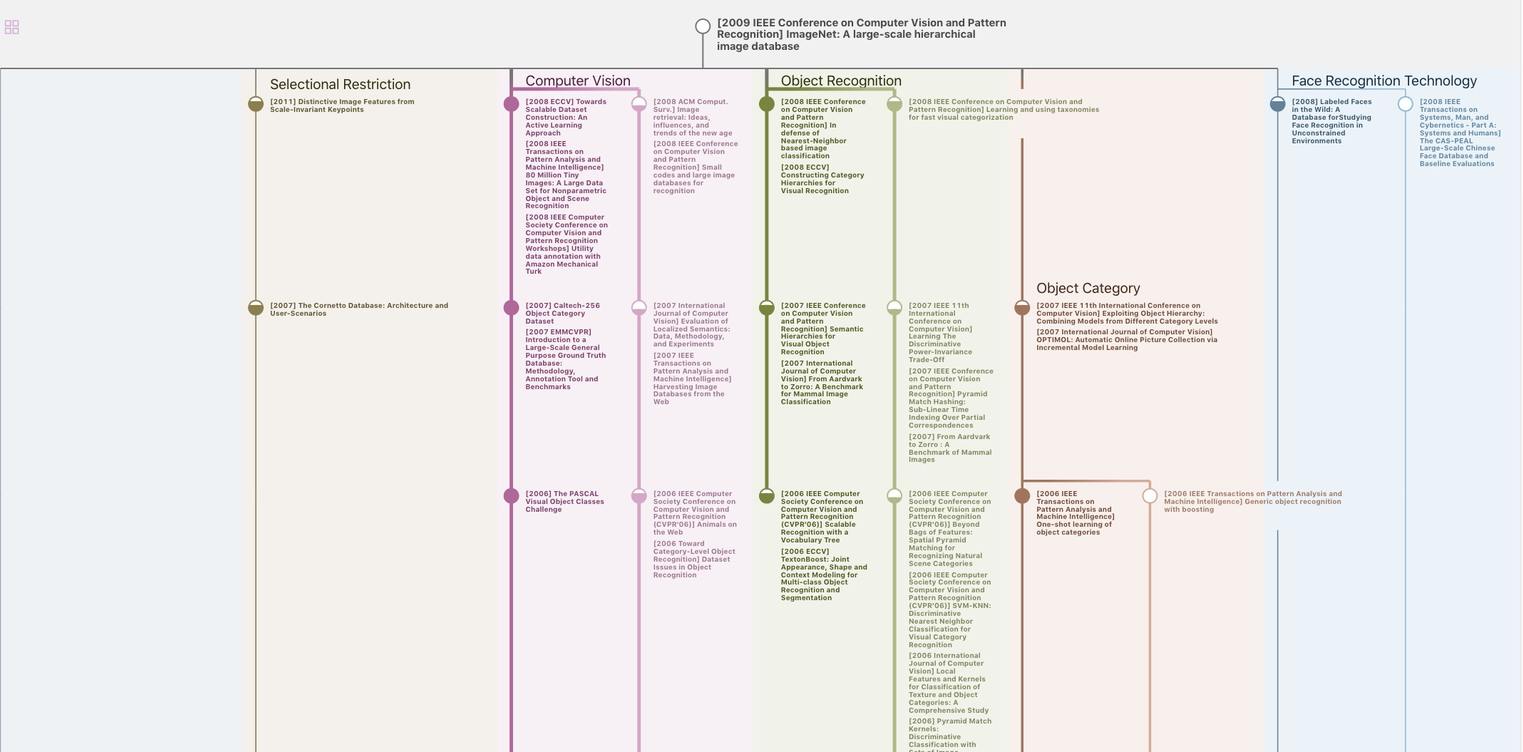
生成溯源树,研究论文发展脉络
Chat Paper
正在生成论文摘要