Generalized nonlinear hybrid-norm parallel sparse filtering for bearing fault diagnosis under complex interference
Measurement Science and Technology(2024)
摘要
Abstract Considerable attention has been garnered by the bearing fault diagnostic method, founded on sparse feature learning, for its exceptional robustness and adaptability to noise. Being an effective sparse learning method, parallel sparse filtering (PSF) has found successful applications in intelligent fault diagnosis. However, influenced by mechanical structural collisions and complex transmission paths, the obtained bearing signals come with abnormal impacts and noise, resulting in a reduced reliability of the PSF. Additionally, PSF constrains features and samples with l_(1⁄2) norm, which is significantly disturbed by noise. To address these issues, generalized nonlinear hybrid-norm parallel sparse filtering (GNHNPSF) is proposed. Firstly, the Sigmoid nonlinear function is examined to suppress the amplitude of the anomalous shock. Subsequently, the hybrid-norm constraints, which involve the application of a l_(1⁄2) norm to the column features of the input signal and a l_(1⁄3) norm to the row features, can be employed to effectively extract distinctive information from the original data. The GNHNPSF is demonstrated to improve the accuracy of bearing fault diagnosis under complex interference, as evidenced by the results of simulation and experiments.
更多查看译文
AI 理解论文
溯源树
样例
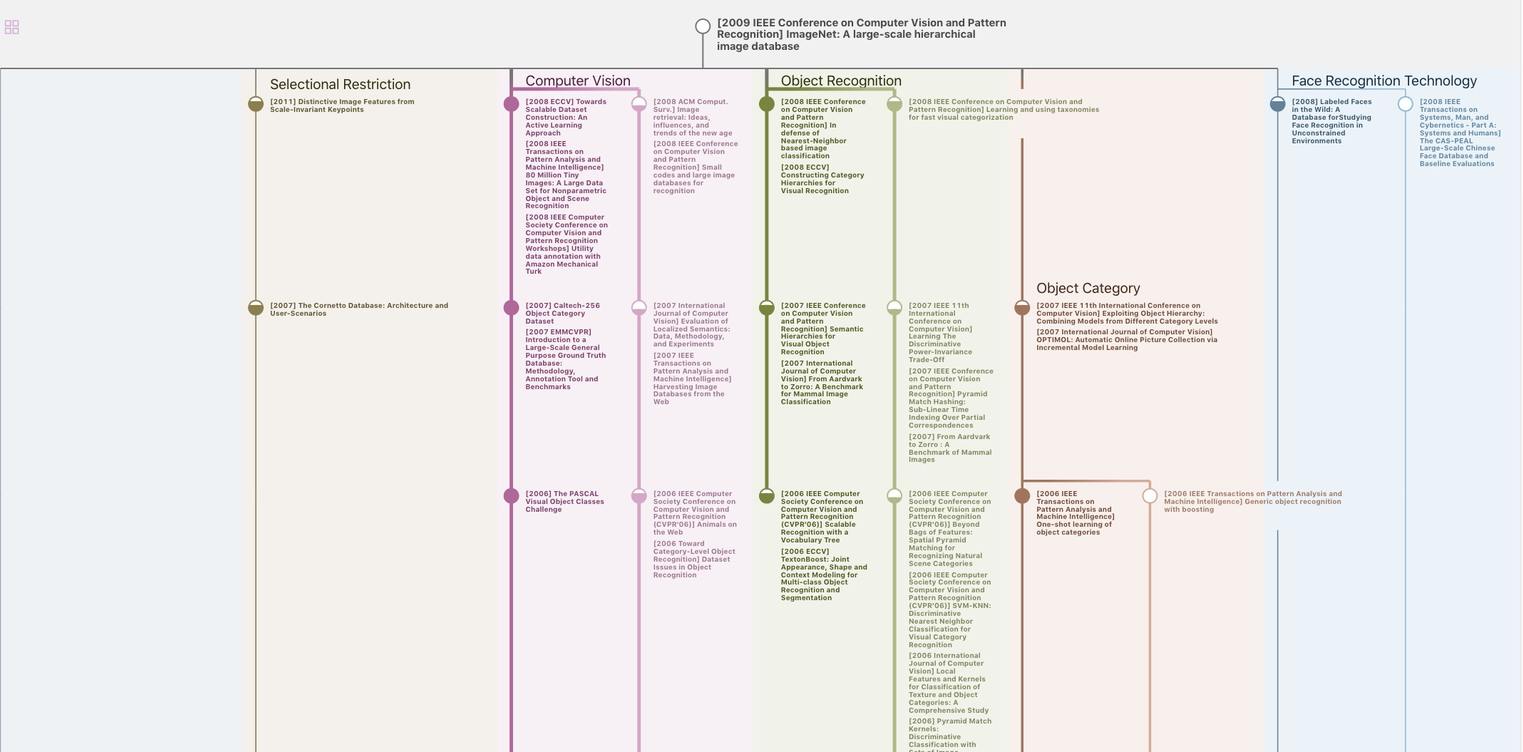
生成溯源树,研究论文发展脉络
Chat Paper
正在生成论文摘要