Deterministic ensemble Kalman filter based on two localization techniques for mitigating sampling errors with a quasi-geostrophic model
Meteorology and Atmospheric Physics(2024)
摘要
In the ensemble Kalman filter (EnKF) framework for data assimilation, a limited ensemble size results in a spurious sampling error and underestimation of the background error covariance. Localization techniques have been explored to mitigate the sampling errors induced by ensemble-based assimilation approaches. In this study, we incorporate a local analysis (LA) scheme and a covariance filter (CL) scheme into the deterministic EnKF (DEnKF), thus formulating a DEnKF method with local analysis (DEnKF(LA)) and a DEnKF method with covariance localization (DEnKF(CL)), respectively. To verify the effectiveness of the proposed algorithm, we perform a series of experiments with a large-scale (quasi-geostrophic) model. The results show that the DEnKF(LA) method yields better assimilation results than the DEnKF(CL), the traditional EnKF with a covariance filter (EnKF(CL)) and the ensemble square-root filter with local analysis (EnSRF(LA)) in terms of various assimilation parameters (i.e., ensemble size, localization, inflation, and observation error variance). In addition, the DEnKF(CL) and DEnKF(LA) methods both yield well-constrained analyses, as determined by examining the spatial state distributions of the true field, the analysis field and the average root-mean-square errors (RMSEs) field for the various methods. Overall, the DEnKF(LA) method clearly outperforms the other data assimilation methods. Hence, the proposed DEnKF(LA) method has good prospects for application in atmospheric and oceanic models.
更多查看译文
AI 理解论文
溯源树
样例
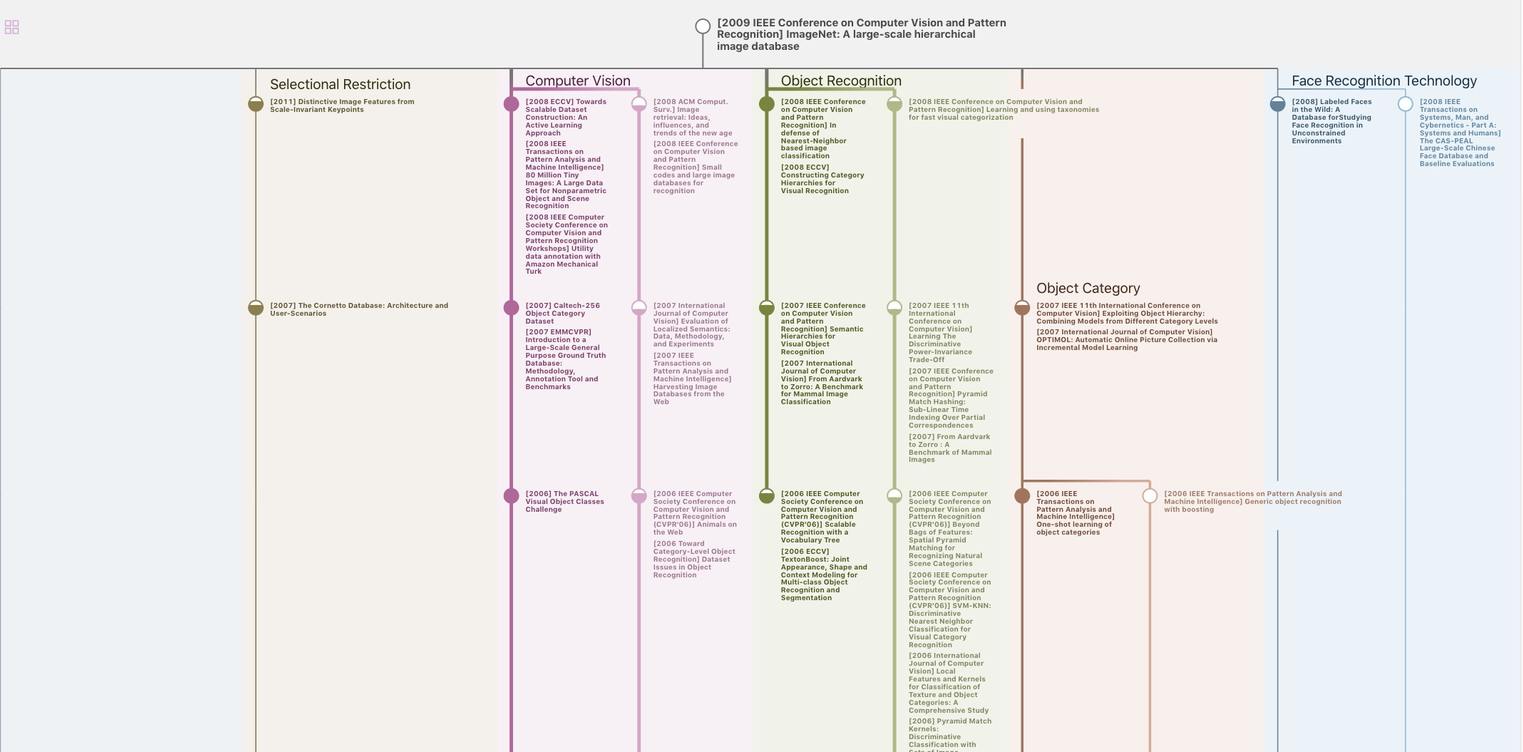
生成溯源树,研究论文发展脉络
Chat Paper
正在生成论文摘要