Spatio-Temporal Feature Engineering for Deep Learning Models in Traffic Flow Forecasting
IEEE access(2024)
Abstract
In the past decade, modern transportation systems have employed various cutting-edge deep-learning approaches for traffic flow prediction. Due to its significant temporal correlations, researchers have mainly focused on extracting temporal features from traffic flow data. As a result, time-series models based on deep learning methods like Gated Recurrent Unit (GRU), Long-Term Short-Term Memory (LSTM), and Temporal Convolutional Networks (TCN) have been introduced as solutions for traffic flow prediction. However, the spatial features of the road network have also shown an impact on the prediction, leading to the application of deep learning methods on spatial dependency modeling for this problem. This paper defines the traffic flow forecasting problem, considering both time-series information with and without spatial information and the corresponding techniques of current solutions to depict spatio-temporal traffic dependency. We propose a new taxonomy of spatial and temporal dependencies in the fine-grained subcategory and the methods depicting them based on neural network-based models. Furthermore, we highlight the architecture of spatial and temporal ensembles in Spatio-temporal modelling based on the fine-grained categories obtained. We point out several open issues and future directions of traffic flow forecasting, such as graph reconstruction, temporal and spatial information data balance, and multi-model spatial and temporal correlations.
MoreTranslated text
Key words
Forecasting,Data models,Long short term memory,Surveys,Predictive models,Feature extraction,Computer architecture,Deep learning,Graph neural networks,Spatiotemporal phenomena,Intelligent transportation systems,Traffic control,graph neural network,spatial-temporal dependence,traffic flow forecasting
AI Read Science
Must-Reading Tree
Example
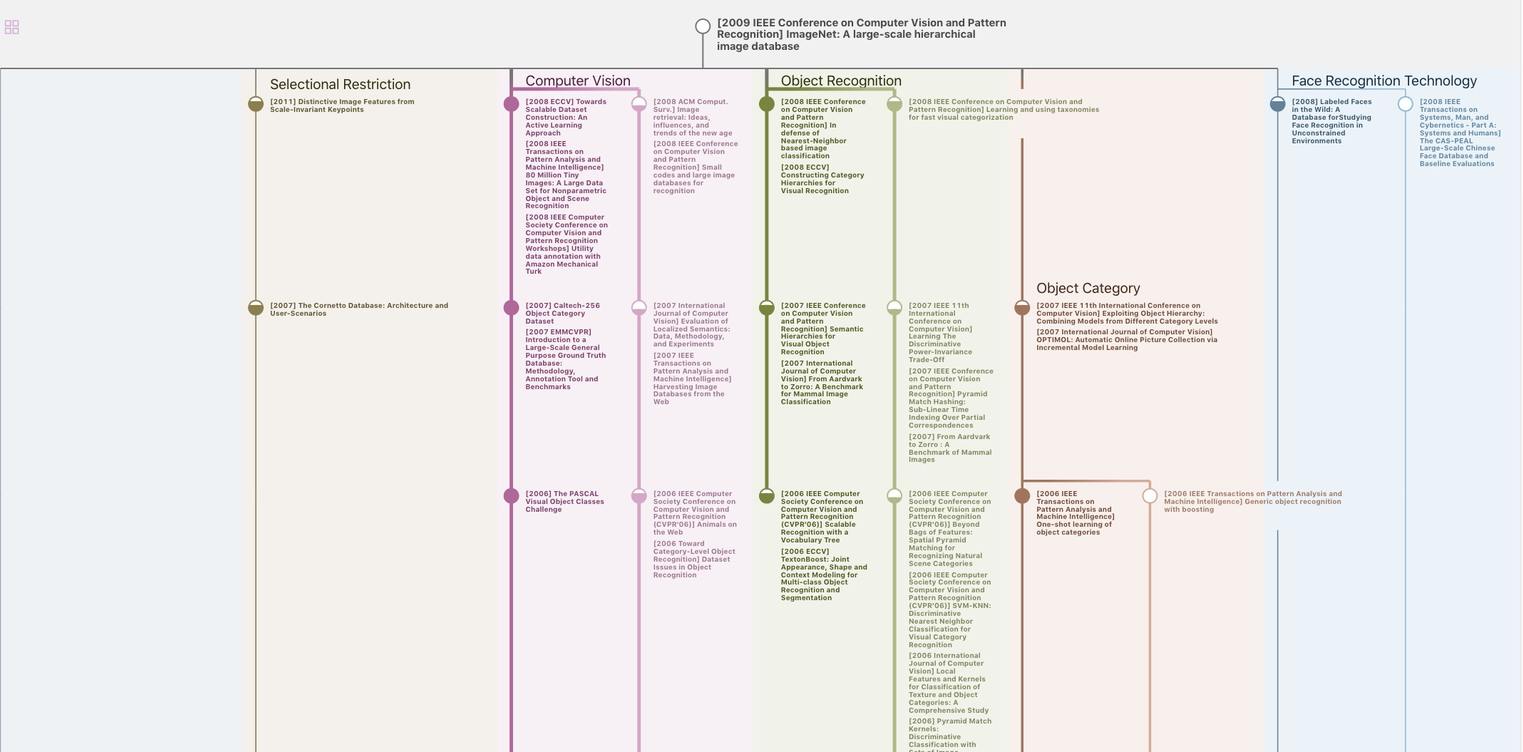
Generate MRT to find the research sequence of this paper
Chat Paper
Summary is being generated by the instructions you defined