Self-supervised dynamic and static feature representation learning method for flotation monitoring
Powder Technology(2024)
Abstract
Froth flotation is a widely used beneficiation method in mineral processing. Accurate grade monitoring is crucial for efficient minerals separation. However, despite recent developments highlighting the potential of deep learning in flotation monitoring, these methods face limitations in application due to the lack of reliable feature extraction and the scarcity of large datasets related to target task. To address these issues, we propose a self-supervised dynamic and static feature collaborative representation learning method. It extracts the spatiotemporal information from froth videos based on a two-stream convolutional architecture and is trained on a video frame prediction task without labeled data until both appearance and motion constraints are satisfied. Experiments on industrial zinc flotation data demonstrate that the learned representations transfer well to downstream grade monitoring tasks. This approach not only solves the label shortage problem when training large-scale deep learning models but also outperforms the state-of-the-art methods in flotation monitoring.
MoreTranslated text
Key words
Feature representation,Deep learning,Self-supervised learning,Video prediction task,Froth flotation monitoring
AI Read Science
Must-Reading Tree
Example
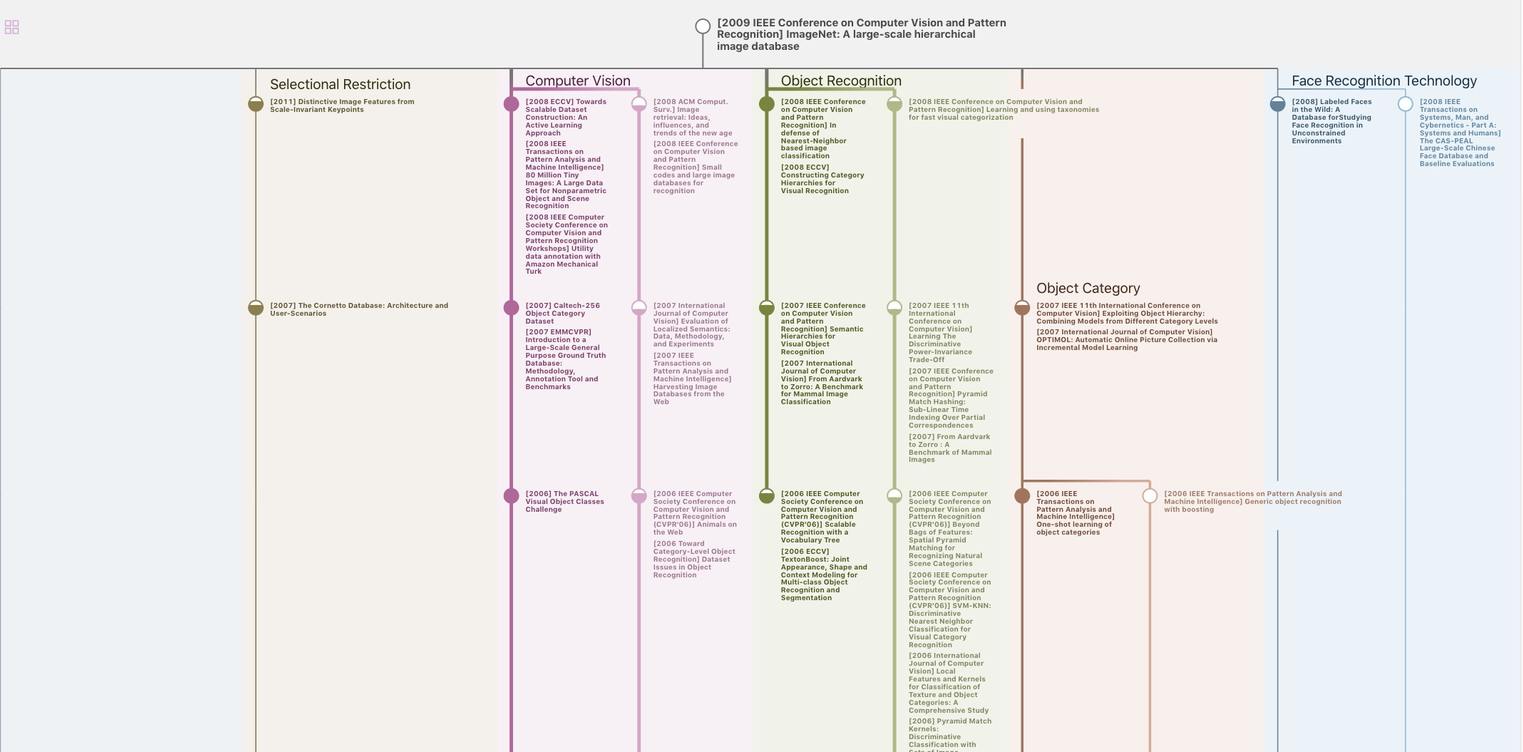
Generate MRT to find the research sequence of this paper
Chat Paper
Summary is being generated by the instructions you defined