Bridging multimodal data and battery science with machine learning
Matter(2024)
摘要
Multimodal data hold paramount significance in the realm of battery science research. Traditional manual tools for data analysis have proven inadequate in meeting the demands of processing and mining multimodal data information. Machine learning emerges as a vital conduit between multimodal data and battery science. This review comprehensively organizes the recent advancements in multimodal data-driven research employing machine learning methodologies within the field of battery research. Specifically, it explores material-data-driven approaches to accelerate the development of advanced battery materials and image-data-driven schemes for cross-scale battery structure analysis and image enhancement, as well as battery assessment driven by condition data using both traditional machine learning and neural-network models. Furthermore, this review delves into the full potential of machine learning in the domain of advanced battery science research, encompassing aspects such as the accumulation of training data, the development of machine learning models, and the application of advanced analysis methods.
更多查看译文
关键词
multimodal data,battery,machine learning,data-driven,intelligent analysis
AI 理解论文
溯源树
样例
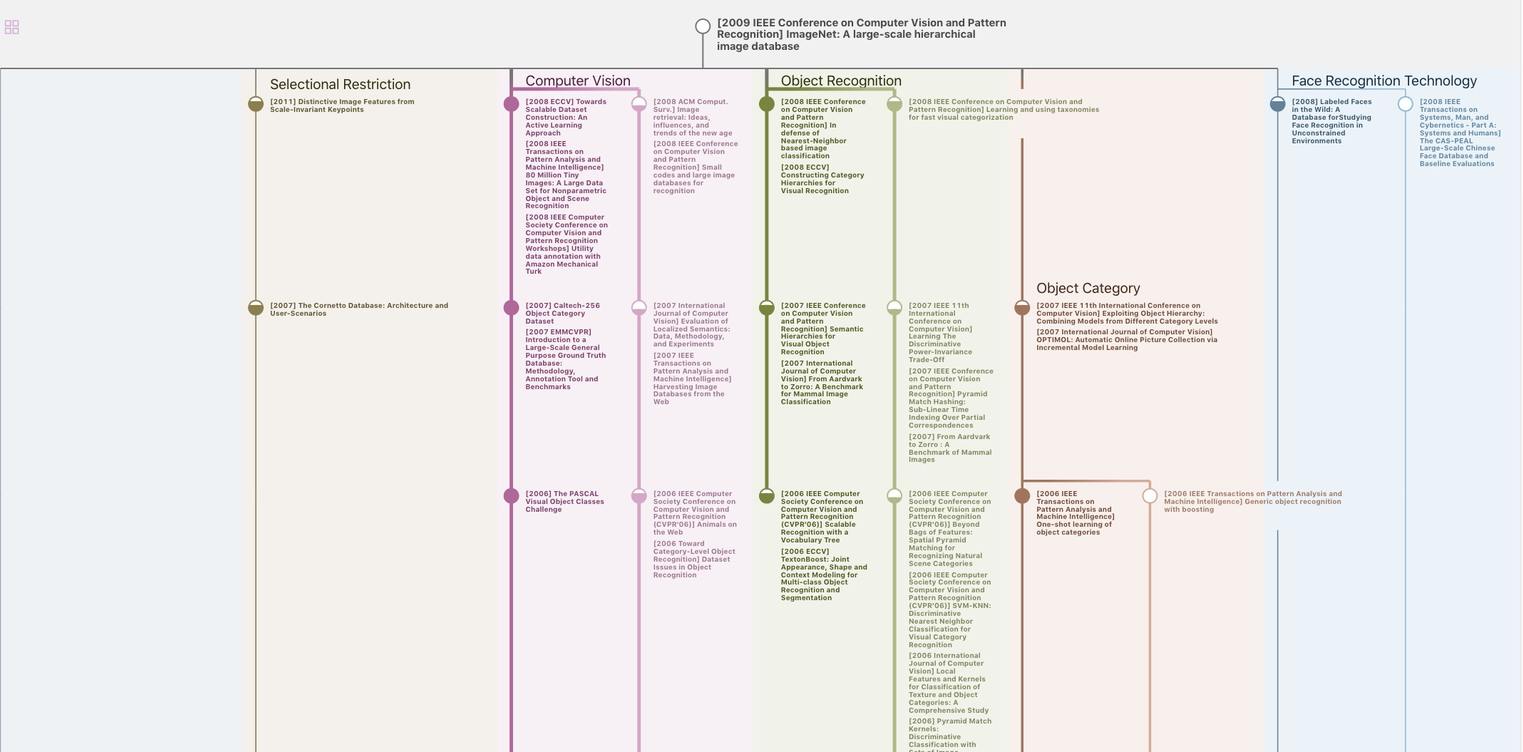
生成溯源树,研究论文发展脉络
Chat Paper
正在生成论文摘要