Towards proactive palliative care in oncology: developing an explainable EHR-based machine learning model for mortality risk prediction
BMC Palliative Care(2024)
摘要
Ex-ante identification of the last year in life facilitates a proactive palliative approach. Machine learning models trained on electronic health records (EHR) demonstrate promising performance in cancer prognostication. However, gaps in literature include incomplete reporting of model performance, inadequate alignment of model formulation with implementation use-case, and insufficient explainability hindering trust and adoption in clinical settings. Hence, we aim to develop an explainable machine learning EHR-based model that prompts palliative care processes by predicting for 365-day mortality risk among patients with advanced cancer within an outpatient setting. Our cohort consisted of 5,926 adults diagnosed with Stage 3 or 4 solid organ cancer between July 1, 2017, and June 30, 2020 and receiving ambulatory cancer care within a tertiary center. The classification problem was modelled using Extreme Gradient Boosting (XGBoost) and aligned to our envisioned use-case: “Given a prediction point that corresponds to an outpatient cancer encounter, predict for mortality within 365-days from prediction point, using EHR data up to 365-days prior.” The model was trained with 75
更多查看译文
关键词
Oncology,Palliative Medicine,Electronic Health Records,Machine learning,Clinical decision support systems
AI 理解论文
溯源树
样例
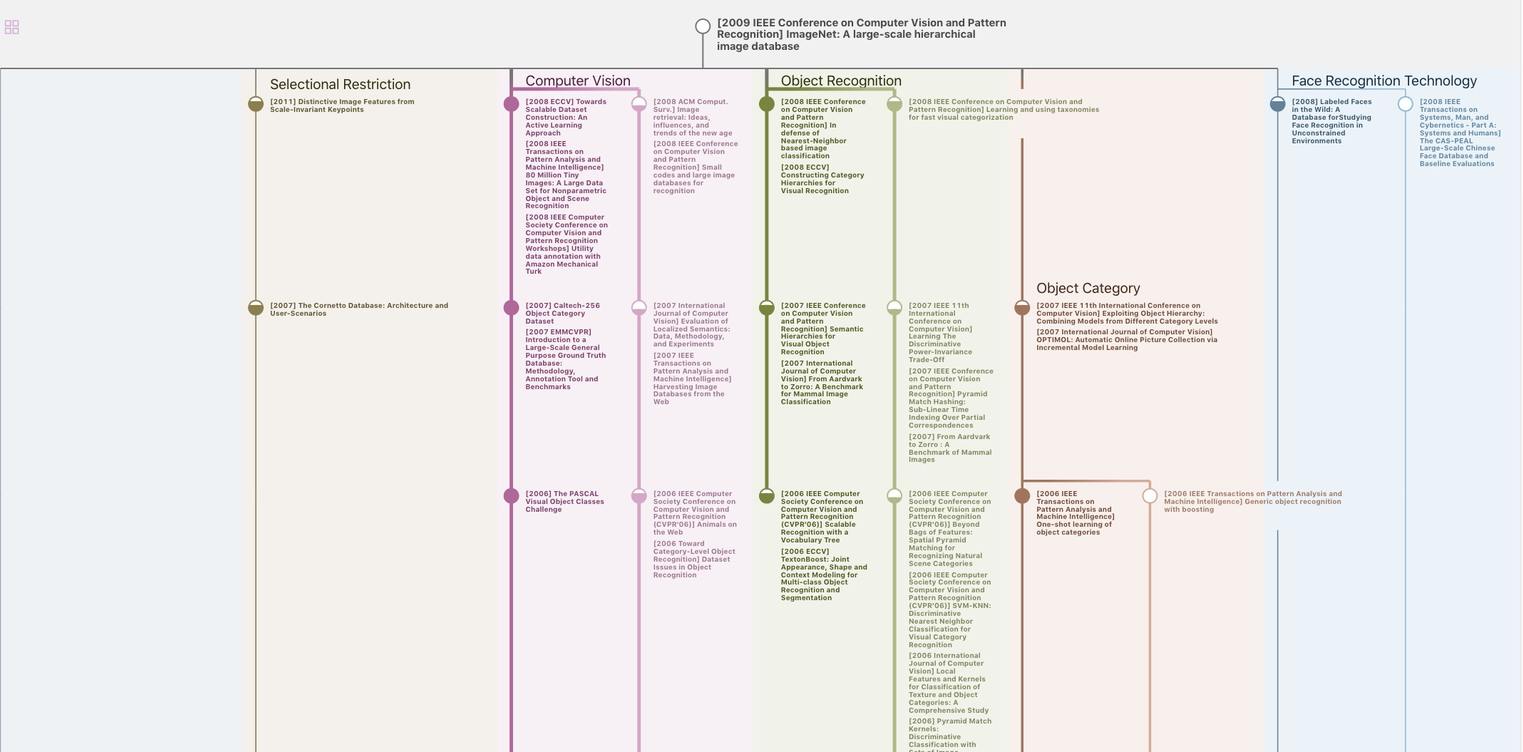
生成溯源树,研究论文发展脉络
Chat Paper
正在生成论文摘要