NPLMV-PS: Neural Point-Light Multi-View Photometric Stereo
CoRR(2024)
Abstract
In this work we present a novel multi-view photometric stereo (PS) method.
Like many works in 3D reconstruction we are leveraging neural shape
representations and learnt renderers. However, our work differs from the
state-of-the-art multi-view PS methods such as PS-NeRF or SuperNormal we
explicity leverage per-pixel intensity renderings rather than relying mainly on
estimated normals.
We model point light attenuation and explicitly raytrace cast shadows in
order to best approximate each points incoming radiance. This is used as input
to a fully neural material renderer that uses minimal prior assumptions and it
is jointly optimised with the surface. Finally, estimated normal and
segmentation maps can also incorporated in order to maximise the surface
accuracy.
Our method is among the first to outperform the classical approach of
DiLiGenT-MV and achieves average 0.2mm Chamfer distance for objects imaged at
approx 1.5m distance away with approximate 400x400 resolution. Moreover, we
show robustness to poor normals in low light count scenario, achieving 0.27mm
Chamfer distance when pixel rendering is used instead of estimated normals.
MoreTranslated text
AI Read Science
Must-Reading Tree
Example
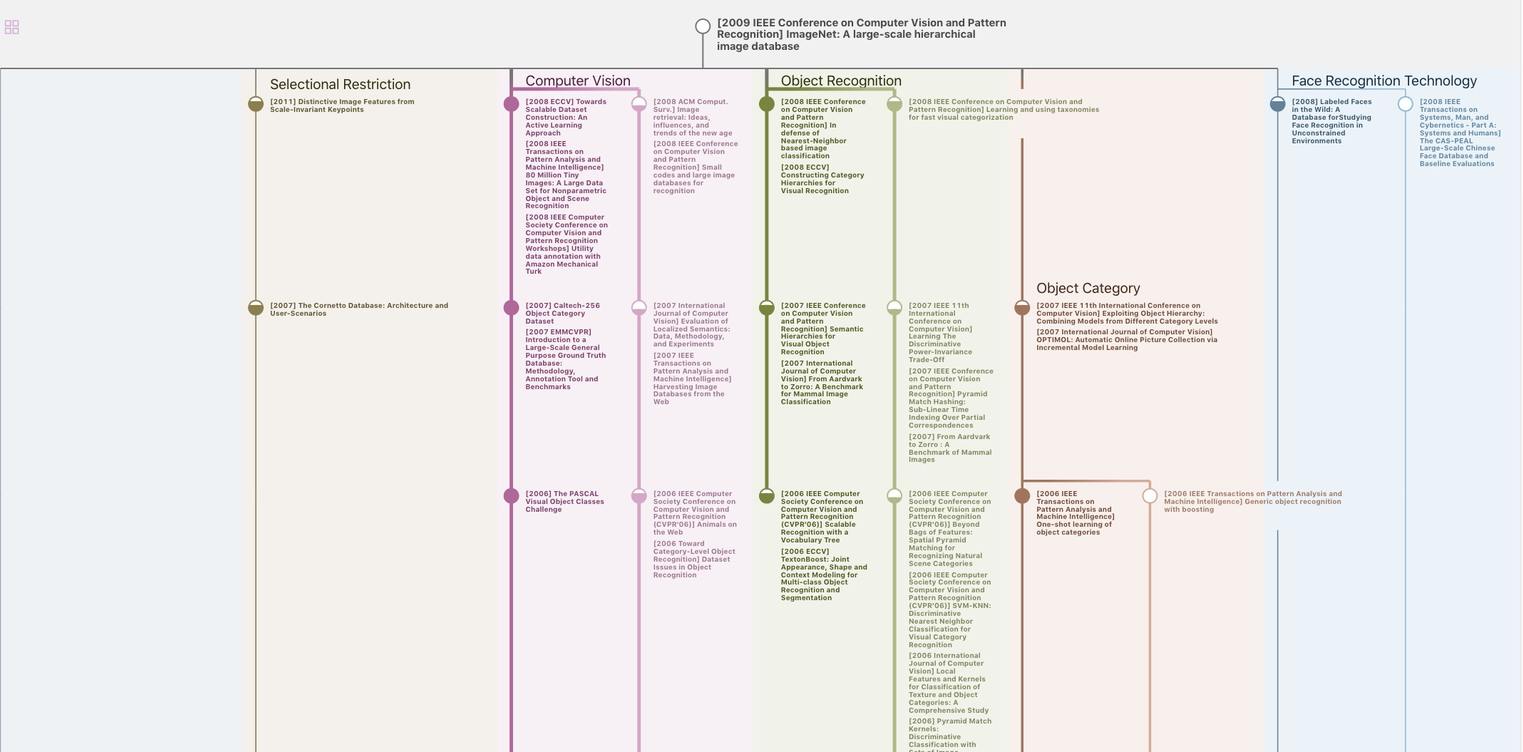
Generate MRT to find the research sequence of this paper
Chat Paper
Summary is being generated by the instructions you defined