Randomized Gradient Descents on Riemannian Manifolds: Almost Sure Convergence to Global Minima in and beyond Quantum Optimization
arxiv(2024)
摘要
We analyze the convergence properties of gradient descent algorithms on
Riemannian manifolds. We study randomization of the tangent space directions of
Riemannian gradient flows for minimizing smooth cost functions (of Morse–Bott
type) to obtain convergence to local optima. We prove that through randomly
projecting Riemannian gradients according to the Haar measure, convergence to
local optima can be obtained almost surely despite the existence of saddle
points. As an application we consider ground state preparation through quantum
optimization over the unitary group. In this setting one can efficiently
approximate the Haar-random projections by implementing unitary 2-designs on
quantum computers. We prove that the respective algorithm almost surely
converges to the global minimum that corresponds to the ground state of a
desired Hamiltonian. Finally, we discuss the time required by the algorithm to
pass a saddle point in a simple two-dimensional setting.
更多查看译文
AI 理解论文
溯源树
样例
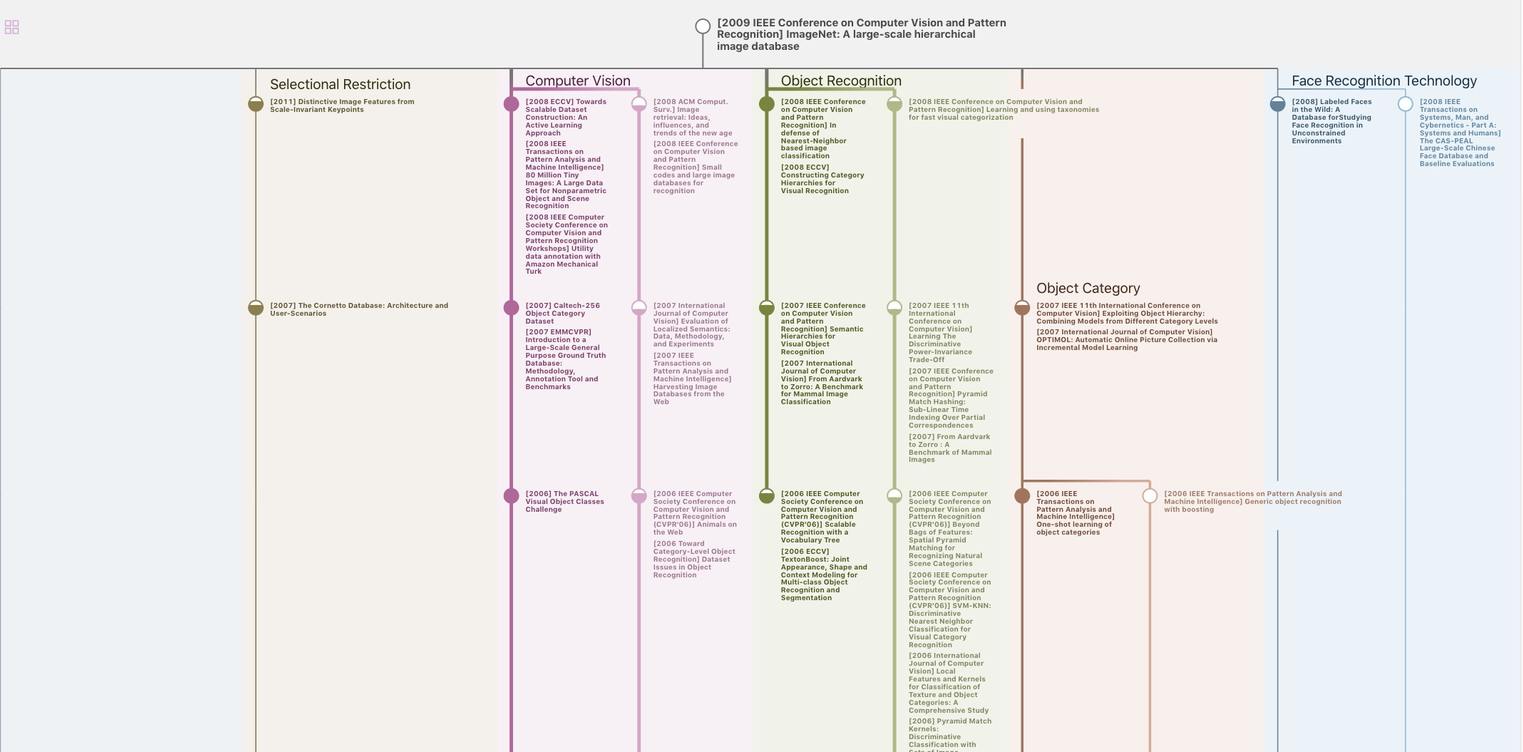
生成溯源树,研究论文发展脉络
Chat Paper
正在生成论文摘要