Dynamic classifier auditing by unsupervised anomaly detection methods: an application in packaging industry predictive maintenance
CoRR(2024)
摘要
Predictive maintenance in manufacturing industry applications is a
challenging research field. Packaging machines are widely used in a large
number of logistic companies' warehouses and must be working uninterruptedly.
Traditionally, preventive maintenance strategies have been carried out to
improve the performance of these machines. However, this kind of policies does
not take into account the information provided by the sensors implemented in
the machines. This paper presents an expert system for the automatic estimation
of work orders to implement predictive maintenance policies for packaging
machines. The key idea is that, from a set of alarms related to sensors
implemented in the machine, the expert system should take a maintenance action
while optimizing the response time. The work order estimator will act as a
classifier, yielding a binary decision of whether a machine must undergo a
maintenance action by a technician or not, followed by an unsupervised anomaly
detection-based filtering stage to audit the classifier's output. The methods
used for anomaly detection were: One-Class Support Vector Machine (OCSVM),
Minimum Covariance Determinant (MCD) and a majority (hard) voting ensemble of
them. All anomaly detection methods improve the performance of the baseline
classifer but the best performance in terms of F1 score was obtained by the
majority voting ensemble.
更多查看译文
AI 理解论文
溯源树
样例
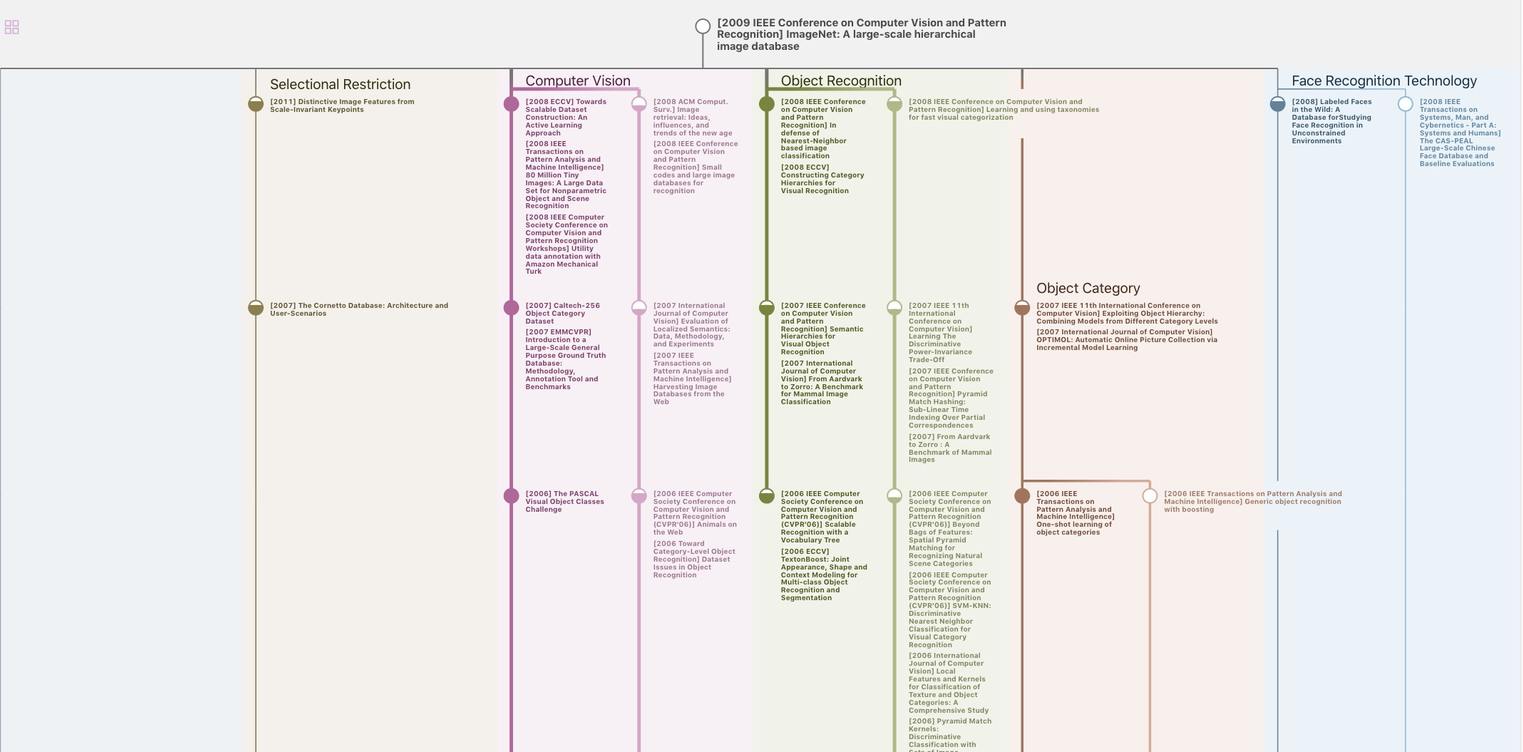
生成溯源树,研究论文发展脉络
Chat Paper
正在生成论文摘要