Beyond MLE: Investigating SEARNN for Low-Resourced Neural Machine Translation
CoRR(2024)
Abstract
Structured prediction tasks, like machine translation, involve learning
functions that map structured inputs to structured outputs. Recurrent Neural
Networks (RNNs) have historically been a popular choice for such tasks,
including in natural language processing (NLP) applications. However, training
RNNs using Maximum Likelihood Estimation (MLE) has its limitations, including
exposure bias and a mismatch between training and testing metrics. SEARNN,
based on the learning to search (L2S) framework, has been proposed as an
alternative to MLE for RNN training. This project explored the potential of
SEARNN to improve machine translation for low-resourced African languages – a
challenging task characterized by limited training data availability and the
morphological complexity of the languages. Through experiments conducted on
translation for English to Igbo, French to , and French to directions, this project evaluated the efficacy of SEARNN over MLE in
addressing the unique challenges posed by these languages. With an average BLEU
score improvement of 5.4% over the MLE objective, we proved that SEARNN is
indeed a viable algorithm to effectively train RNNs on machine translation for
low-resourced languages.
MoreTranslated text
AI Read Science
Must-Reading Tree
Example
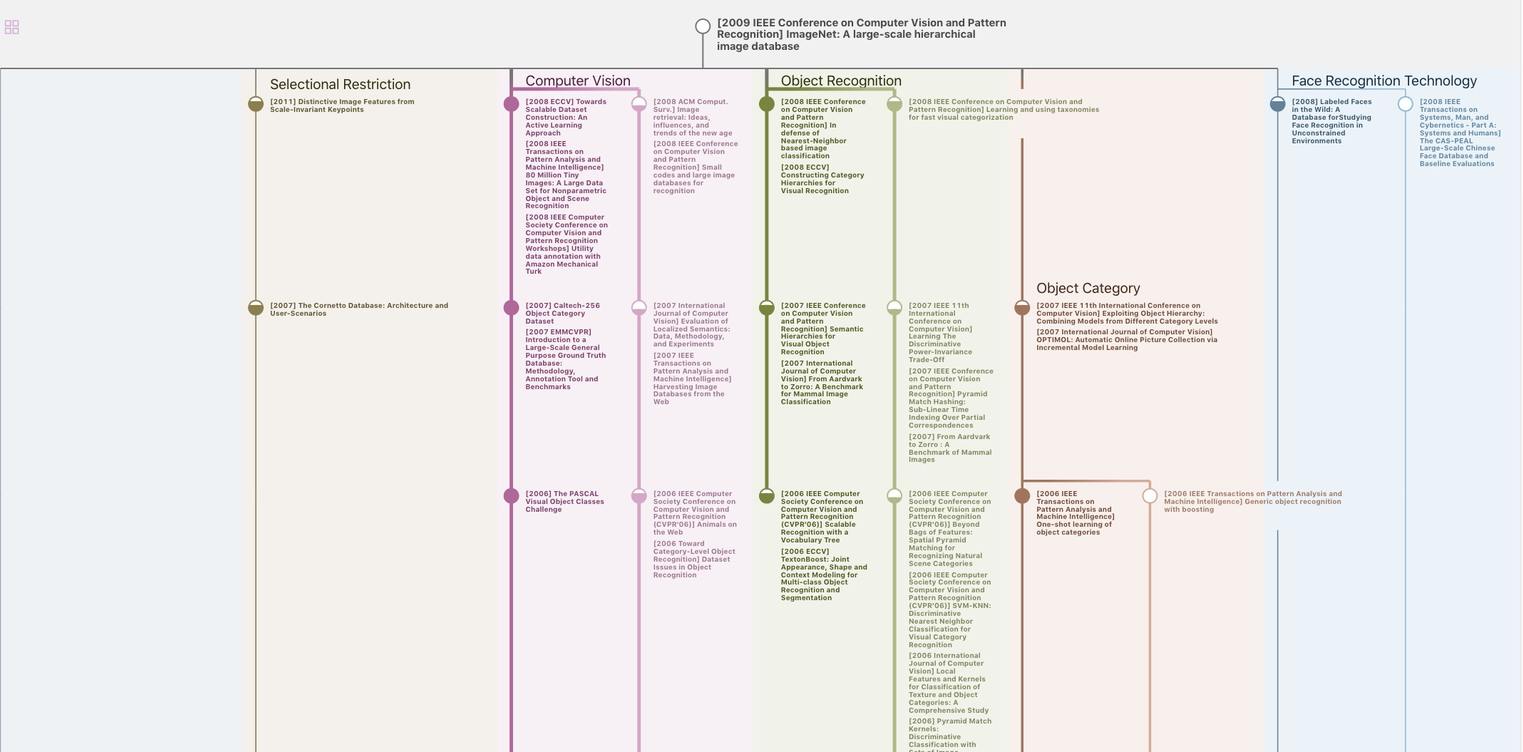
Generate MRT to find the research sequence of this paper
Chat Paper
Summary is being generated by the instructions you defined