"What do you want from theory alone?" Experimenting with Tight Auditing of Differentially Private Synthetic Data Generation
CoRR(2024)
摘要
Differentially private synthetic data generation (DP-SDG) algorithms are used
to release datasets that are structurally and statistically similar to
sensitive data while providing formal bounds on the information they leak.
However, bugs in algorithms and implementations may cause the actual
information leakage to be higher. This prompts the need to verify whether the
theoretical guarantees of state-of-the-art DP-SDG implementations also hold in
practice. We do so via a rigorous auditing process: we compute the information
leakage via an adversary playing a distinguishing game and running membership
inference attacks (MIAs). If the leakage observed empirically is higher than
the theoretical bounds, we identify a DP violation; if it is non-negligibly
lower, the audit is loose.
We audit six DP-SDG implementations using different datasets and threat
models and find that black-box MIAs commonly used against DP-SDGs are severely
limited in power, yielding remarkably loose empirical privacy estimates. We
then consider MIAs in stronger threat models, i.e., passive and active
white-box, using both existing and newly proposed attacks. Overall, we find
that, currently, we do not only need white-box MIAs but also worst-case
datasets to tightly estimate the privacy leakage from DP-SDGs. Finally, we show
that our automated auditing procedure finds both known DP violations (in 4 out
of the 6 implementations) as well as a new one in the DPWGAN implementation
that was successfully submitted to the NIST DP Synthetic Data Challenge.
The source code needed to reproduce our experiments is available from
https://github.com/spalabucr/synth-audit.
更多查看译文
AI 理解论文
溯源树
样例
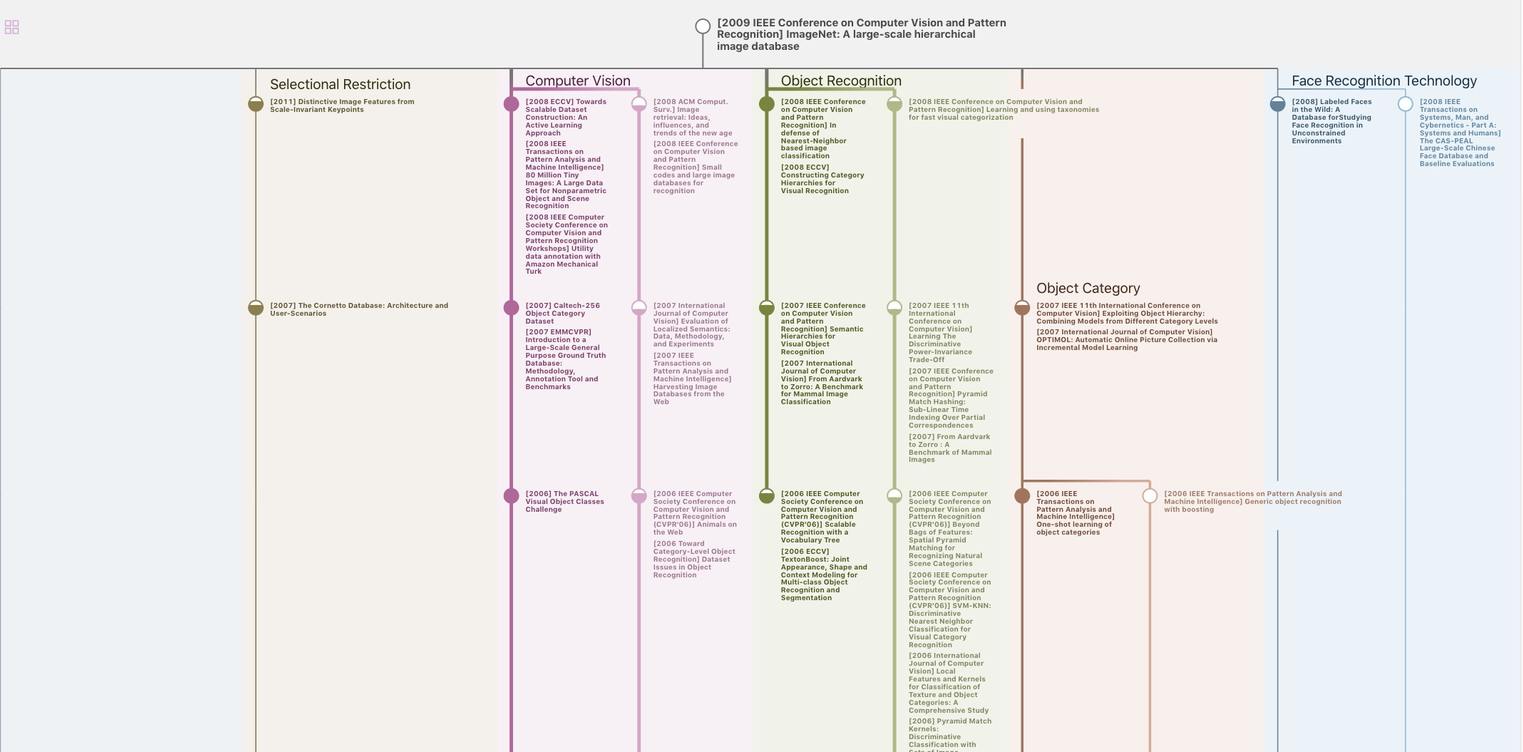
生成溯源树,研究论文发展脉络
Chat Paper
正在生成论文摘要