A One-Step Methodology for Identifying Concrete Pathologies Using Neural Networks—Using YOLO v8 and Dataset Review
Applied Sciences(2024)
摘要
Pathologies in concrete structures can be visually evidenced on the concrete surface, such as by fissures or cracks, fragmentation of part of the concrete, concrete efflorescence, corrosion stains on the concrete surface, or exposed steel bars, the latter two occurring in reinforced concrete. Therefore, these pathologies can be analyzed via the images of concrete structures. This article proposes a methodology for visually inspecting concrete structures using deep neural networks. This method makes it possible to speed up the detection task and increase its effectiveness by saving time in preparing the identifications to be analyzed and eliminating or reducing errors, such as those resulting from human errors caused by the execution of tedious, repetitive analysis tasks. The methodology was tested to analyze its accuracy. The neural network architecture used for detection was YOLO, versions 4 and 8, which was tested to analyze the gain with migration to a more recent version. The dataset for classification was Ozgnel, which was trained with YOLO version 8, and the detection dataset was CODEBRIM. The use of a dedicated classification dataset allows for a better-trained network for this function and results in the elimination of false positives in the detection stage. The classification achieved 99.65% accuracy.
更多查看译文
AI 理解论文
溯源树
样例
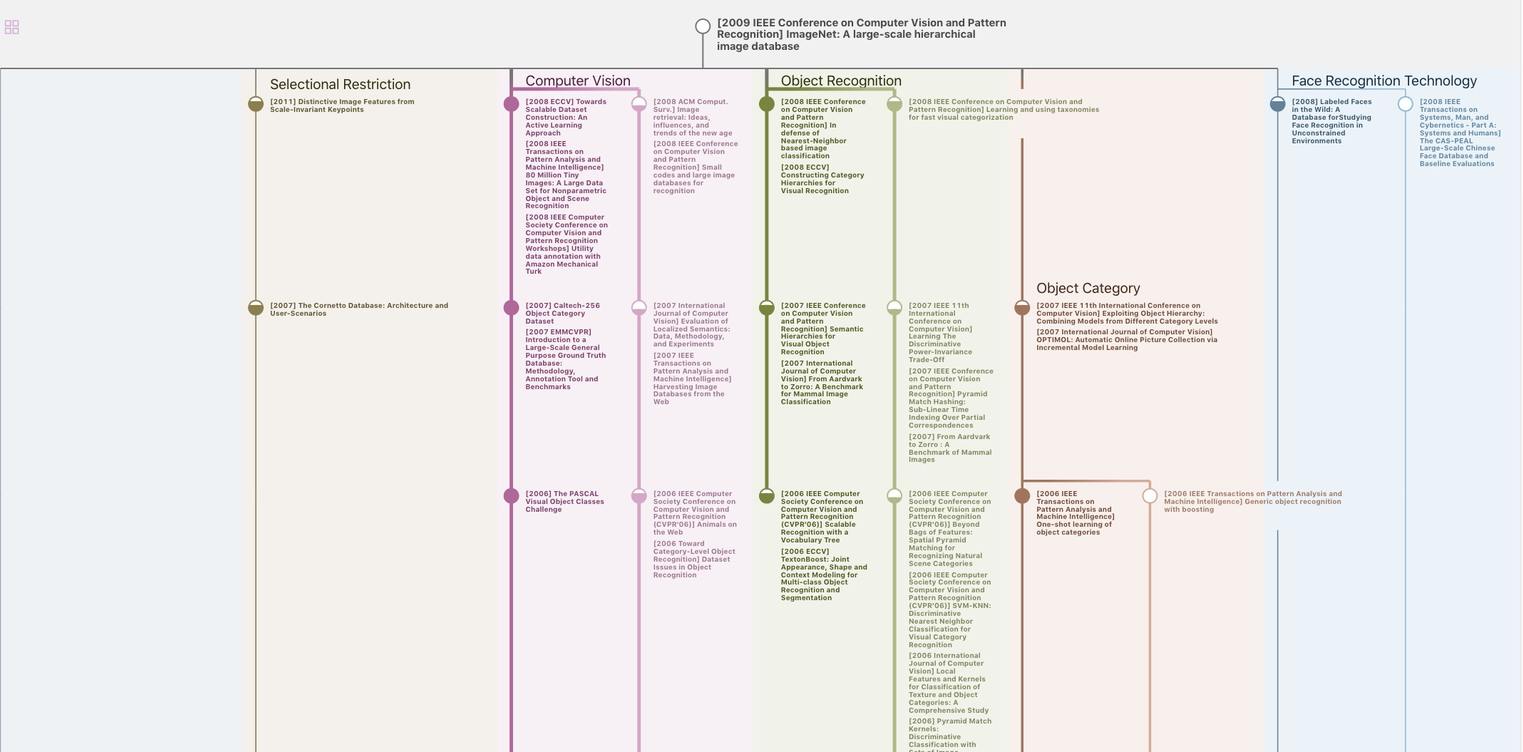
生成溯源树,研究论文发展脉络
Chat Paper
正在生成论文摘要