ro-PINN: A reduced order physics-informed neural network for solving the macroscopic model of pedestrian flows
Transportation Research Part C: Emerging Technologies(2024)
摘要
Given the importance of pedestrian flow simulation in reproducing pedestrian flows and optimizing pedestrian facilities, it is crucial to accurately simulate macroscopic pedestrian flows. Physics-informed neural network (PINN) is a recently proposed advanced scheme for solving partial differential equations (PDE) that can approximate the solution values by minimizing the initial values, boundary values, and residuals of the PDE. However, few studies have discussed how the performance of PINN changes in the face of macroscopic pedestrian flow equations with high-order derivatives and multiple variables. In this study, we propose to compare the performance of three schemes, i.e., the vanilla PINN, the extended-variable PINN (ev-PINN), and the reduced-order PINN (ro-PINN), by solving the macroscopic pedestrian flow equations coupled by the conservation equation and Eikonal equation in the steady-state and transient cases. The results show that the comparison of the PINN models under different hyperparameters indicates that the ro-PINN is the most stable in training, while the other two schemes have a certain degree of fluctuation in the face of different hyperparameters. Secondly, when the traditional numerical solution scheme is used as the reference solution, the ro-PINN solution works best in the steady-state and transient cases, and the solution results of different solution variables such as density and flow are closest to the reference solution, which can make the whole solution process easier and the solution results more accurate by reducing the derivative order in the automatic differentiation part and increasing the output dimension of the neural network. On the contrary, compared with vanilla PINN, ev-PINN not only did not improve the solution results, but even reduced the performance. Finally, we compare the running time of the three PINN schemes, and although increasing the output dimension and decreasing the order of PDE increase the computing time, it is not significant. Therefore, the ro-PINN can be used as an effective alternative model for simulating macroscopic pedestrian flow evolution.
更多查看译文
关键词
Traffic,Pedestrian flow model,Neural network,Numerical solution,PINN
AI 理解论文
溯源树
样例
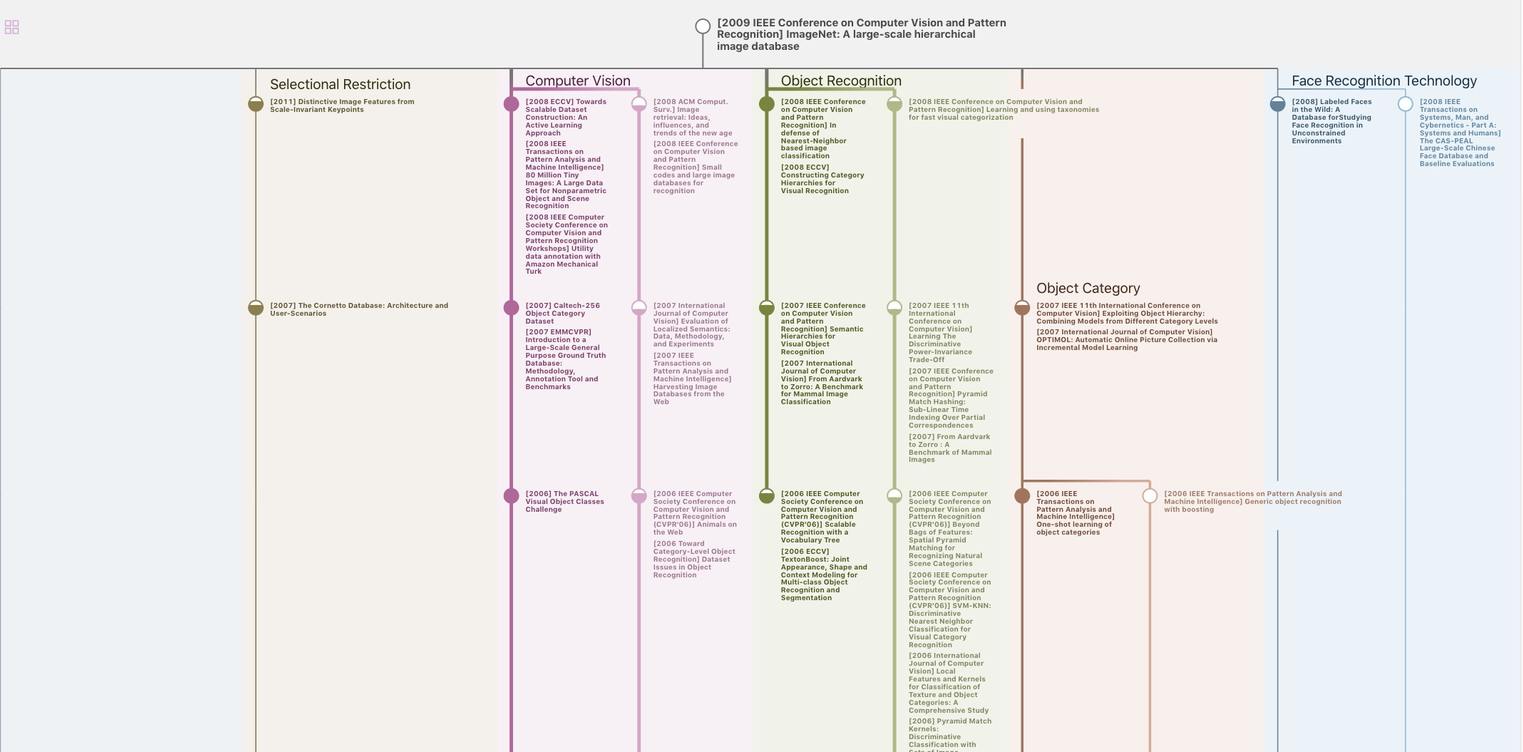
生成溯源树,研究论文发展脉络
Chat Paper
正在生成论文摘要