Kernel Expansions for High-Dimensional Mean-Field Control with Non-local Interactions
arxiv(2024)
摘要
Mean-field control (MFC) problems aim to find the optimal policy to control
massive populations of interacting agents. These problems are crucial in areas
such as economics, physics, and biology. We consider the non-local setting,
where the interactions between agents are governed by a suitable kernel. For
N agents, the interaction cost has 𝒪(N^2) complexity, which can
be prohibitively slow to evaluate and differentiate when N is large. To this
end, we propose an efficient primal-dual algorithm that utilizes basis
expansions of the kernels. The basis expansions reduce the cost of computing
the interactions, while the primal-dual methodology decouples the agents at the
expense of solving for a moderate number of dual variables. We also demonstrate
that our approach can further be structured in a multi-resolution manner, where
we estimate optimal dual variables using a moderate N and solve decoupled
trajectory optimization problems for large N. We illustrate the effectiveness
of our method on an optimal control of 5000 interacting quadrotors.
更多查看译文
AI 理解论文
溯源树
样例
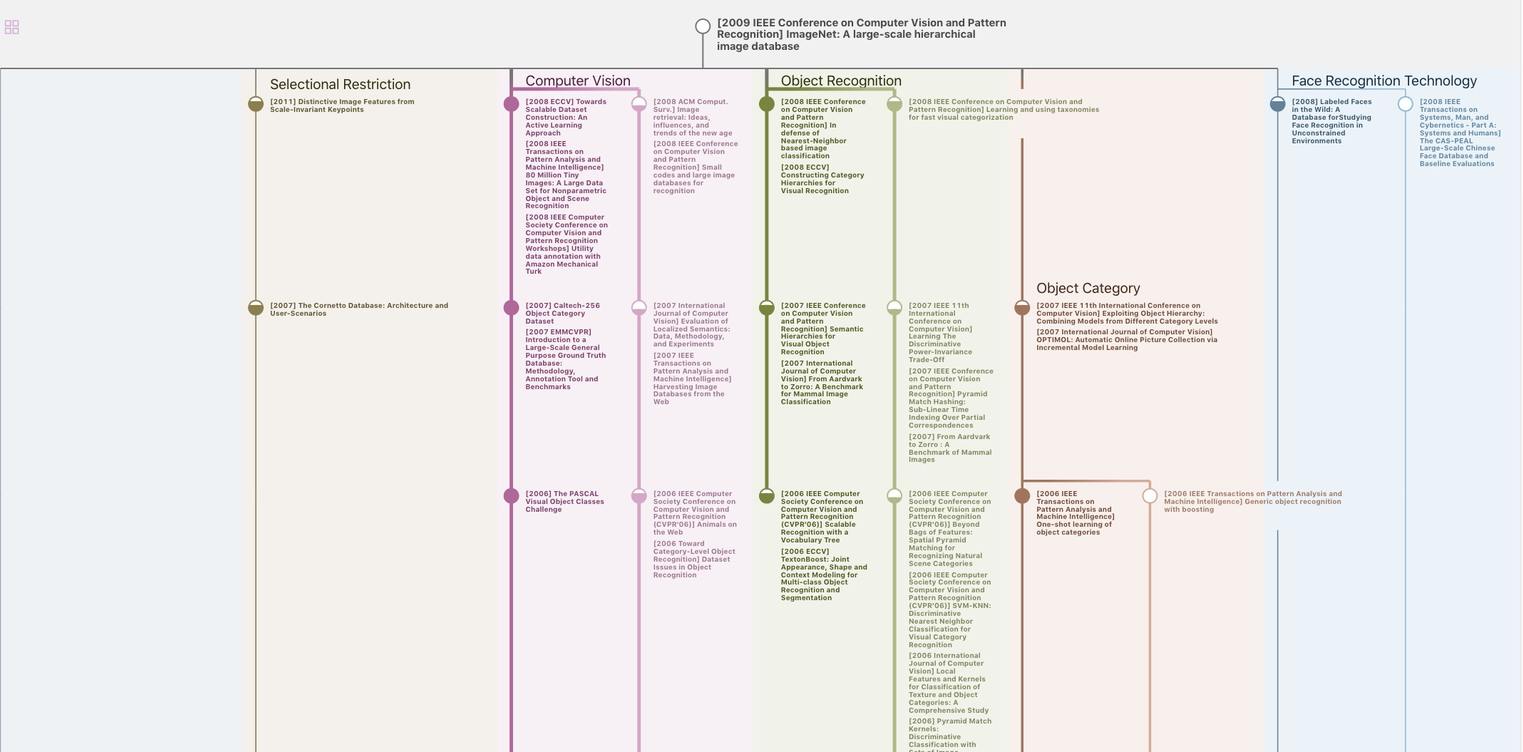
生成溯源树,研究论文发展脉络
Chat Paper
正在生成论文摘要