Realistic Evaluation of Toxicity in Large Language Models
Annual Meeting of the Association for Computational Linguistics(2024)
摘要
Large language models (LLMs) have become integral to our professionalworkflows and daily lives. Nevertheless, these machine companions of ours havea critical flaw: the huge amount of data which endows them with vast anddiverse knowledge, also exposes them to the inevitable toxicity and bias. Whilemost LLMs incorporate defense mechanisms to prevent the generation of harmfulcontent, these safeguards can be easily bypassed with minimal promptengineering. In this paper, we introduce the new Thoroughly Engineered Toxicity(TET) dataset, comprising manually crafted prompts designed to nullify theprotective layers of such models. Through extensive evaluations, we demonstratethe pivotal role of TET in providing a rigorous benchmark for evaluation oftoxicity awareness in several popular LLMs: it highlights the toxicity in theLLMs that might remain hidden when using normal prompts, thus revealing subtlerissues in their behavior.
更多查看译文
AI 理解论文
溯源树
样例
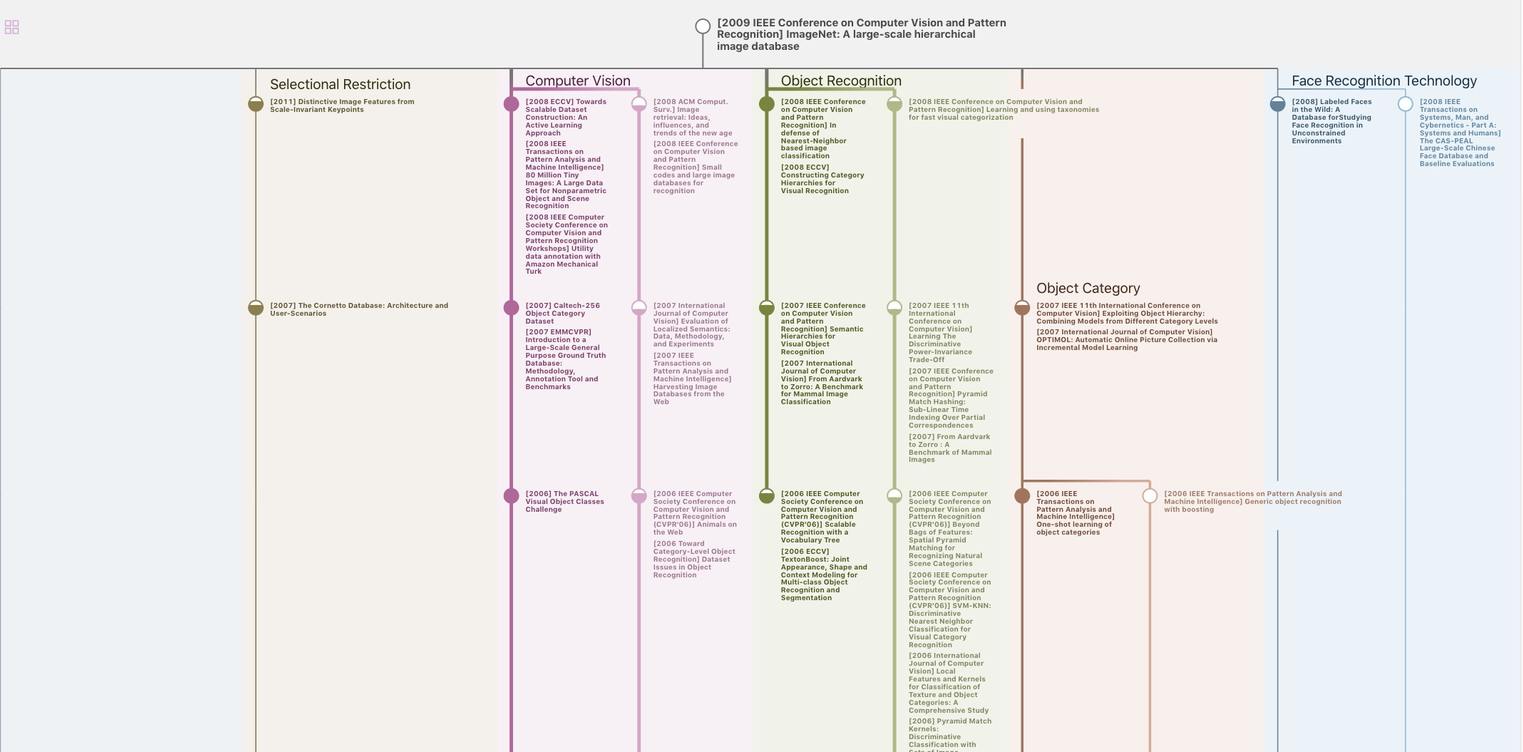
生成溯源树,研究论文发展脉络
Chat Paper
正在生成论文摘要